29 Platanus hispanica
29.1 Données d’occurrences GBIF
Collecte des données du GBIF :
<<gbif download metadata>>
Status: SUCCEEDED
DOI: 10.15468/dl.tr8zzp
Format: SIMPLE_CSV
Download key: 0232026-230224095556074
Created: 2023-05-11T06:36:46.402+00:00
Modified: 2023-05-11T06:42:16.400+00:00
Download link: https://api.gbif.org/v1/occurrence/download/request/0232026-230224095556074.zip
Total records: 264214
Conversion en objets géographiques (format Simple feature
de sf
) et
exploration rapide des données (classe du jeu de données et premières lignes du
tableau) :
Simple feature collection with 7216 features and 50 fields
Attribute-geometry relationship: 50 constant, 0 aggregate, 0 identity
Geometry type: POINT
Dimension: XY
Bounding box: xmin: -123.169868 ymin: -44.417713 xmax: 175.055841 ymax: 59.34687
Geodetic CRS: WGS 84
# A tibble: 7,216 × 51
gbifID datasetKey occurrenceID kingdom phylum class order family genus species infraspecificEpithet
* <int64> <chr> <chr> <chr> <chr> <chr> <chr> <chr> <chr> <chr> <chr>
1 3.e9 5f06e39d-… USFS - New … Plantae Trach… Magn… Prot… Plata… Plat… Platan… ""
2 3.e9 5f06e39d-… USFS - New … Plantae Trach… Magn… Prot… Plata… Plat… Platan… ""
3 3.e9 d1e9202b-… NYCstreetTr… Plantae Trach… Magn… Prot… Plata… Plat… Platan… ""
4 3.e9 c4e1739b-… BISON:New Y… Plantae Trach… Magn… Prot… Plata… Plat… Platan… ""
5 3.e9 d1e9202b-… NYCstreetTr… Plantae Trach… Magn… Prot… Plata… Plat… Platan… ""
6 3.e9 c4e1739b-… BISON:New Y… Plantae Trach… Magn… Prot… Plata… Plat… Platan… ""
7 3.e9 c4e1739b-… BISON:New Y… Plantae Trach… Magn… Prot… Plata… Plat… Platan… ""
8 3.e9 d1e9202b-… NYCstreetTr… Plantae Trach… Magn… Prot… Plata… Plat… Platan… ""
9 3.e9 5f06e39d-… USFS - New … Plantae Trach… Magn… Prot… Plata… Plat… Platan… ""
10 3.e9 c4e1739b-… BISON:New Y… Plantae Trach… Magn… Prot… Plata… Plat… Platan… ""
# ℹ 7,206 more rows
# ℹ 40 more variables: taxonRank <chr>, scientificName <chr>, verbatimScientificName <chr>,
# verbatimScientificNameAuthorship <chr>, countryCode <chr>, locality <chr>, stateProvince <chr>,
# occurrenceStatus <chr>, individualCount <int>, publishingOrgKey <chr>, decimalLatitude <dbl>,
# decimalLongitude <dbl>, coordinateUncertaintyInMeters <dbl>, coordinatePrecision <dbl>,
# elevation <dbl>, elevationAccuracy <dbl>, depth <dbl>, depthAccuracy <dbl>, eventDate <dttm>,
# day <int>, month <int>, year <int>, taxonKey <int>, speciesKey <int>, basisOfRecord <chr>, …
Il y a 7 216 occurrences dans le jeu de données. On les affiche sur la carte du monde :
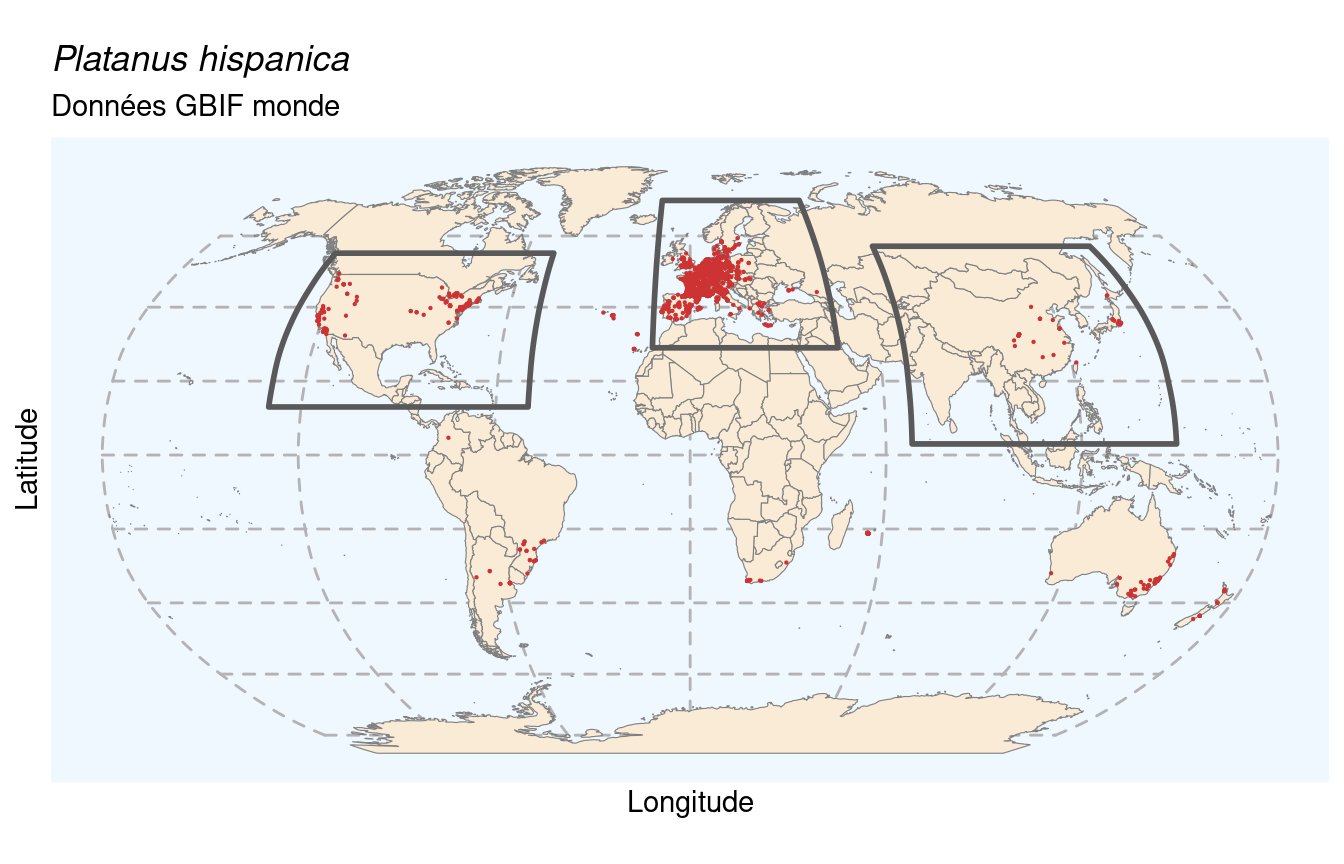
Figure 29.1: Occurrences de Platanus hispanica dans le monde.
29.1.1 Données par région
On évalue la proportion d’occurrences dans chaque région, en utilisant les 3 masques créés précédemment. Si on a au moins 50 % des occurrences dans une région, on peut continuer en travaillant uniquement sur ces données. Sinon, on vérifie qu’au moins 67 % des données tombent dans l’ensemble des régions, et on prend la région la plus représentée. Au final, s’il s’agit d’une espèce endémique d’Europe, on n’a pas besoin de manipulation supplémentaire.
[1] 0.9131097561
[1] 0.05349223947
[1] 0.004988913525
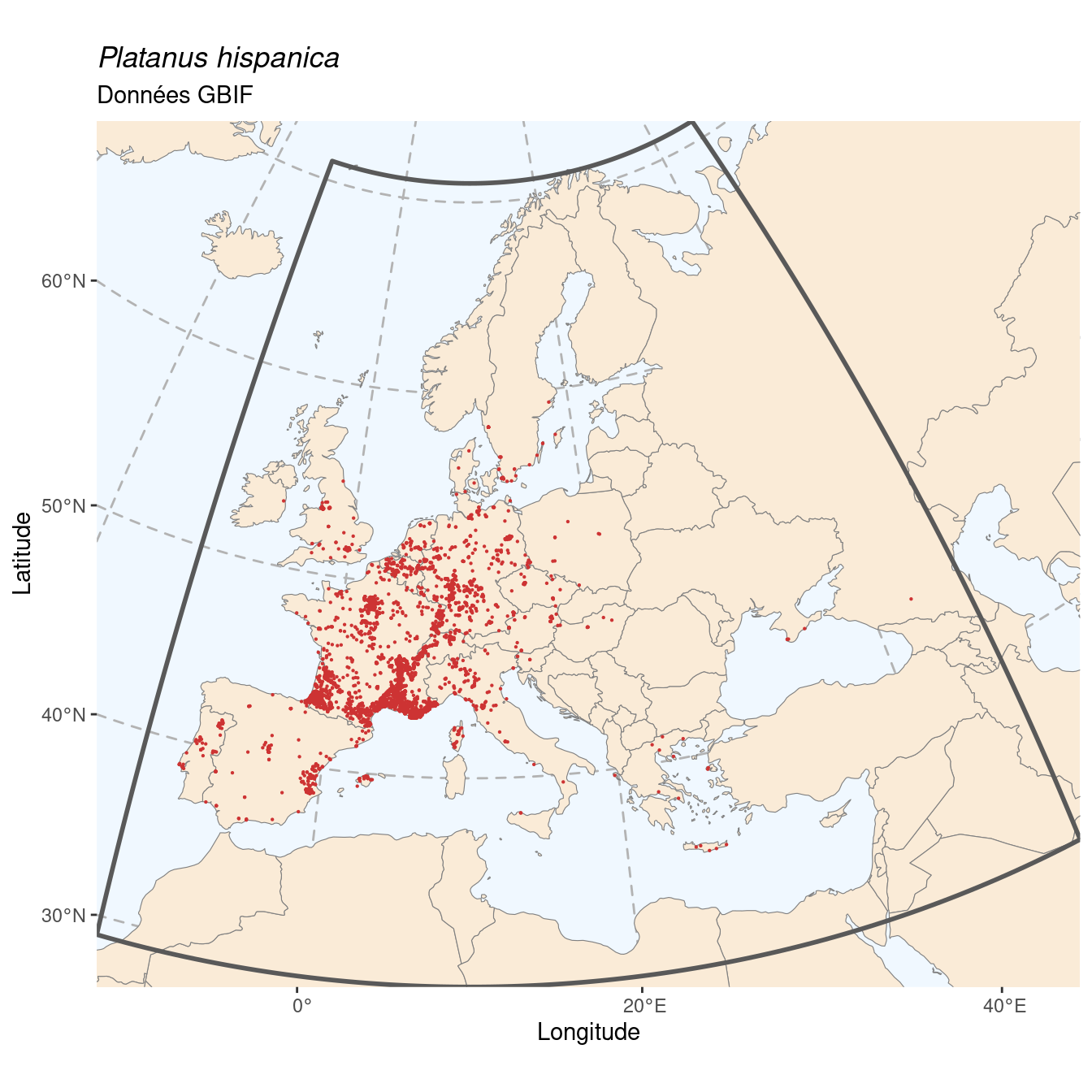
Figure 29.2: Occurrence de Platanus hispanica dans la région d’endémisme.
29.1.2 Licences
On s’intéresse ici au problème particulier des licences :
CC_BY_4_0 CC_BY_NC_4_0 CC0_1_0
5302 1056 231
[1] 83.97328881
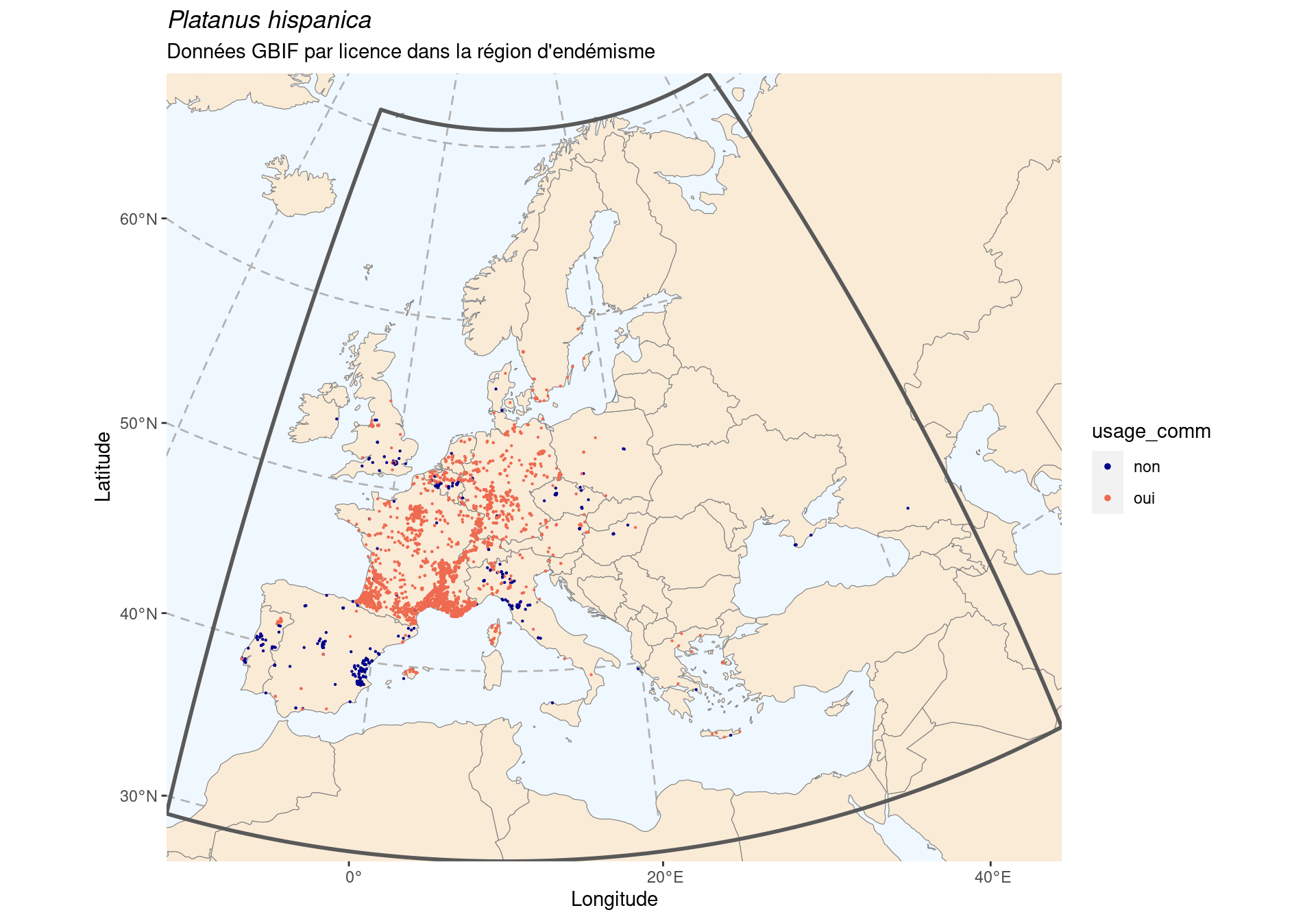
Figure 29.3: Occurrence de Platanus hispanica dans la région d’endémisme, selon la licence : CC BY-NC en bleu ; CC0 et CC BY en orange.
Idéalement, on aimerait avoir au moins 50 % de données vraiment libres
d’utilisation (c’est-à-dire sans la clause -NC
). On continue en supprimant les
données en CC BY-NC.
[1] 5533
29.1.3 Sous-échantillonnage
Si le jeu de données contient 25 000 occurrences ou plus, on procède au sous-échantillonnage aléatoire pour en conserver un maximum de 25 000.
Simple feature collection with 5533 features and 50 fields
Attribute-geometry relationship: 50 constant, 0 aggregate, 0 identity
Geometry type: POINT
Dimension: XY
Bounding box: xmin: -9.429703 ymin: 34.9729 xmax: 26.244364 ymax: 59.34687
Geodetic CRS: WGS 84
# A tibble: 5,533 × 51
gbifID datasetKey occurrenceID kingdom phylum class order family genus species infraspecificEpithet
<int64> <chr> <chr> <chr> <chr> <chr> <chr> <chr> <chr> <chr> <chr>
1 1e9 75956ee6-… http://cbnm… Plantae Trach… Magn… Prot… Plata… Plat… Platan… ""
2 1e9 75956ee6-… http://pifh… Plantae Trach… Magn… Prot… Plata… Plat… Platan… ""
3 1e9 75956ee6-… http://flor… Plantae Trach… Magn… Prot… Plata… Plat… Platan… ""
4 1e9 75956ee6-… http://flor… Plantae Trach… Magn… Prot… Plata… Plat… Platan… ""
5 1e9 75956ee6-… http://pifh… Plantae Trach… Magn… Prot… Plata… Plat… Platan… ""
6 1e9 75956ee6-… http://flor… Plantae Trach… Magn… Prot… Plata… Plat… Platan… ""
7 1e9 75956ee6-… http://pifh… Plantae Trach… Magn… Prot… Plata… Plat… Platan… ""
8 1e9 75956ee6-… http://ofsa… Plantae Trach… Magn… Prot… Plata… Plat… Platan… ""
9 1e9 75956ee6-… http://flor… Plantae Trach… Magn… Prot… Plata… Plat… Platan… ""
10 1e9 75956ee6-… http://flor… Plantae Trach… Magn… Prot… Plata… Plat… Platan… ""
# ℹ 5,523 more rows
# ℹ 40 more variables: taxonRank <chr>, scientificName <chr>, verbatimScientificName <chr>,
# verbatimScientificNameAuthorship <chr>, countryCode <chr>, locality <chr>, stateProvince <chr>,
# occurrenceStatus <chr>, individualCount <int>, publishingOrgKey <chr>, decimalLatitude <dbl>,
# decimalLongitude <dbl>, coordinateUncertaintyInMeters <dbl>, coordinatePrecision <dbl>,
# elevation <dbl>, elevationAccuracy <dbl>, depth <dbl>, depthAccuracy <dbl>, eventDate <dttm>,
# day <int>, month <int>, year <int>, taxonKey <int>, speciesKey <int>, basisOfRecord <chr>, …
29.2 Modélisation de la niche climatique
29.2.1 Préparer les données
Organisation des données d’entrées (occurrences et données environnementales) et des données produites de pseudo-absences :
class : SpatVector
geometry : points
dimensions : 5533, 0 (geometries, attributes)
extent : -9.429703, 26.24436, 34.9729, 59.34687 (xmin, xmax, ymin, ymax)
coord. ref. : lon/lat WGS 84 (EPSG:4326)
-=-=-=-=-=-=-=-=-=-=-=-=-=-=-= plhi Data Formating -=-=-=-=-=-=-=-=-=-=-=-=-=-=-=
! Response variable is considered as only presences... Is it really what you want?
! No data has been set aside for modeling evaluation
!!! Some data are located in the same raster cell.
Please set `filter.raster = TRUE` if you want an automatic filtering.
Checking Pseudo-absence selection arguments...
> random pseudo absences selection
> Pseudo absences are selected in explanatory variables
! Some NAs have been automatically removed from your data
!!! Some data are located in the same raster cell.
Please set `filter.raster = TRUE` if you want an automatic filtering.
-=-=-=-=-=-=-=-=-=-=-=-=-=-=-=-=-=-= Done -=-=-=-=-=-=-=-=-=-=-=-=-=-=-=-=-=-=
-=-=-=-=-=-=-=-=-=-=-=-=-=-= BIOMOD.formated.data -=-=-=-=-=-=-=-=-=-=-=-=-=-=
dir.name = Output
sp.name = plhi
5519 presences, 0 true absences and 16505 undefined points in dataset
6 explanatory variables
temp_max_august temp_min temp_wet_quart temp_season
Min. : 0.50 Min. :-21.768 Min. :-11.467 Min. : 0.0
1st Qu.:20.93 1st Qu.: -8.697 1st Qu.: 9.764 1st Qu.: 615.6
Median :24.95 Median : -1.776 Median : 13.176 Median : 716.5
Mean :25.51 Mean : -3.592 Mean : 12.663 Mean : 752.1
3rd Qu.:28.69 3rd Qu.: 1.696 3rd Qu.: 16.320 3rd Qu.: 894.9
Max. :44.88 Max. : 10.589 Max. : 24.703 Max. :1373.9
prec_wet_quart prec_season
Min. : 5.0 Min. : 7.483
1st Qu.: 176.0 1st Qu.: 23.066
Median : 220.0 Median : 31.850
Mean : 223.2 Mean : 35.867
3rd Qu.: 264.0 3rd Qu.: 40.589
Max. :1222.0 Max. :123.444
-=-=-=-=-=-=-=-=-=-=-=-=-=-=-=-=-=-=-=-=-=-=-=-=-=-=-=-=-=-=-=-=-=-=-=-=-=-=-=-=
On visualise les pseudo-absences :
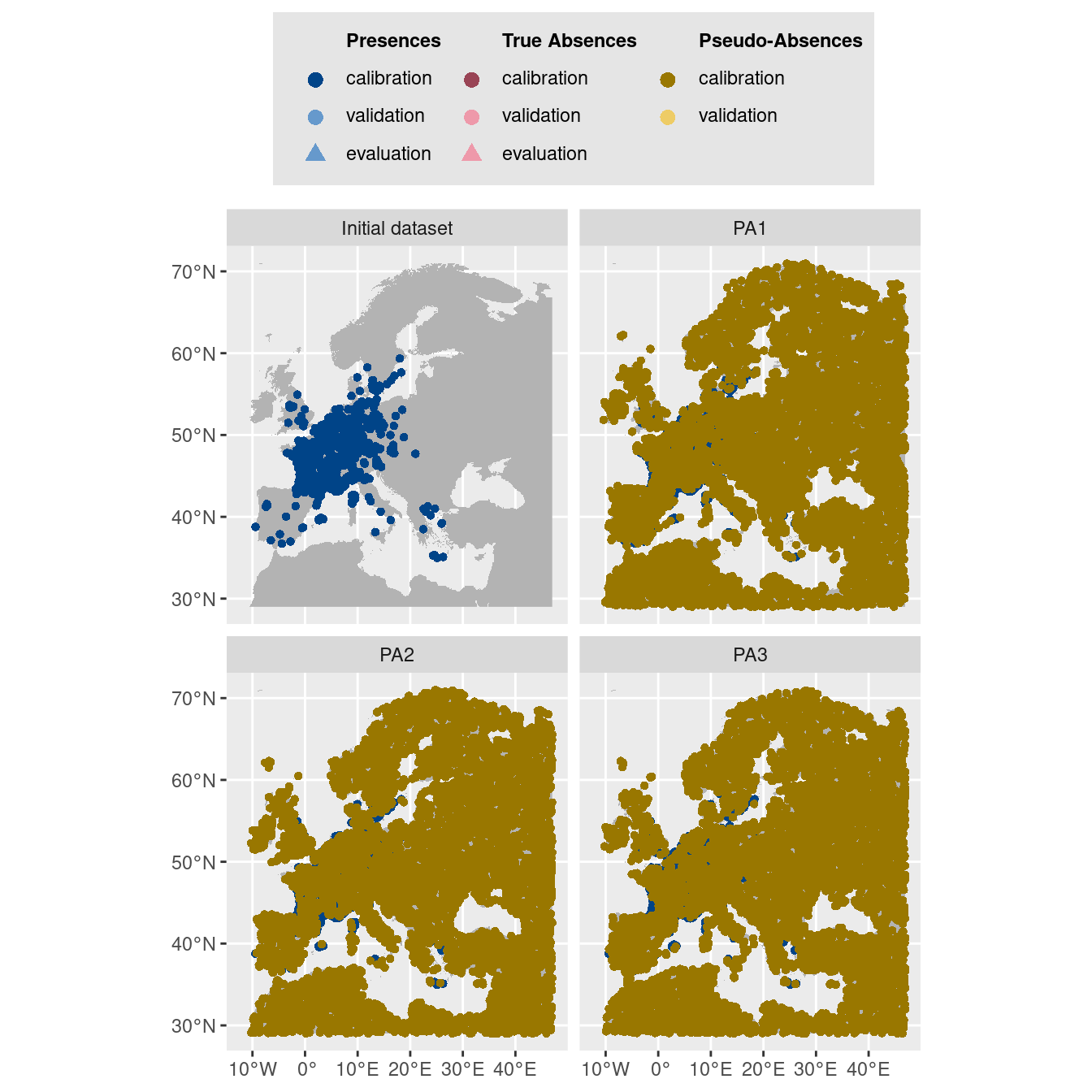
Figure 29.5: Les données d’occurrences (en haut à gauche) et les trois jeux de données aléatoires de pseudo-absences.
$data.vect
class : SpatVector
geometry : points
dimensions : 38675, 2 (geometries, attributes)
extent : -10.39583, 46.97917, 29.02083, 70.97917 (xmin, xmax, ymin, ymax)
coord. ref. :
names : resp dataset
type : <num> <chr>
values : 10 Initial dataset
10 Initial dataset
10 Initial dataset
$data.label
9 10
"**Presences**" "Presences (calibration)"
11 12
"Presences (validation)" "Presences (evaluation)"
19 20
"**True Absences**" "True Absences (calibration)"
21 22
"True Absences (validation)" "True Absences (evaluation)"
29 30
"**Pseudo-Absences**" "Pseudo-Absences (calibration)"
31 1
"Pseudo-Absences (validation)" "Background"
$data.plot
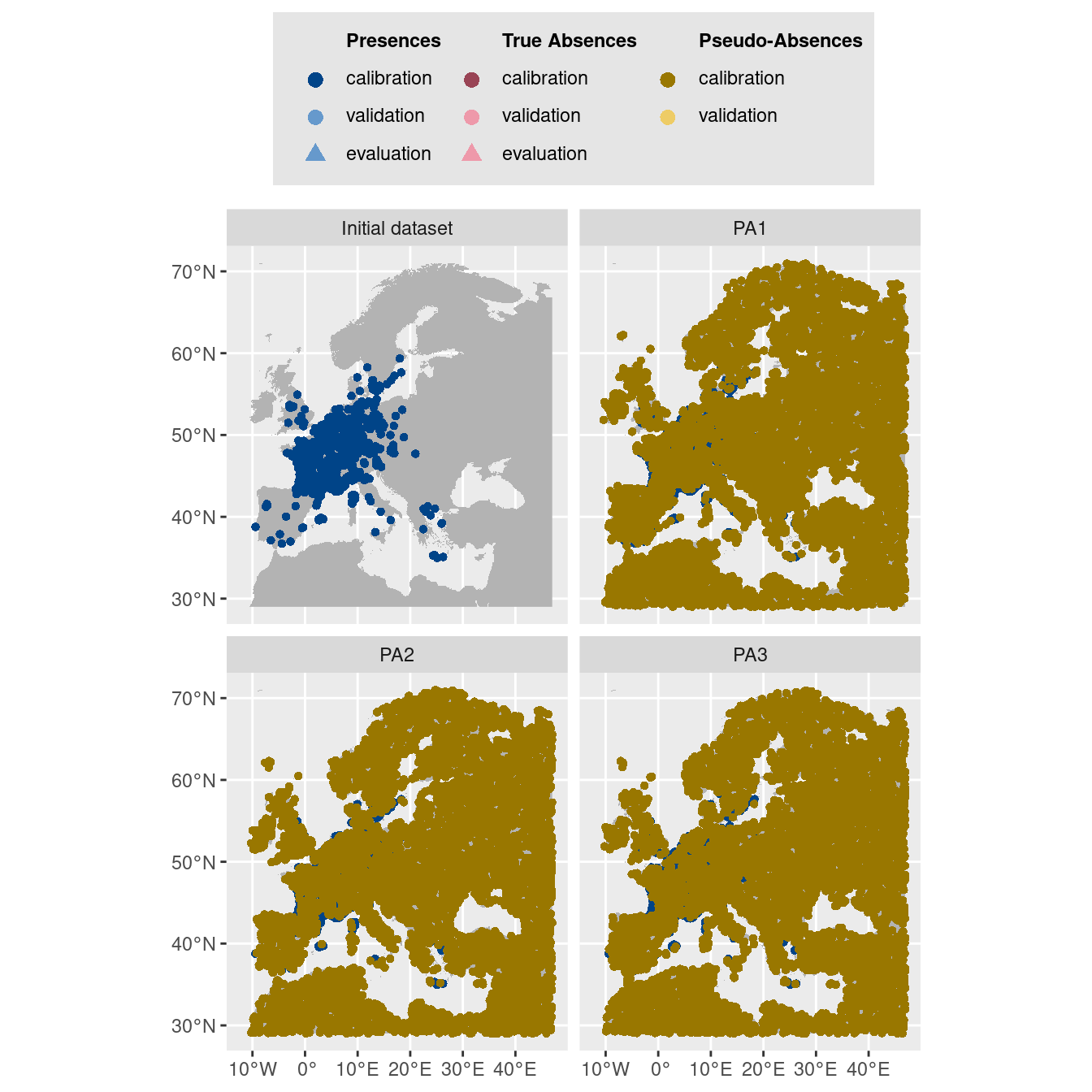
Figure 29.6: Les données d’occurrences (en haut à gauche) et les trois jeux de données aléatoires de pseudo-absences.
29.2.2 Modèles individuels de niche
-=-=-=-=-=-=-=-=-=-=-=-=-=-=-= Build Single Models -=-=-=-=-=-=-=-=-=-=-=-=-=-=-=
Checking Models arguments...
Creating suitable Workdir...
! Weights where automatically defined for plhi_PA1 to rise a 0.5 prevalence !
! Weights where automatically defined for plhi_PA2 to rise a 0.5 prevalence !
! Weights where automatically defined for plhi_PA3 to rise a 0.5 prevalence !
-=-=-=-=-=-=-=-=-=-=-=-=-=-= plhi Modeling Summary -=-=-=-=-=-=-=-=-=-=-=-=-=-=
6 environmental variables ( temp_max_august temp_min temp_wet_quart temp_season prec_wet_quart prec_season )
Number of evaluation repetitions : 1
Models selected : GAM MARS MAXNET GBM ANN RF
Total number of model runs: 18
-=-=-=-=-=-=-=-=-=-=-=-=-=-=-=-=-=-=-=-=-=-=-=-=-=-=-=-=-=-=-=-=-=-=-=-=-=-=-=-=
-=-=-=- Run : plhi_PA1
-=-=-=--=-=-=- plhi_PA1_RUN1
-=-=-=- Run : plhi_PA2
-=-=-=--=-=-=- plhi_PA2_RUN1
-=-=-=- Run : plhi_PA3
-=-=-=--=-=-=- plhi_PA3_RUN1
-=-=-=-=-=-=-=-=-=-=-=-=-=-=-=-=-=-= Done -=-=-=-=-=-=-=-=-=-=-=-=-=-=-=-=-=-=
29.2.2.1 Évaluation de la performance
full.name PA run algo metric.eval cutoff sensitivity
1 plhi_PA1_RUN1_GAM PA1 RUN1 GAM TSS 444.0 98.075
2 plhi_PA1_RUN1_GAM PA1 RUN1 GAM ROC 509.5 97.441
3 plhi_PA1_RUN1_MARS PA1 RUN1 MARS TSS 463.0 96.557
4 plhi_PA1_RUN1_MARS PA1 RUN1 MARS ROC 454.5 96.716
5 plhi_PA1_RUN1_MAXNET PA1 RUN1 MAXNET TSS 348.0 96.285
6 plhi_PA1_RUN1_MAXNET PA1 RUN1 MAXNET ROC 358.5 95.810
specificity calibration validation evaluation
1 60.235 0.583 0.591 NA
2 60.935 0.731 0.730 NA
3 87.912 0.845 0.842 NA
4 87.822 0.964 0.960 NA
5 88.771 0.851 0.839 NA
6 89.404 0.966 0.963 NA
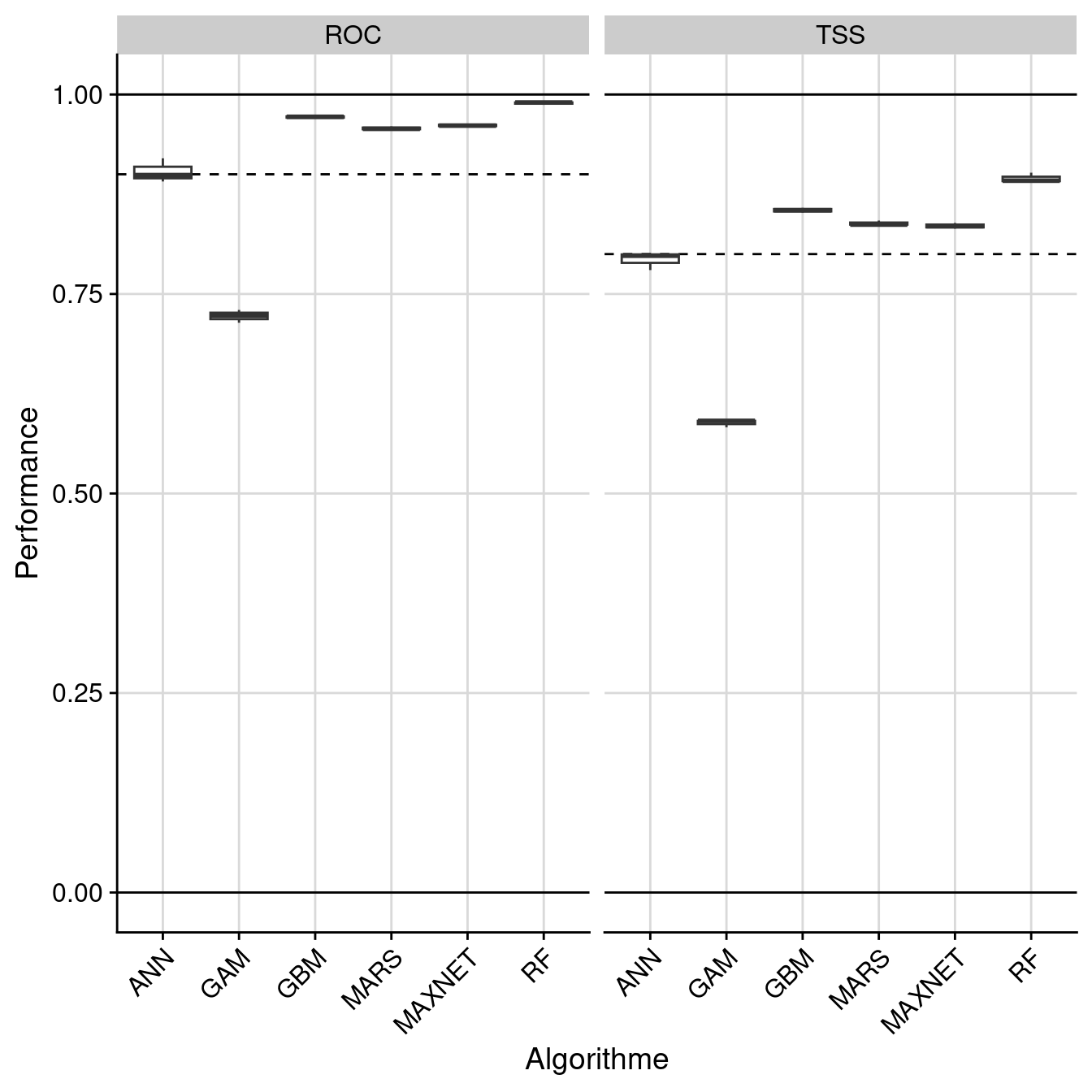
Figure 29.7: Évaluation de la performance des modèles individuels : True Skill Statistic & aire sous la courbe de ROC.
29.2.2.2 Importance des variables environnementales
full.name PA run algo expl.var rand var.imp
1 plhi_PA1_RUN1_GAM PA1 RUN1 GAM temp_max_august 1 0.103510
2 plhi_PA1_RUN1_GAM PA1 RUN1 GAM temp_min 1 0.380937
3 plhi_PA1_RUN1_GAM PA1 RUN1 GAM temp_wet_quart 1 0.015113
4 plhi_PA1_RUN1_GAM PA1 RUN1 GAM temp_season 1 0.247897
5 plhi_PA1_RUN1_GAM PA1 RUN1 GAM prec_wet_quart 1 0.011113
6 plhi_PA1_RUN1_GAM PA1 RUN1 GAM prec_season 1 0.052348
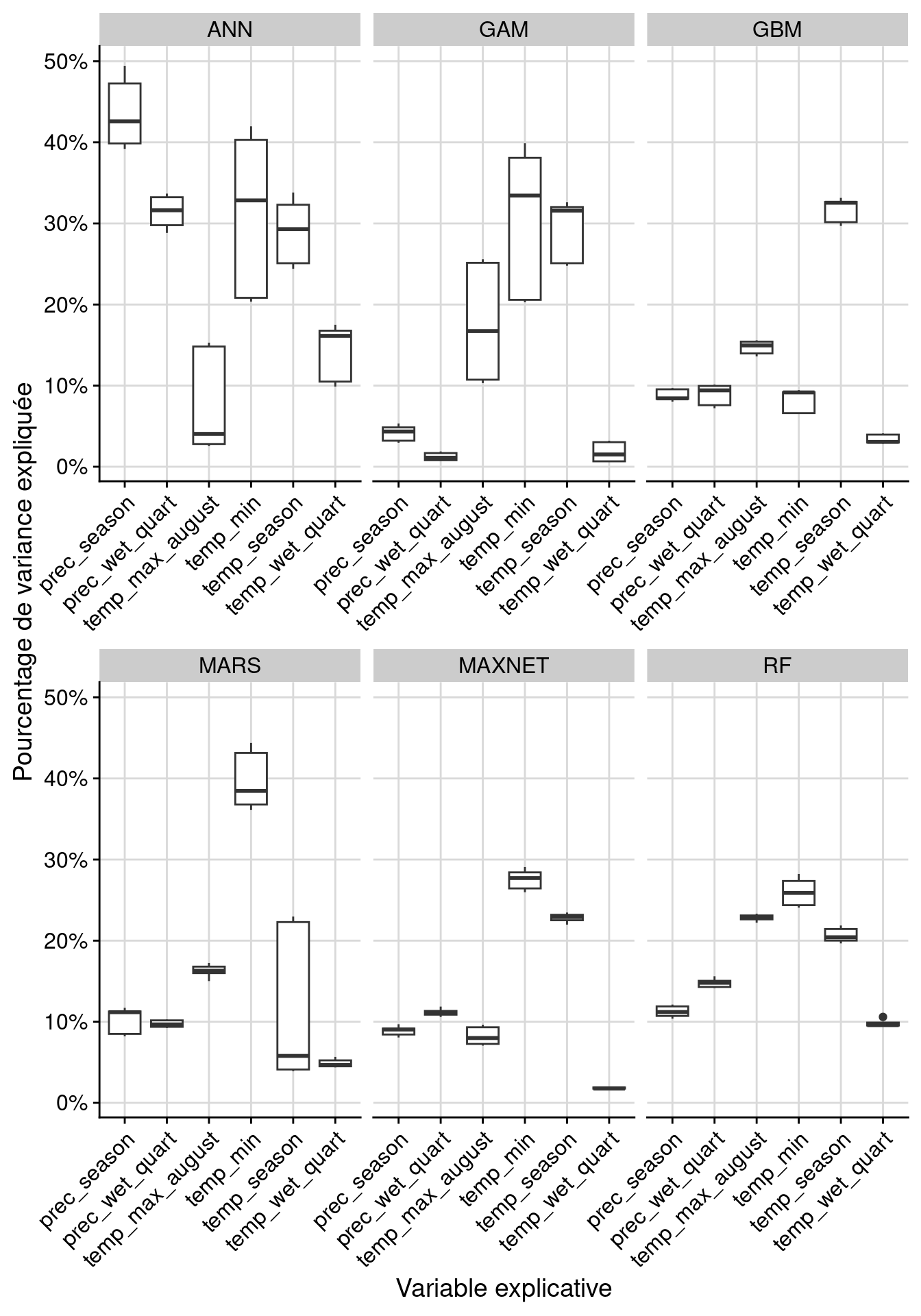
Figure 29.8: Pourcentage de variance expliquée pour chaque variable, décomposé par algorithme.
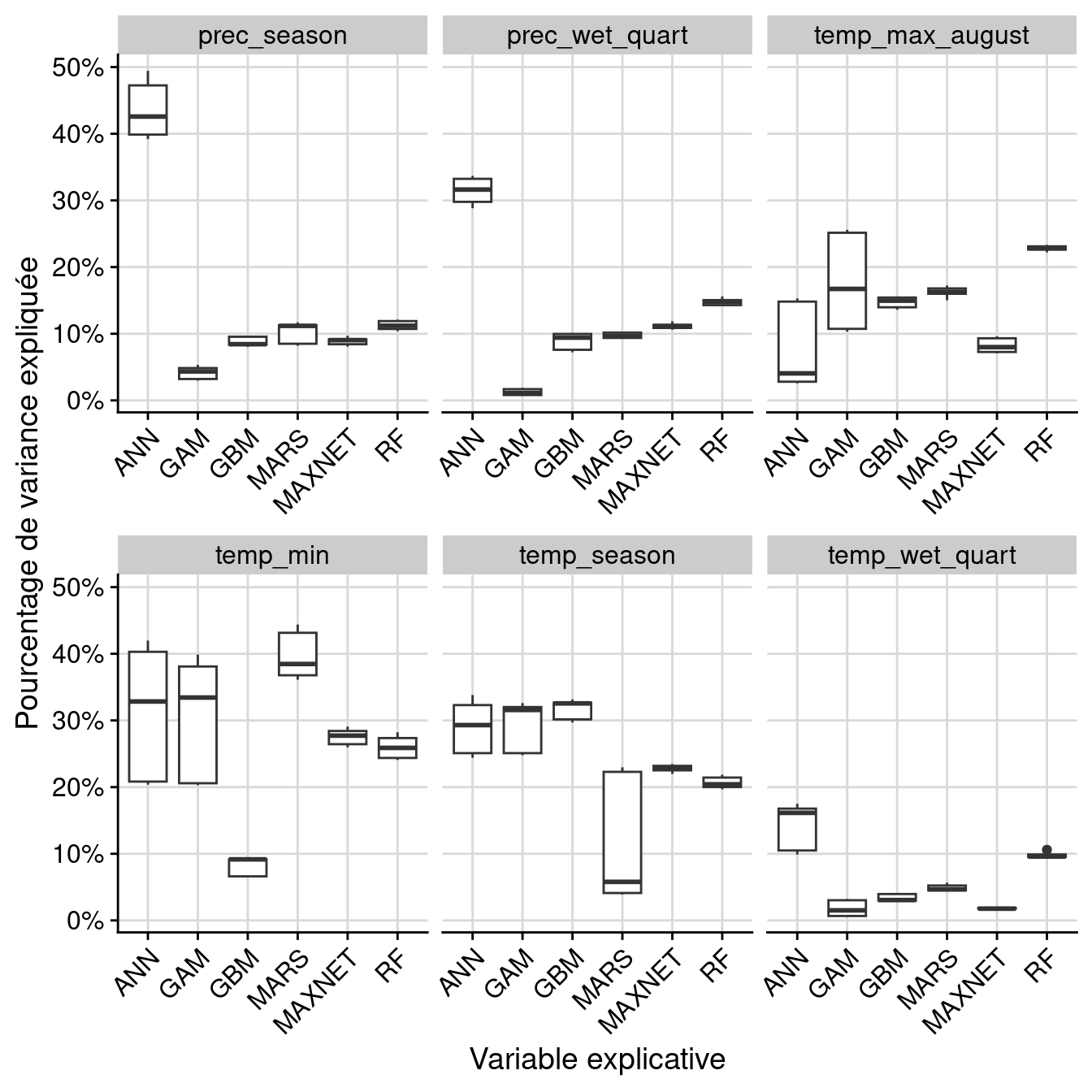
Figure 29.9: Pourcentage de variance expliquée pour chaque variable, décomposé par variable.
29.2.3 Modèles d’ensemble de niche
Seulement modèles avec TSS > 0.8
-=-=-=-=-=-=-=-=-=-=-=-=-= BIOMOD.ensemble.models.out -=-=-=-=-=-=-=-=-=-=-=-=-=
sp.name : plhi
expl.var.names : temp_max_august temp_min temp_wet_quart temp_season
prec_wet_quart prec_season
models computed:
plhi_EMcvByTSS_mergedData_mergedRun_mergedAlgo, plhi_EMwmeanByTSS_mergedData_mergedRun_mergedAlgo
models failed: none
-=-=-=-=-=-=-=-=-=-=-=-=-=-=-=-=-=-=-=-=-=-=-=-=-=-=-=-=-=-=-=-=-=-=-=-=-=-=-=-=
29.2.3.1 Évaluation de la qualité
full.name merged.by.PA merged.by.run
1 plhi_EMwmeanByTSS_mergedData_mergedRun_mergedAlgo mergedData mergedRun
2 plhi_EMwmeanByTSS_mergedData_mergedRun_mergedAlgo mergedData mergedRun
merged.by.algo filtered.by algo metric.eval cutoff sensitivity specificity
1 mergedAlgo TSS EMwmean TSS 604.0 94.709 94.311
2 mergedAlgo TSS EMwmean ROC 645.5 93.858 95.304
calibration validation evaluation
1 0.891 NA NA
2 0.990 NA NA
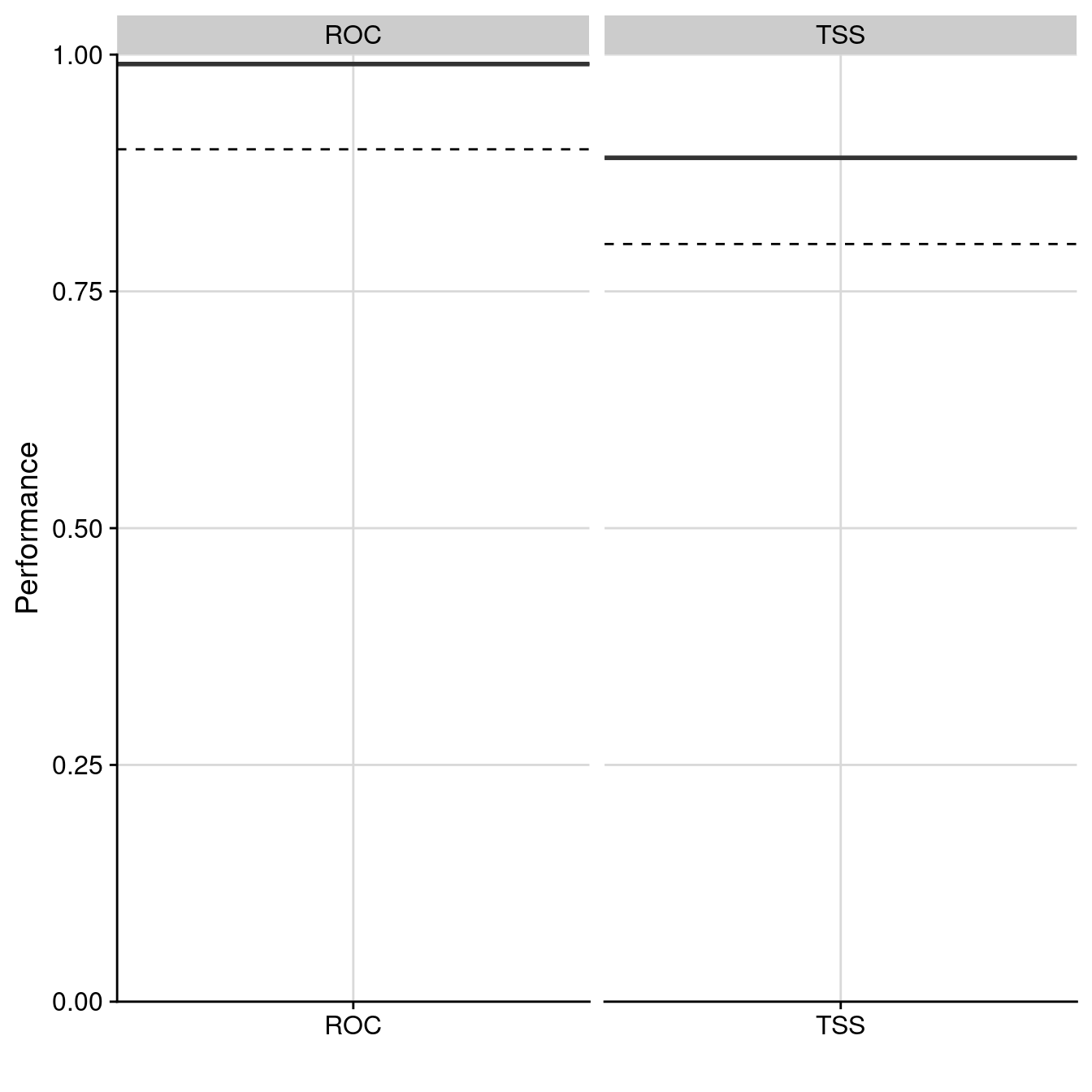
Figure 29.11: Évaluation de la qualité du modèle d’ensemble : Aire sous la courbe de ROC & True Skill Statistic.
full.name merged.by.PA merged.by.run
1 plhi_EMcvByTSS_mergedData_mergedRun_mergedAlgo mergedData mergedRun
2 plhi_EMcvByTSS_mergedData_mergedRun_mergedAlgo mergedData mergedRun
3 plhi_EMcvByTSS_mergedData_mergedRun_mergedAlgo mergedData mergedRun
4 plhi_EMcvByTSS_mergedData_mergedRun_mergedAlgo mergedData mergedRun
5 plhi_EMcvByTSS_mergedData_mergedRun_mergedAlgo mergedData mergedRun
6 plhi_EMcvByTSS_mergedData_mergedRun_mergedAlgo mergedData mergedRun
merged.by.algo filtered.by algo expl.var rand var.imp
1 mergedAlgo TSS EMcv temp_max_august 1 0.184667
2 mergedAlgo TSS EMcv temp_min 1 0.345774
3 mergedAlgo TSS EMcv temp_wet_quart 1 0.050990
4 mergedAlgo TSS EMcv temp_season 1 0.248789
5 mergedAlgo TSS EMcv prec_wet_quart 1 0.183716
6 mergedAlgo TSS EMcv prec_season 1 0.103680
Par variable :
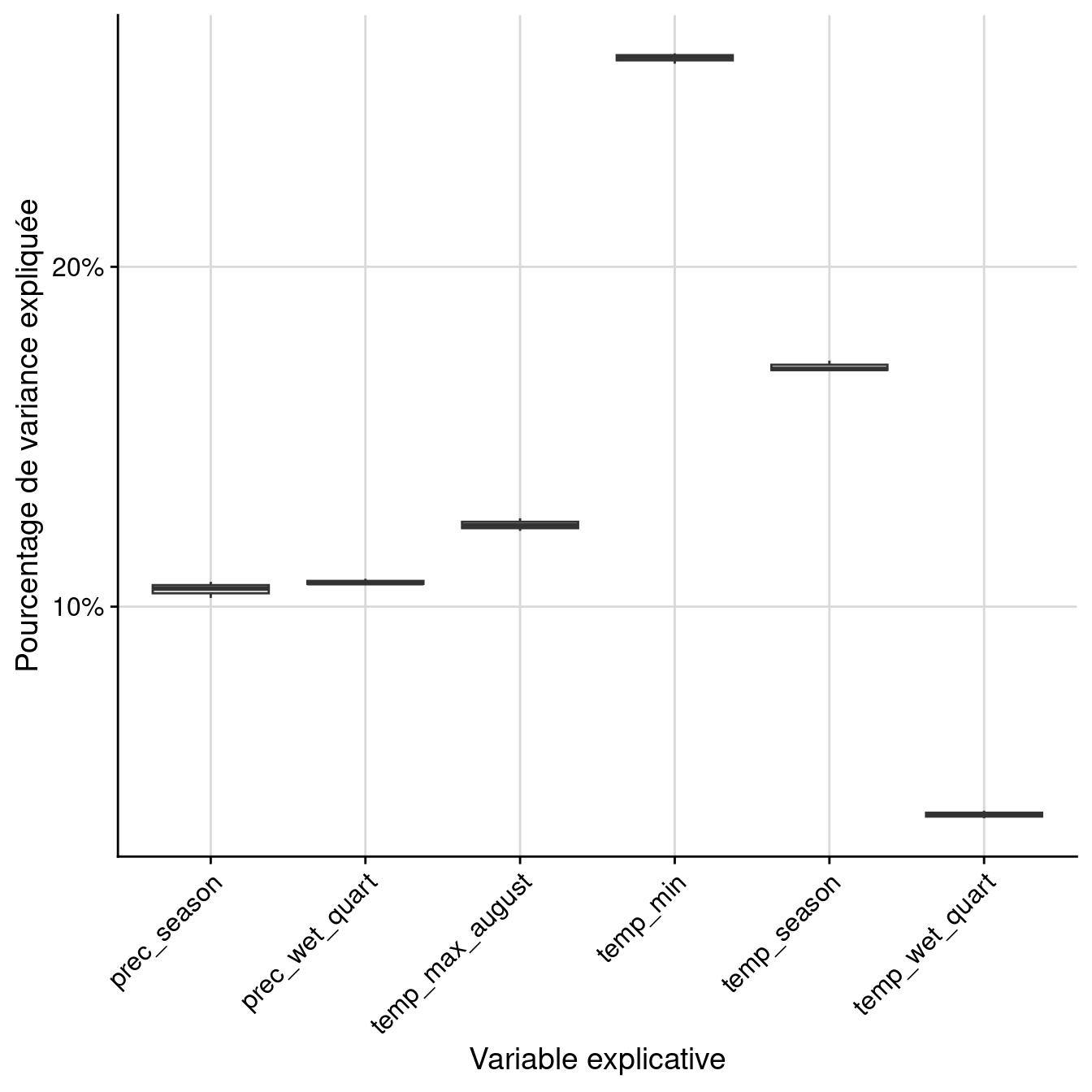
Figure 29.12: Pourcentage de variance expliquée par variable dans le modèle d’ensemble.
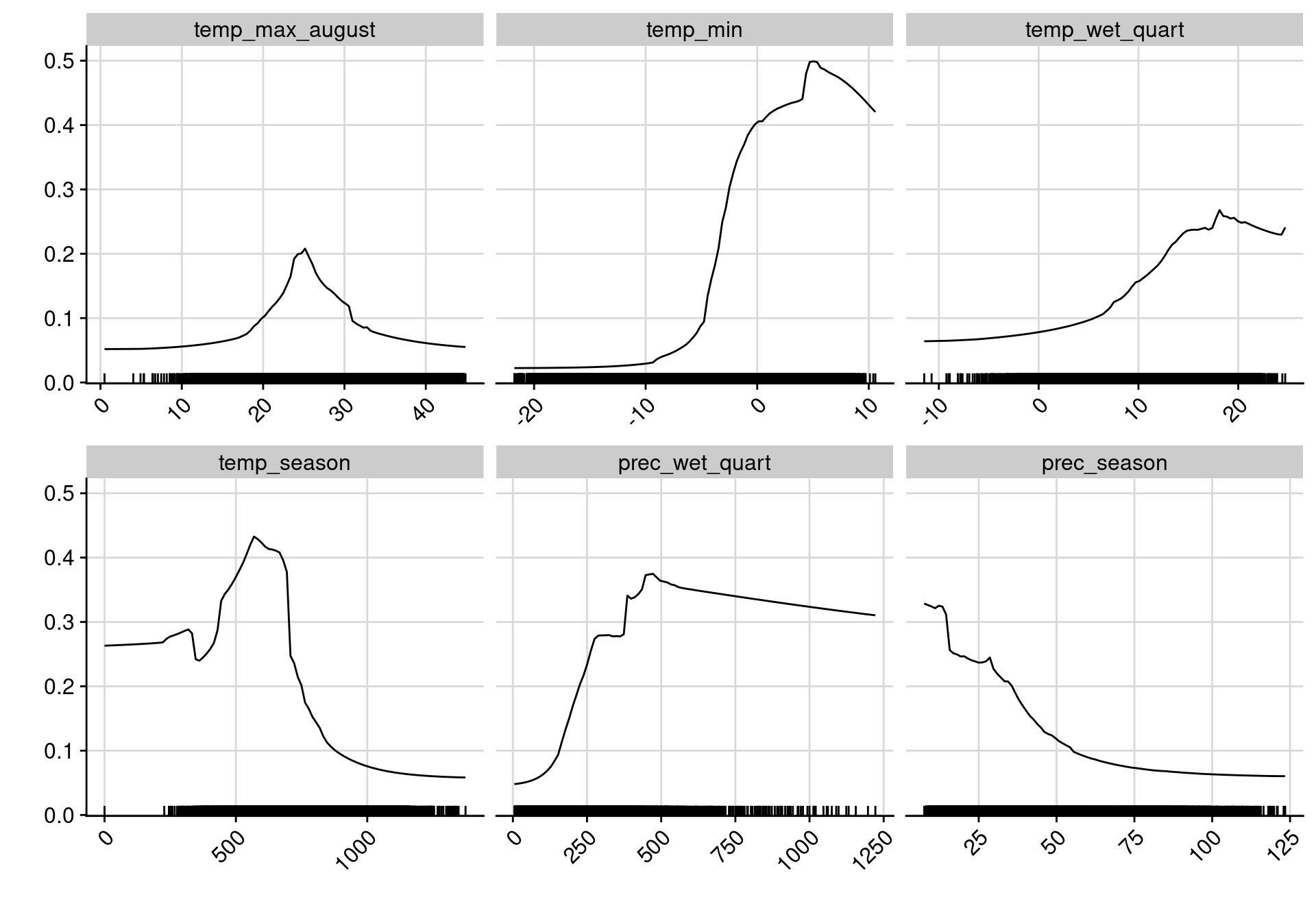
Figure 29.13: Courbes de réponse de chaque variable climatique dans le modèle d’ensemble.
29.3 Projections
29.3.1 Distribution potentielle contemporaine
Contemporaine : Cartographie des observations sur couches climatiques Extraction des conditions climatiques favorables
-=-=-=-=-=-=-=-=-=-=-=-=-=-= BIOMOD.projection.out -=-=-=-=-=-=-=-=-=-=-=-=-=-=
Projection directory : Output/plhi/current
sp.name : plhi
expl.var.names : temp_max_august temp_min temp_wet_quart temp_season
prec_wet_quart prec_season
modeling.id : AllModels ( Output/plhi/plhi.AllModels.models.out )
models.projected :
plhi_PA1_RUN1_GAM, plhi_PA1_RUN1_MARS, plhi_PA1_RUN1_MAXNET, plhi_PA1_RUN1_GBM, plhi_PA1_RUN1_ANN, plhi_PA1_RUN1_RF, plhi_PA2_RUN1_GAM, plhi_PA2_RUN1_MARS, plhi_PA2_RUN1_MAXNET, plhi_PA2_RUN1_GBM, plhi_PA2_RUN1_ANN, plhi_PA2_RUN1_RF, plhi_PA3_RUN1_GAM, plhi_PA3_RUN1_MARS, plhi_PA3_RUN1_MAXNET, plhi_PA3_RUN1_GBM, plhi_PA3_RUN1_ANN, plhi_PA3_RUN1_RF
available binary projection : TSS, ROC
available filtered projection : TSS, ROC
-=-=-=-=-=-=-=-=-=-=-=-=-=-=-=-=-=-=-=-=-=-=-=-=-=-=-=-=-=-=-=-=-=-=-=-=-=-=-=-=
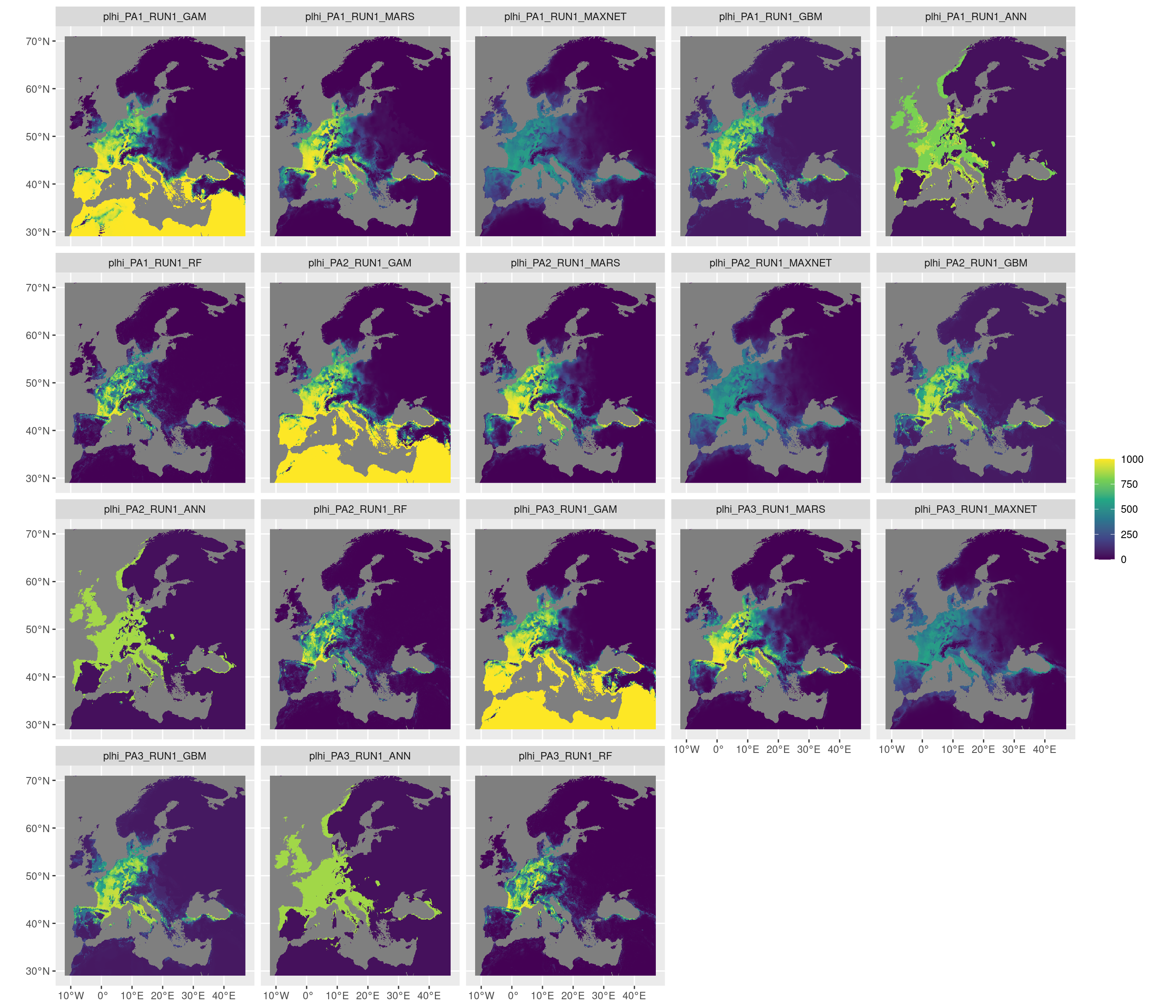
Figure 29.14: Projection de la distribution potentielle contemporaine sur l’Europe, selon les 6 algorithmes et les 3 runs.
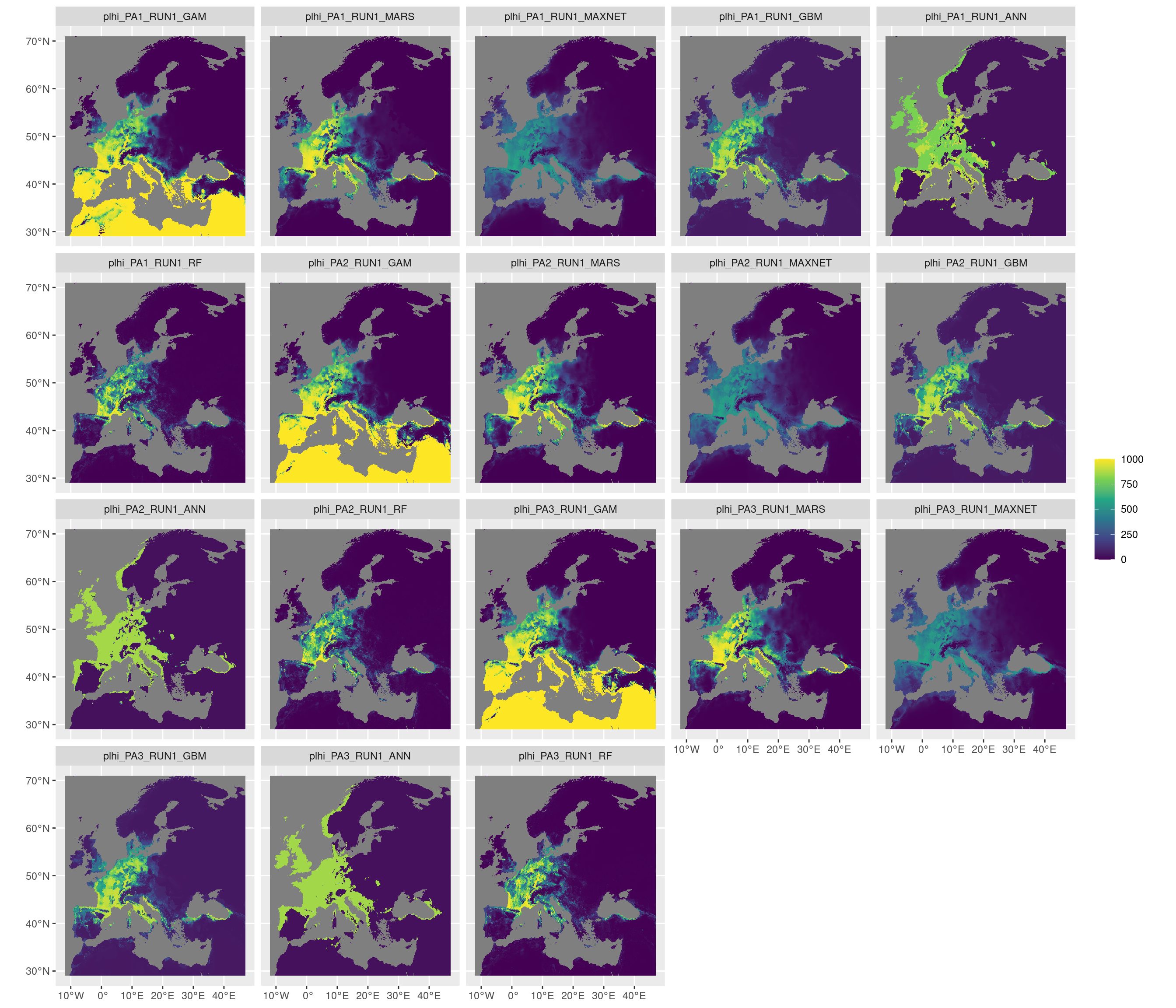
Figure 29.15: Projection de la distribution potentielle contemporaine sur l’Europe, selon les 6 algorithmes et les 3 runs.
-=-=-=-=-=-=-=-=-=-=-=-=-=-= BIOMOD.projection.out -=-=-=-=-=-=-=-=-=-=-=-=-=-=
Projection directory : Output/plhi/current
sp.name : plhi
expl.var.names : temp_max_august temp_min temp_wet_quart temp_season
prec_wet_quart prec_season
modeling.id : AllModels ( Output/plhi/plhi.AllModels.ensemble.models.out )
models.projected :
plhi_EMcvByTSS_mergedData_mergedRun_mergedAlgo, plhi_EMwmeanByTSS_mergedData_mergedRun_mergedAlgo
-=-=-=-=-=-=-=-=-=-=-=-=-=-=-=-=-=-=-=-=-=-=-=-=-=-=-=-=-=-=-=-=-=-=-=-=-=-=-=-=
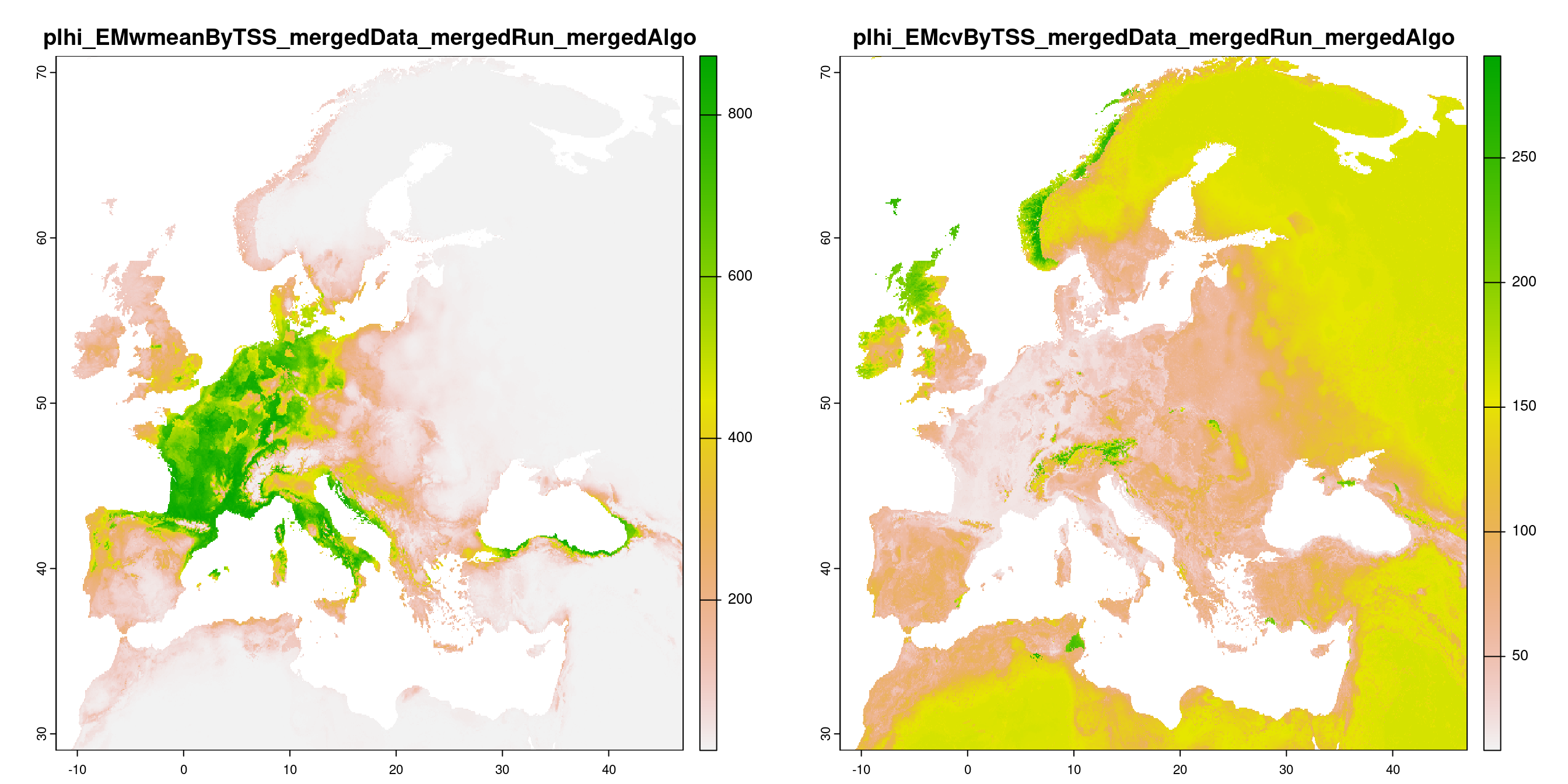
Figure 29.16: A) Projection de la distribution potentielle contemporaine (modèle d’ensemble) ; B) Incertitude associée à la projection.
29.3.2 Interpolation à l’échelle de la métropole
- → conditions climatiques favorables à fine échelle
On projette la niche climatique sur ce raster à fine échelle.
-=-=-=-=-=-=-=-=-=-=-=-=-=-= BIOMOD.projection.out -=-=-=-=-=-=-=-=-=-=-=-=-=-=
Projection directory : Output/plhi/cont_gre
sp.name : plhi
expl.var.names : temp_max_august temp_min temp_wet_quart temp_season
prec_wet_quart prec_season
modeling.id : AllModels ( Output/plhi/plhi.AllModels.models.out )
models.projected :
plhi_PA1_RUN1_GAM, plhi_PA1_RUN1_MARS, plhi_PA1_RUN1_MAXNET, plhi_PA1_RUN1_GBM, plhi_PA1_RUN1_ANN, plhi_PA1_RUN1_RF, plhi_PA2_RUN1_GAM, plhi_PA2_RUN1_MARS, plhi_PA2_RUN1_MAXNET, plhi_PA2_RUN1_GBM, plhi_PA2_RUN1_ANN, plhi_PA2_RUN1_RF, plhi_PA3_RUN1_GAM, plhi_PA3_RUN1_MARS, plhi_PA3_RUN1_MAXNET, plhi_PA3_RUN1_GBM, plhi_PA3_RUN1_ANN, plhi_PA3_RUN1_RF
available binary projection : TSS, ROC
available filtered projection : TSS, ROC
-=-=-=-=-=-=-=-=-=-=-=-=-=-=-=-=-=-=-=-=-=-=-=-=-=-=-=-=-=-=-=-=-=-=-=-=-=-=-=-=
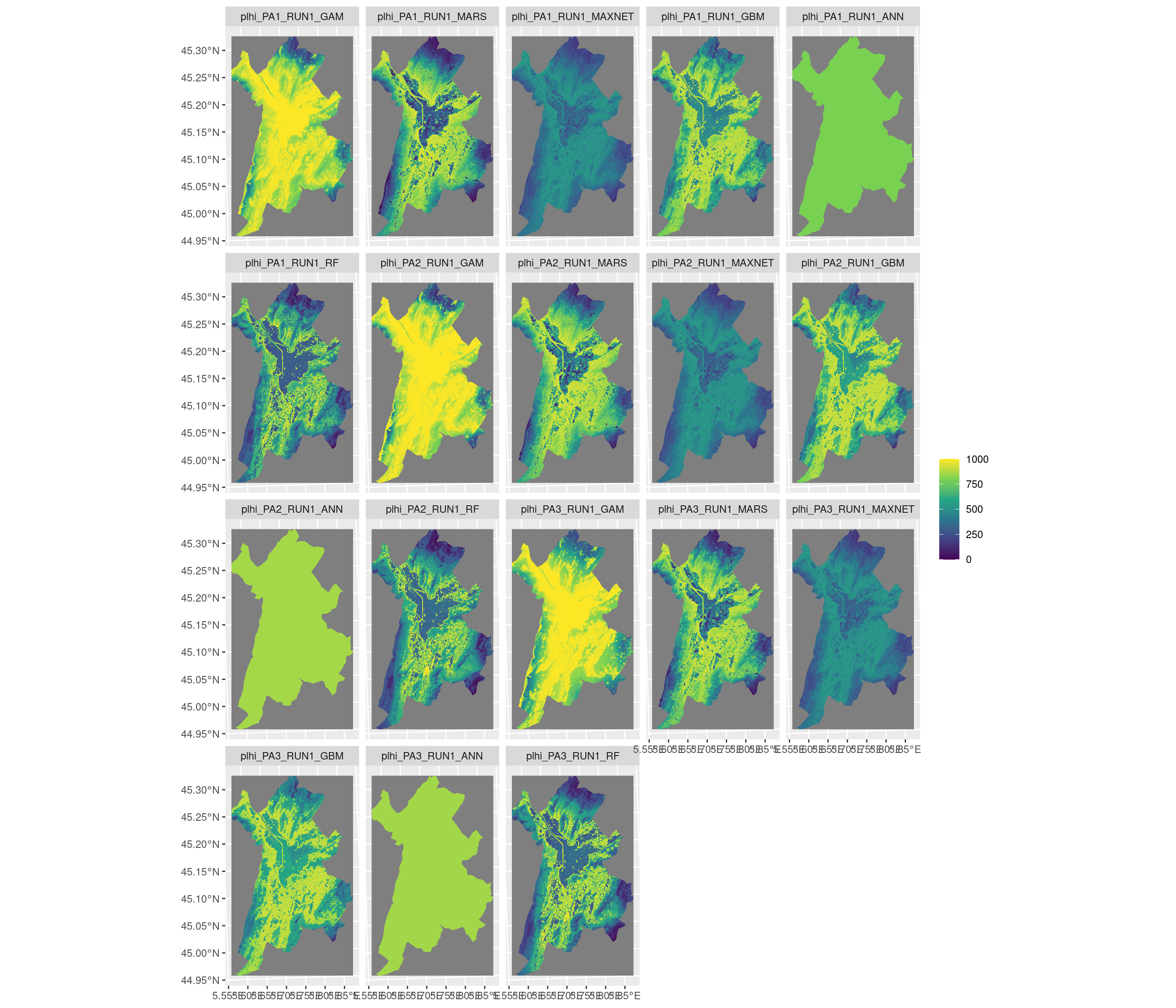
Figure 29.17: Projection de la distribution potentielle contemporaine sur la métropole de Grenoble, selon les 6 algorithmes et les 3 runs.
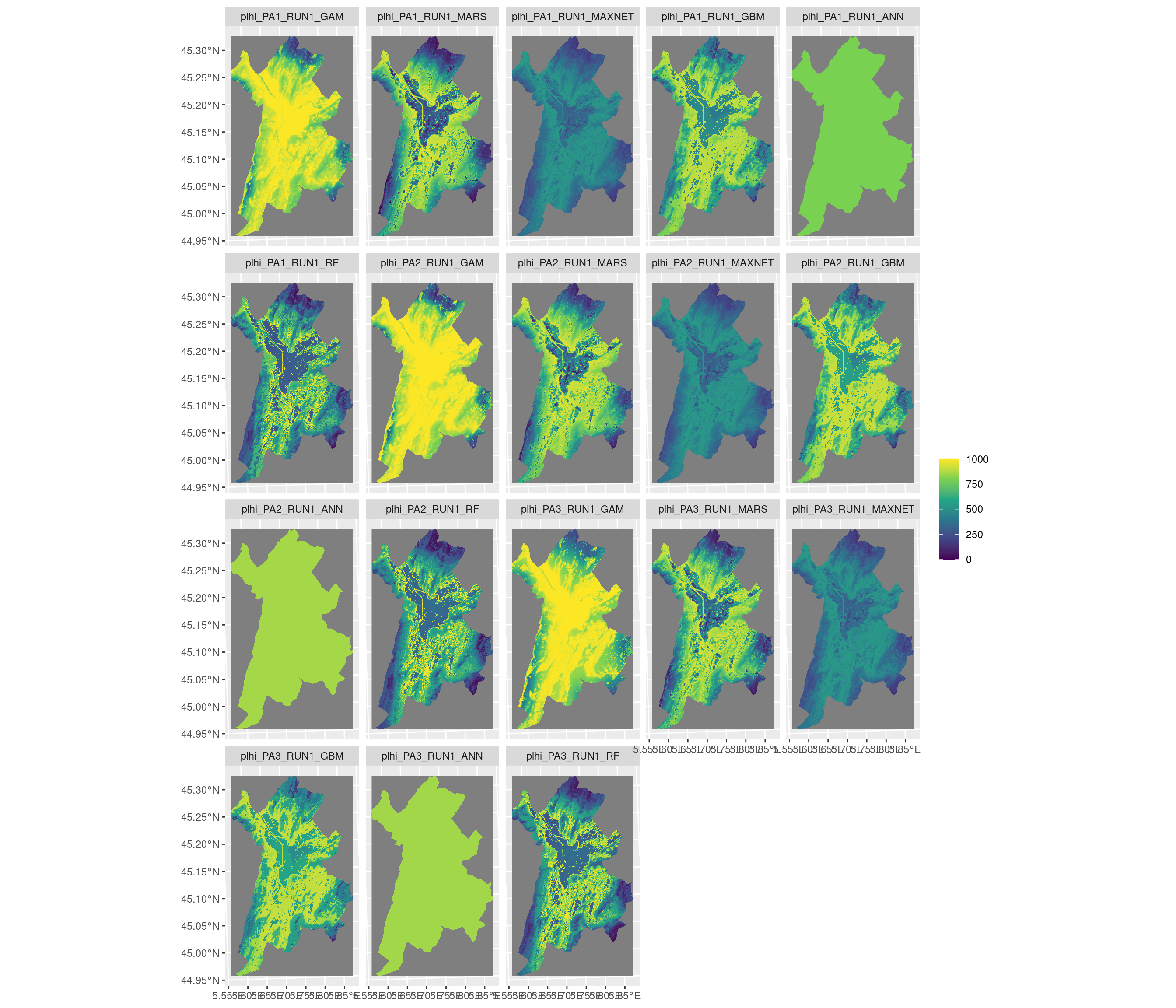
Figure 29.18: Projection de la distribution potentielle contemporaine sur la métropole de Grenoble, selon les 6 algorithmes et les 3 runs.
-=-=-=-=-=-=-=-=-=-=-=-=-=-= BIOMOD.projection.out -=-=-=-=-=-=-=-=-=-=-=-=-=-=
Projection directory : Output/plhi/cont_gre
sp.name : plhi
expl.var.names : temp_max_august temp_min temp_wet_quart temp_season
prec_wet_quart prec_season
modeling.id : AllModels ( Output/plhi/plhi.AllModels.ensemble.models.out )
models.projected :
plhi_EMcvByTSS_mergedData_mergedRun_mergedAlgo, plhi_EMwmeanByTSS_mergedData_mergedRun_mergedAlgo
-=-=-=-=-=-=-=-=-=-=-=-=-=-=-=-=-=-=-=-=-=-=-=-=-=-=-=-=-=-=-=-=-=-=-=-=-=-=-=-=
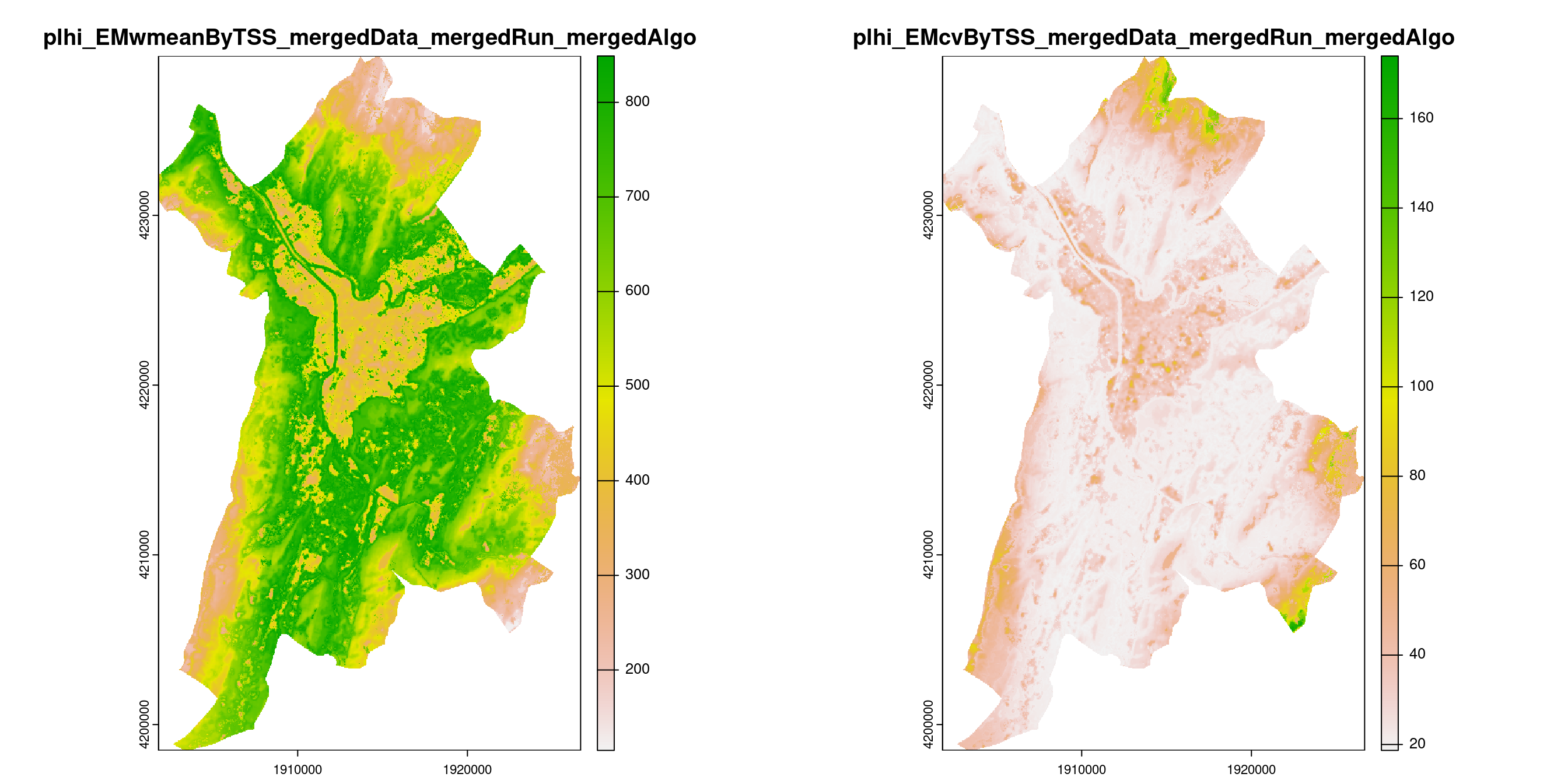
Figure 29.19: A) Projection de la distribution potentielle contemporaine (modèle d’ensemble) sur la métropole de Grenoble ; B) Incertitude associée à la projection.
29.3.3 Distributions potentielles futures
Futur : Projection à l’échelle de l’Europe jusqu’à l’horizon 2100
Toutes les fenêtres temporelles × scénarios pour le GCM « IPSL-CM6A-LR »
- SSP1-2.6
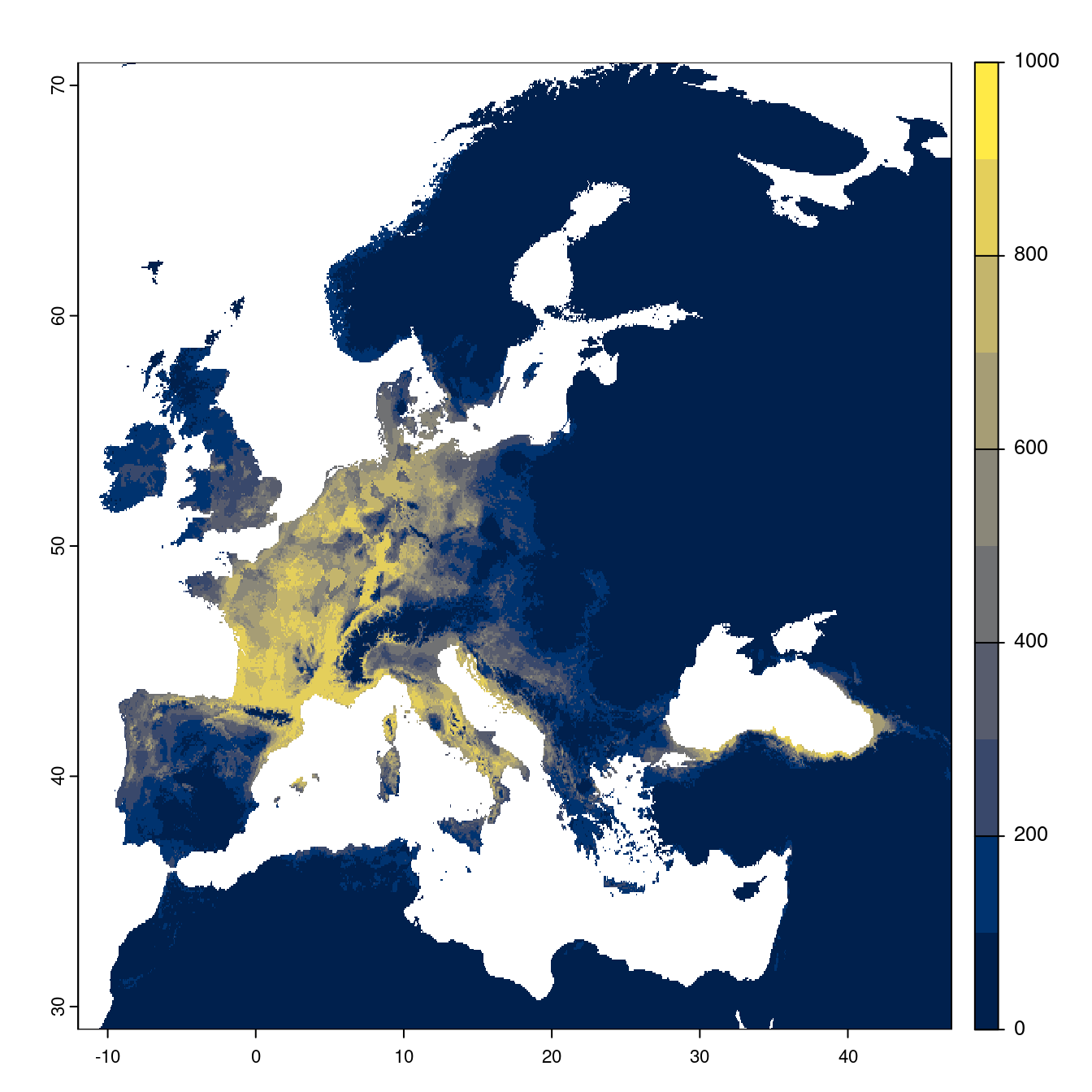
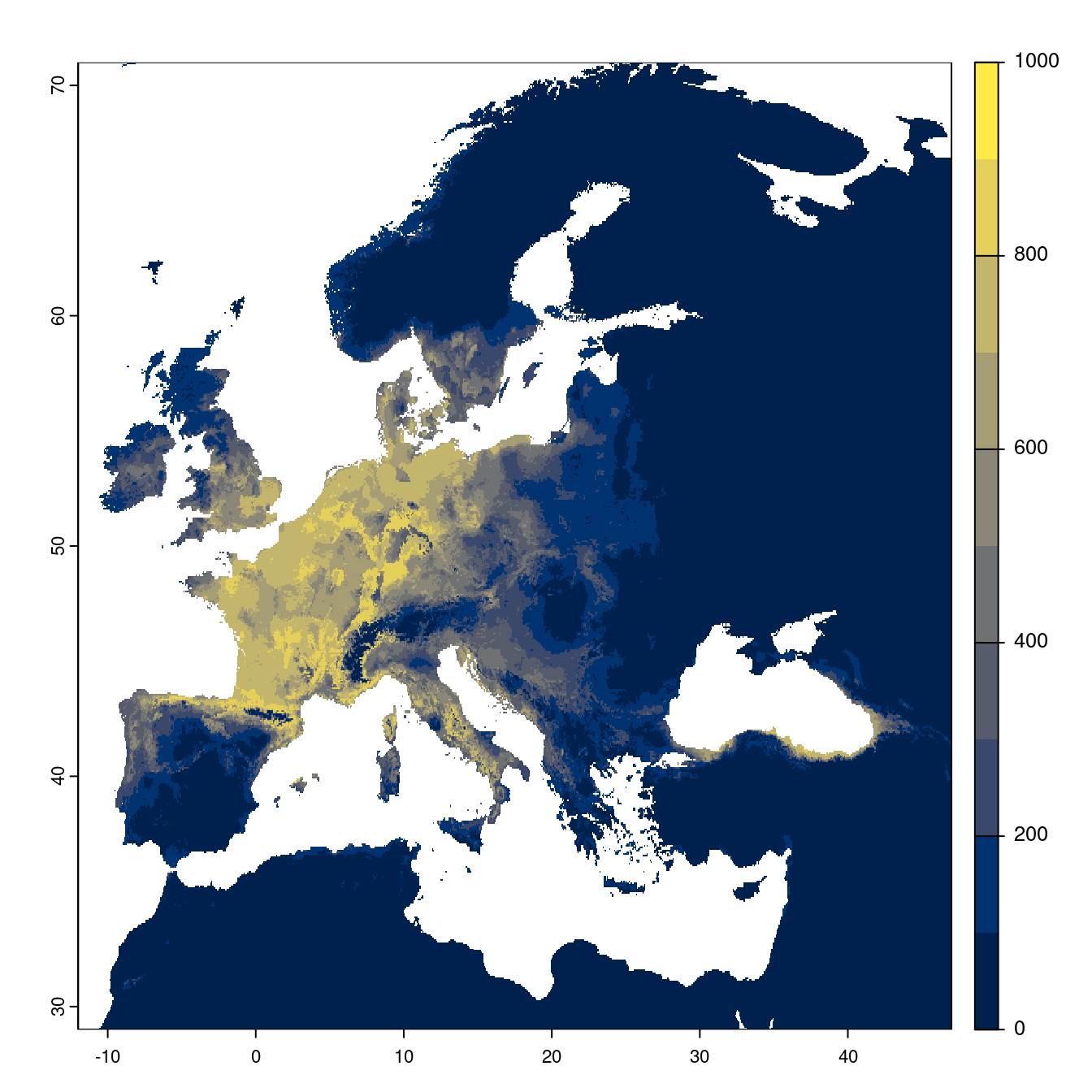
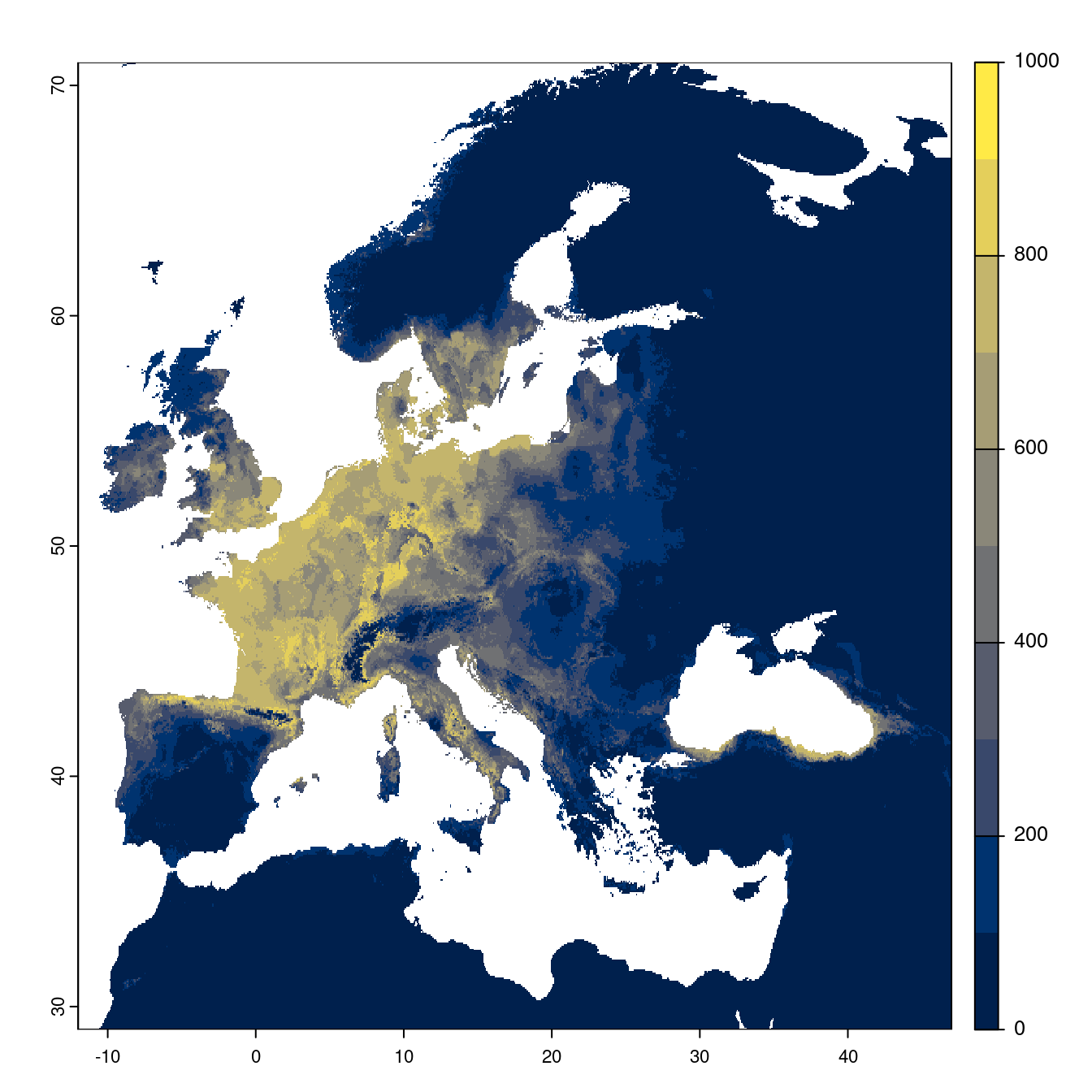
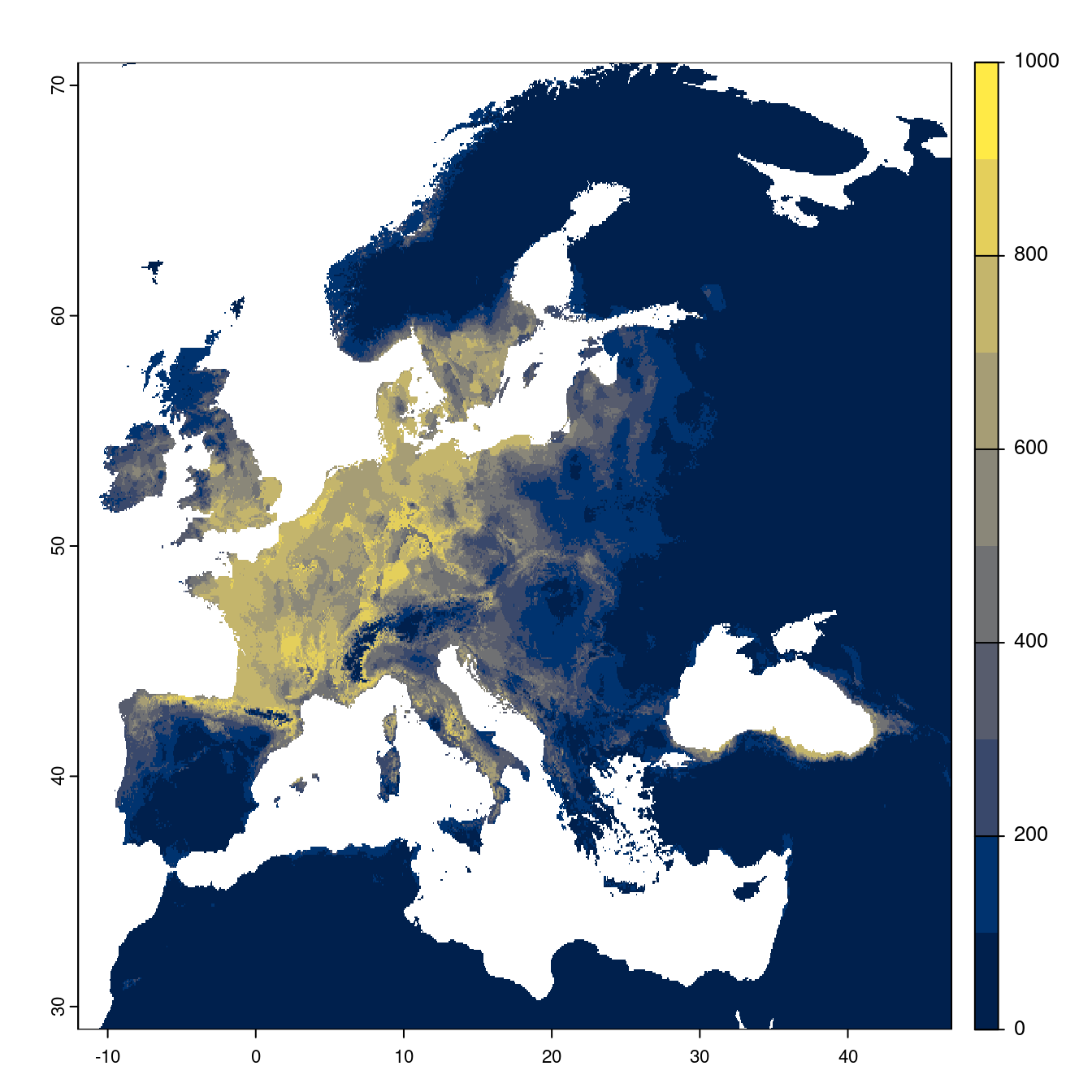
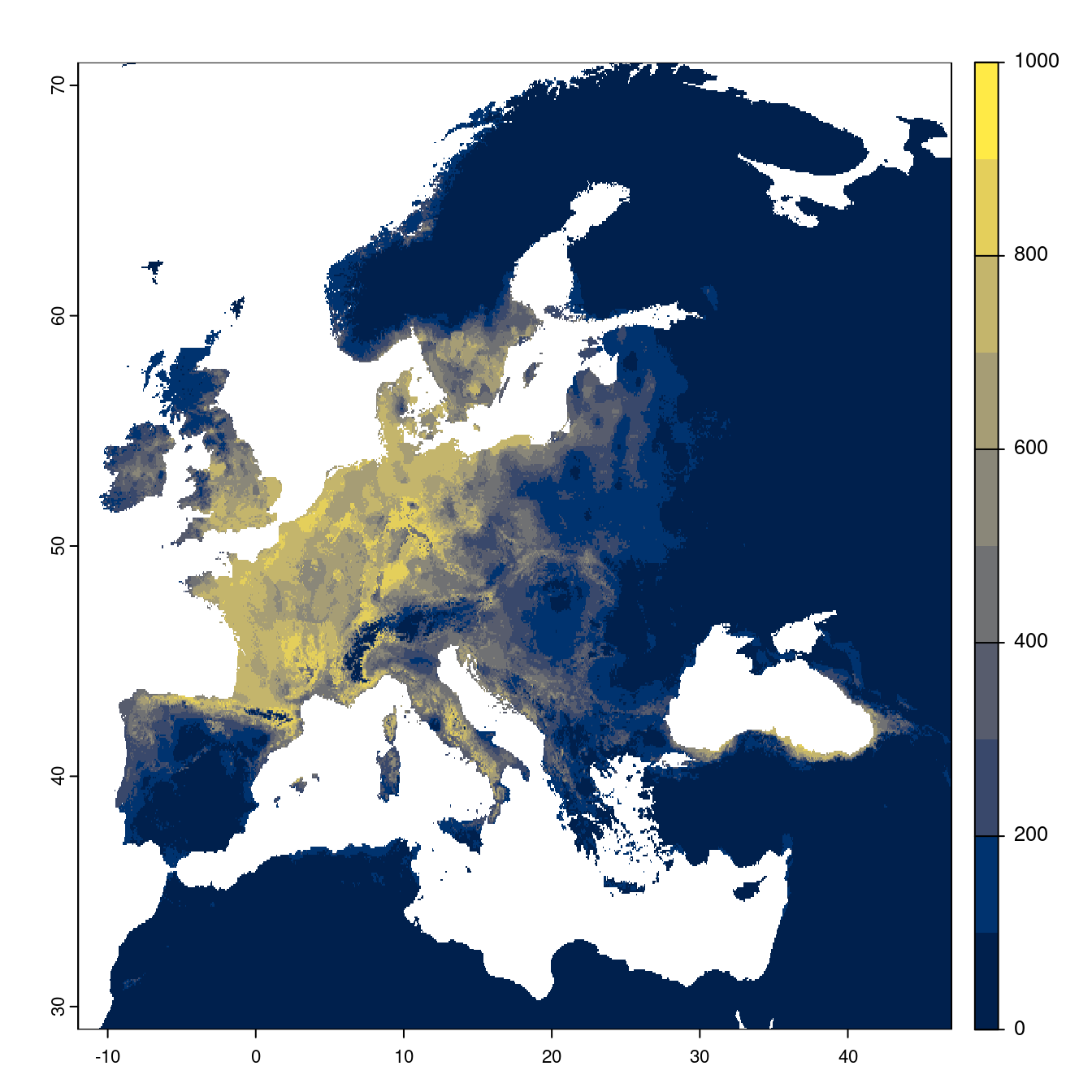
Figure 29.20: Projection des distributions potentielles futures jusqu’à l’horizon 2100 sous le scénario SSP1-2.6 (modèle d’ensemble).
On obtient pour la métrique TSS une carte unique de moyenne pondérée sur les algorithmes et sur les jeux de pseudo-absences.
- SSP2-4.5
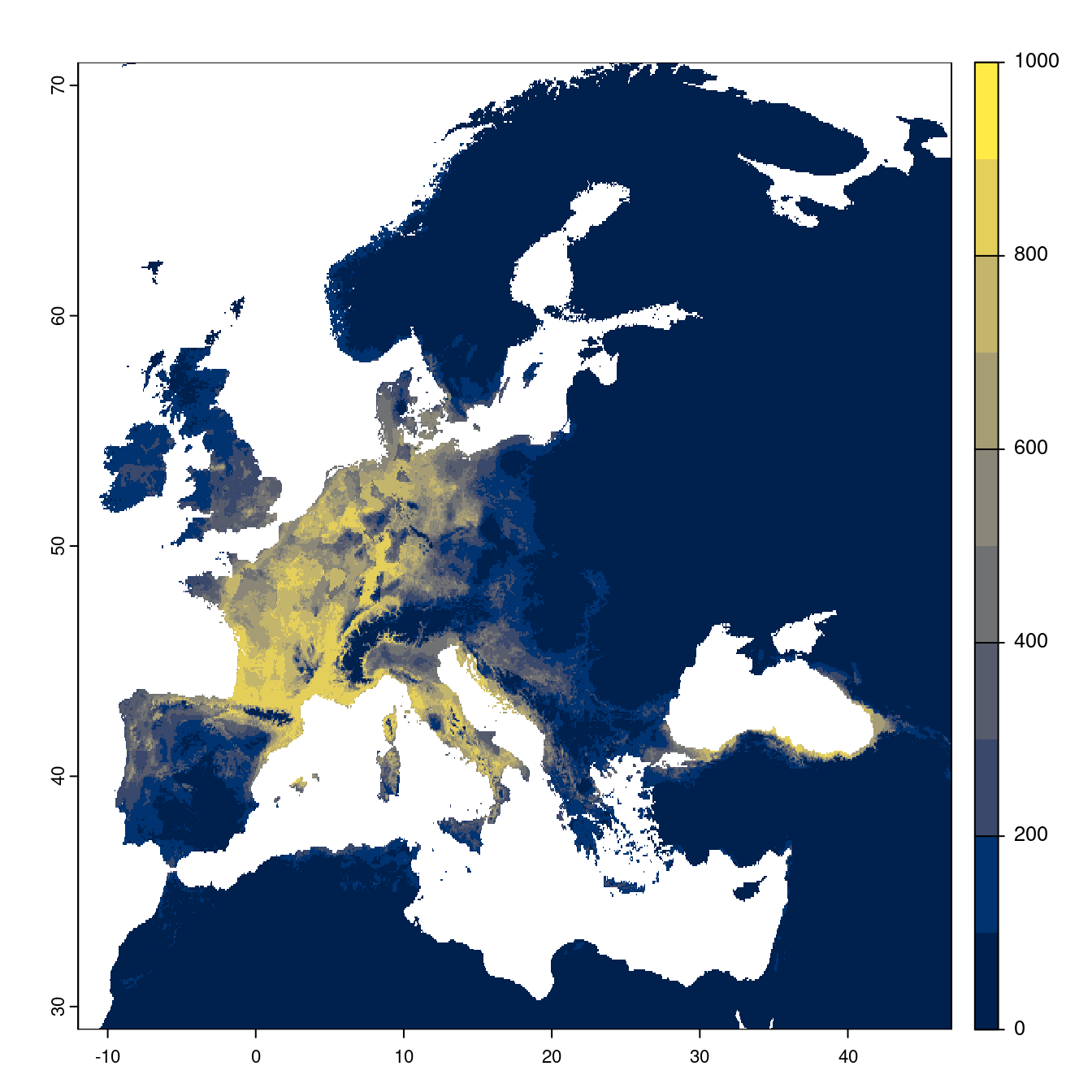
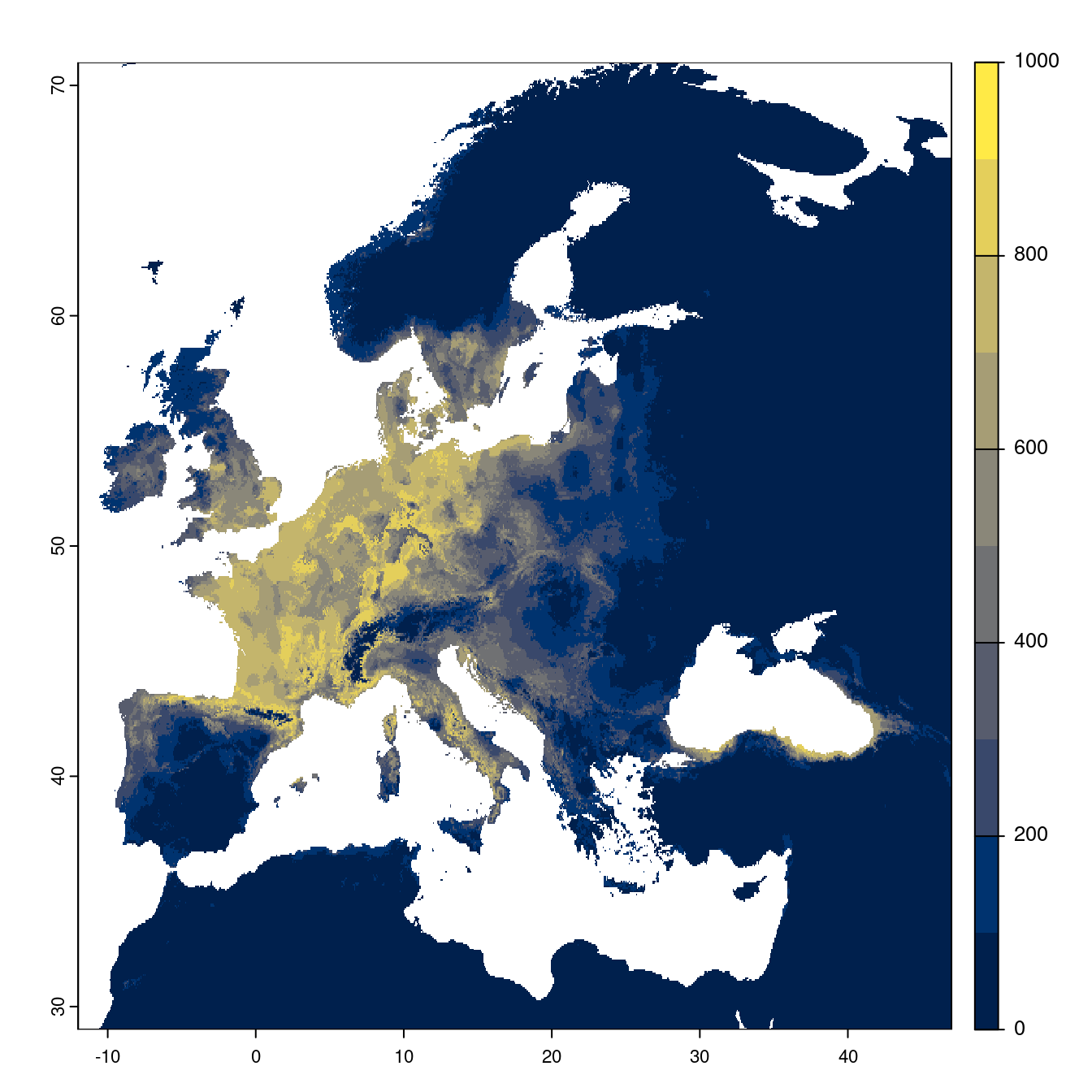
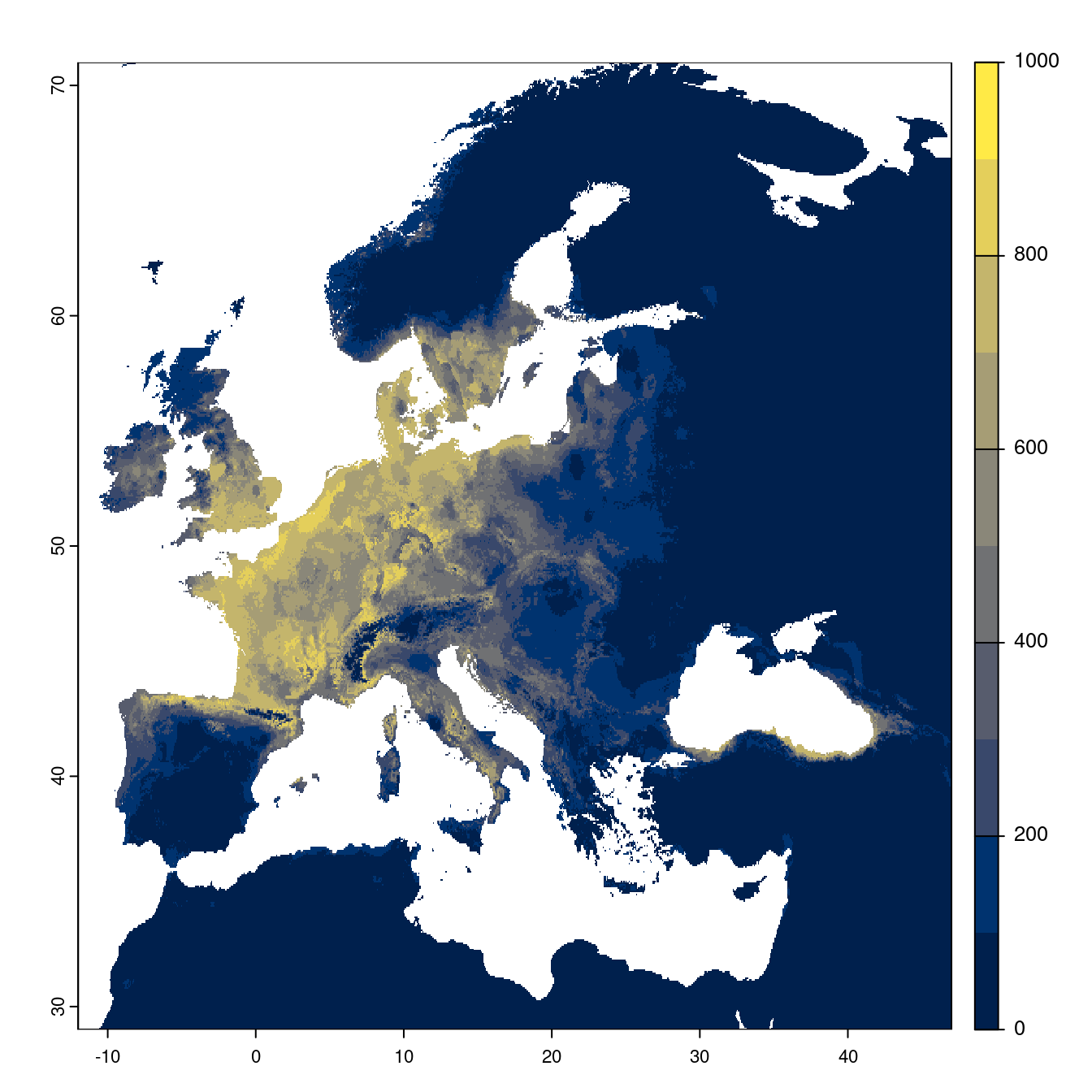
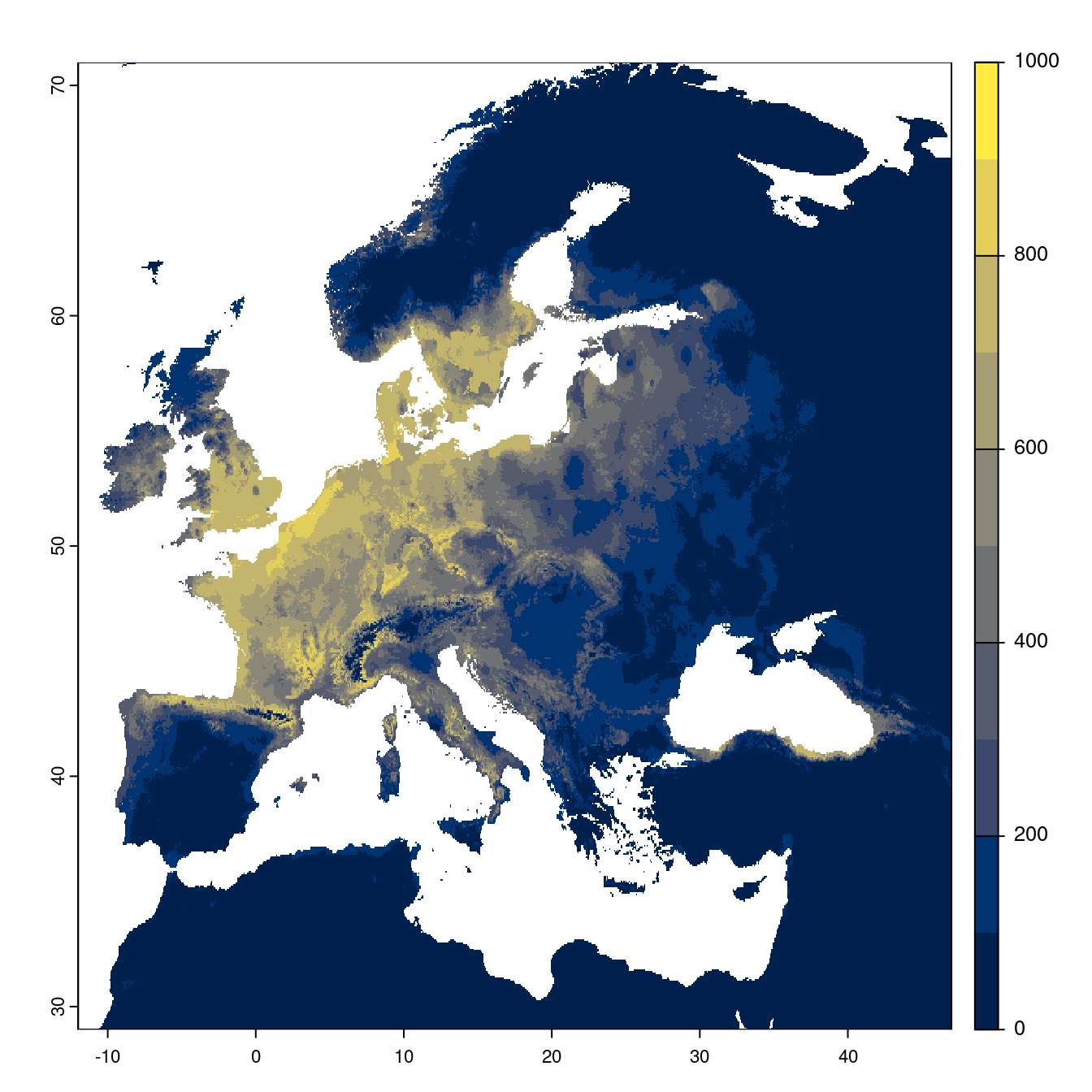
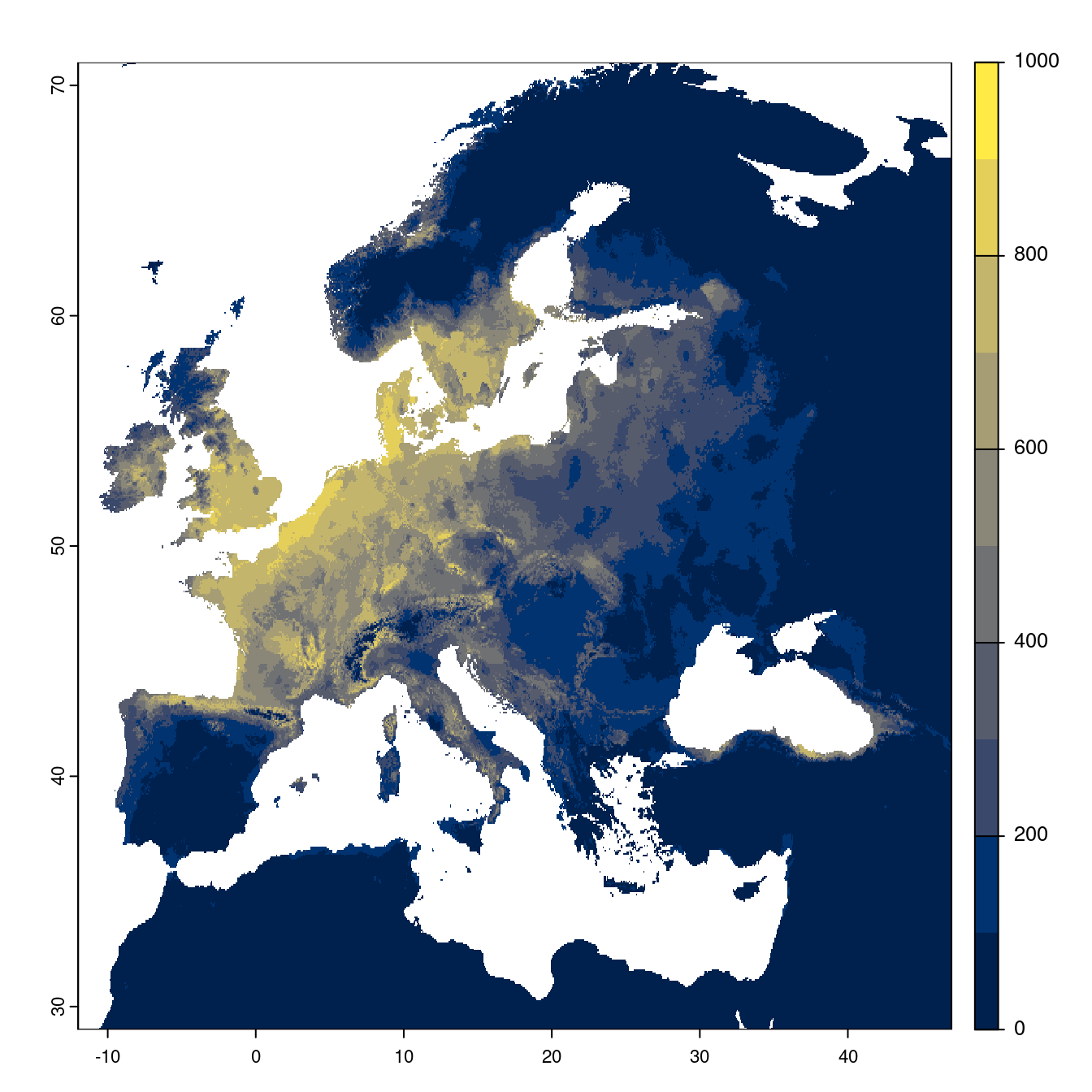
Figure 29.21: Projection des distributions potentielles futures jusqu’à l’horizon 2100 sous le scénario SSP2-4.5 (modèle d’ensemble).
- SSP3-7.0
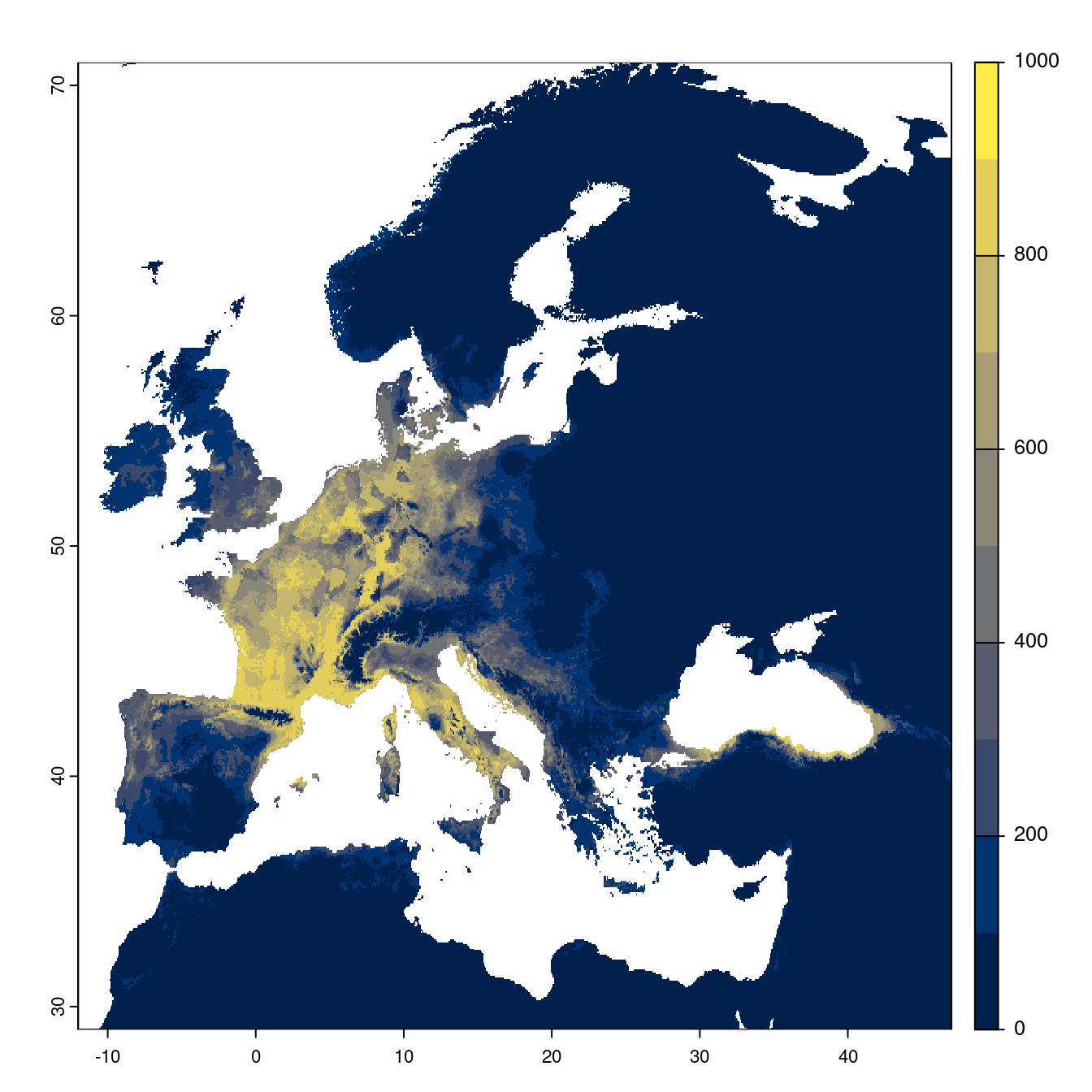
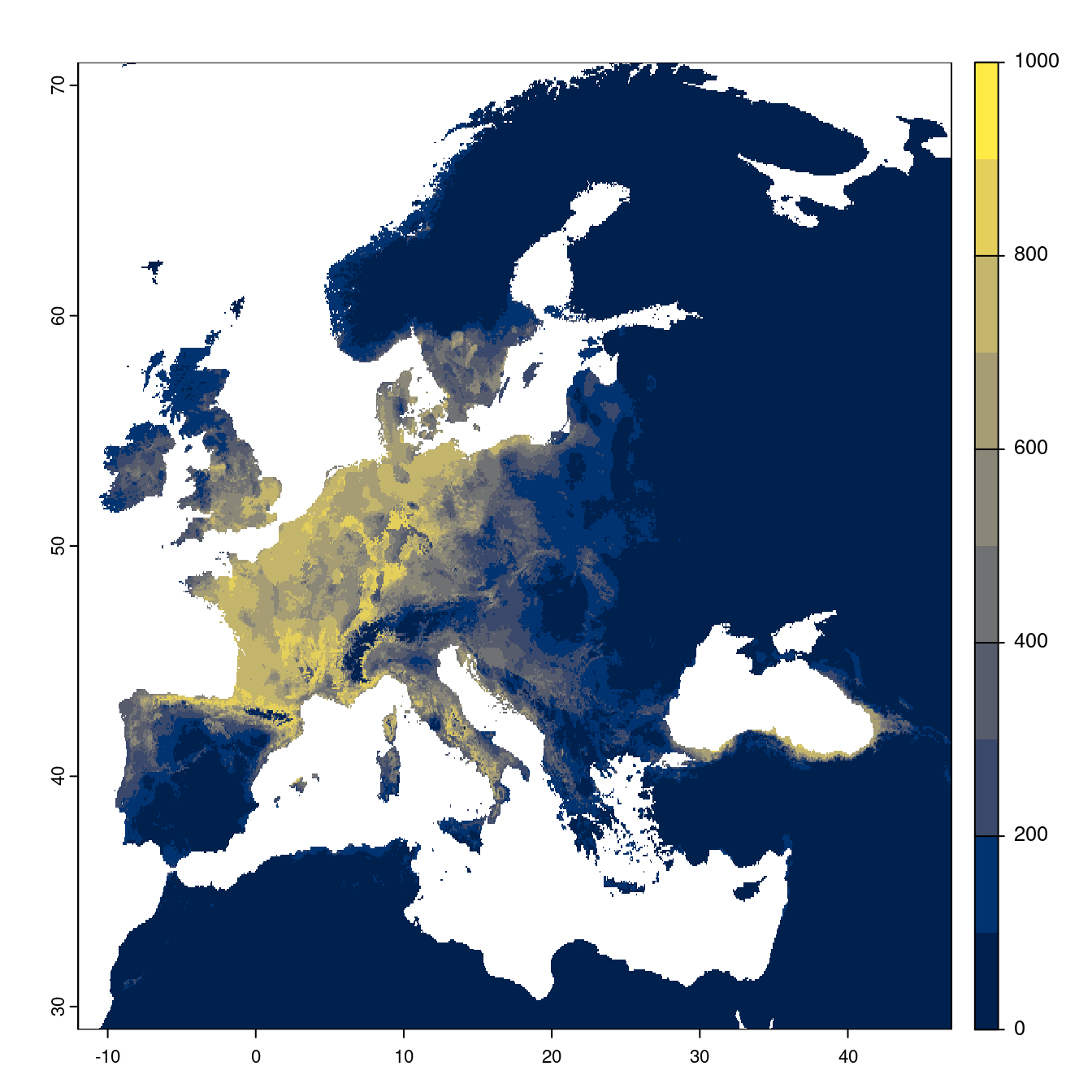
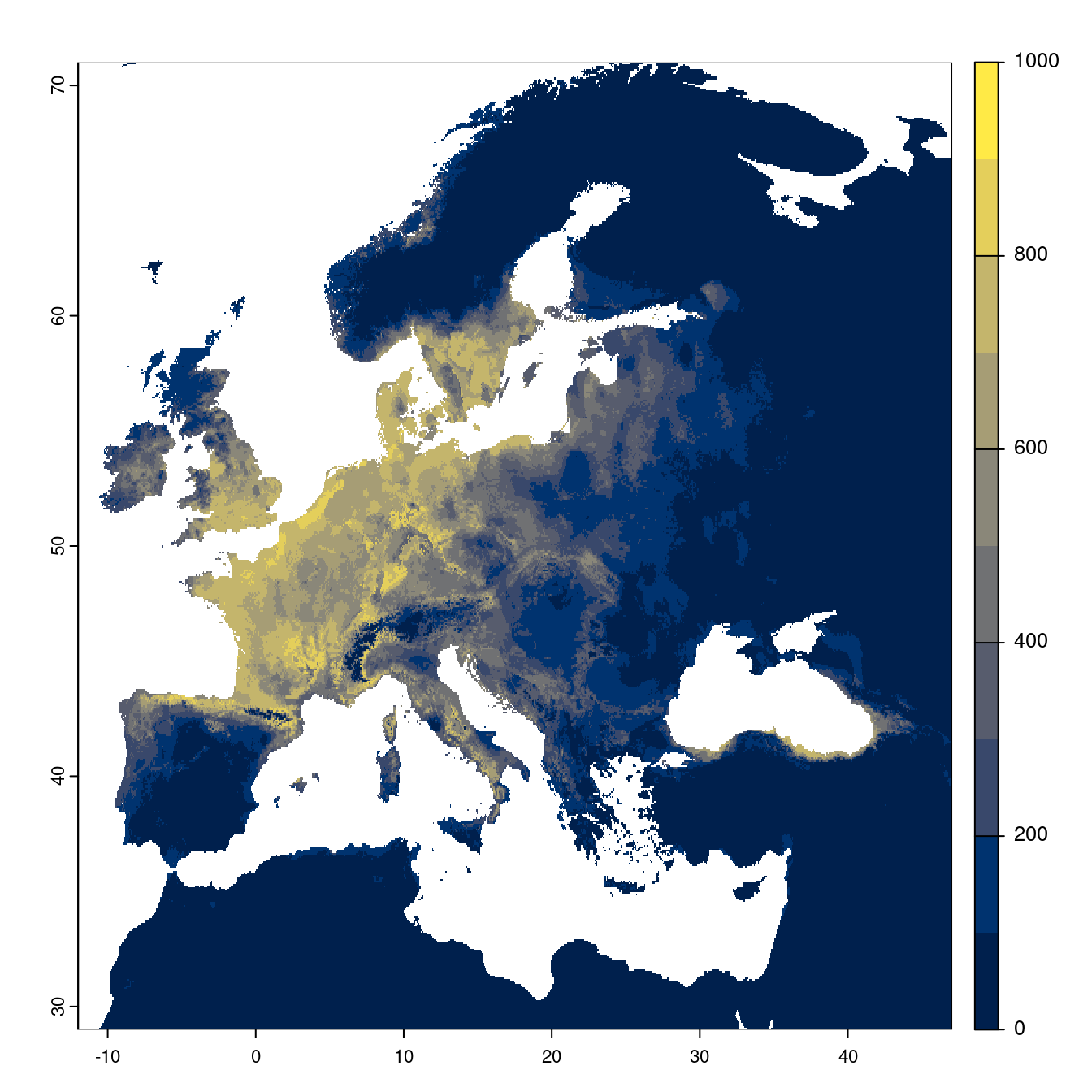
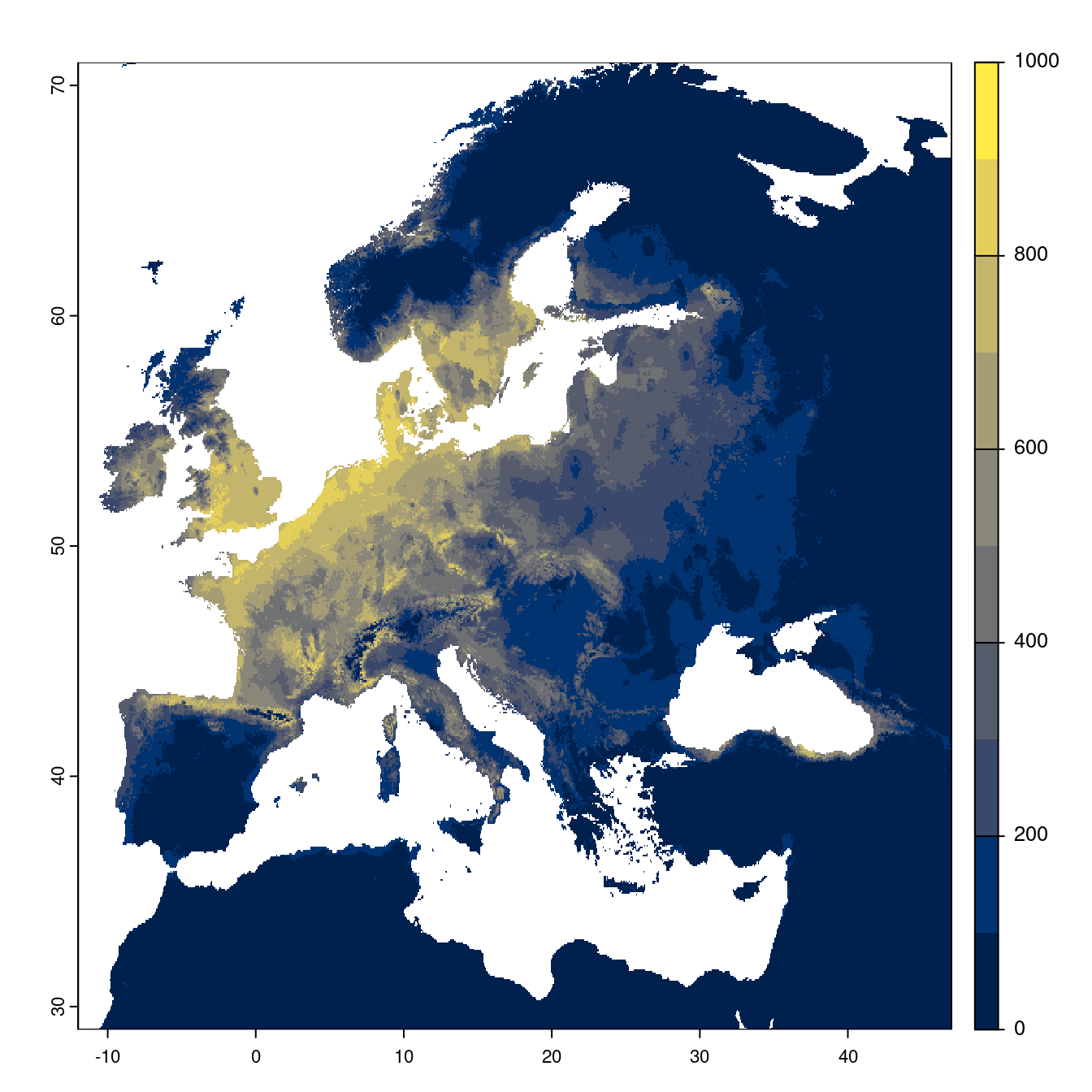
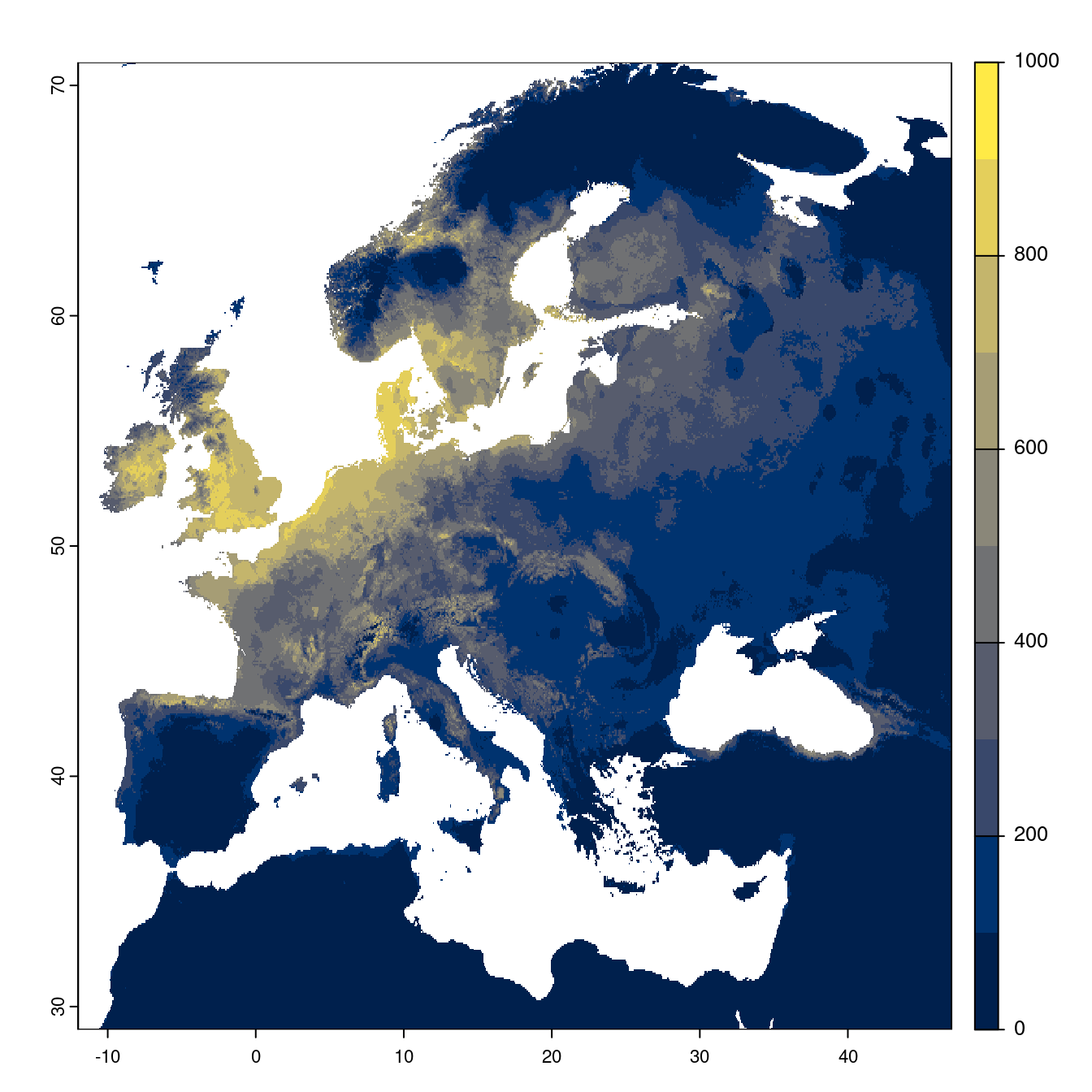
Figure 29.22: Projection des distributions potentielles futures jusqu’à l’horizon 2100 sous le scénario SSP3-7.0 (modèle d’ensemble).
- SSP5-8.5
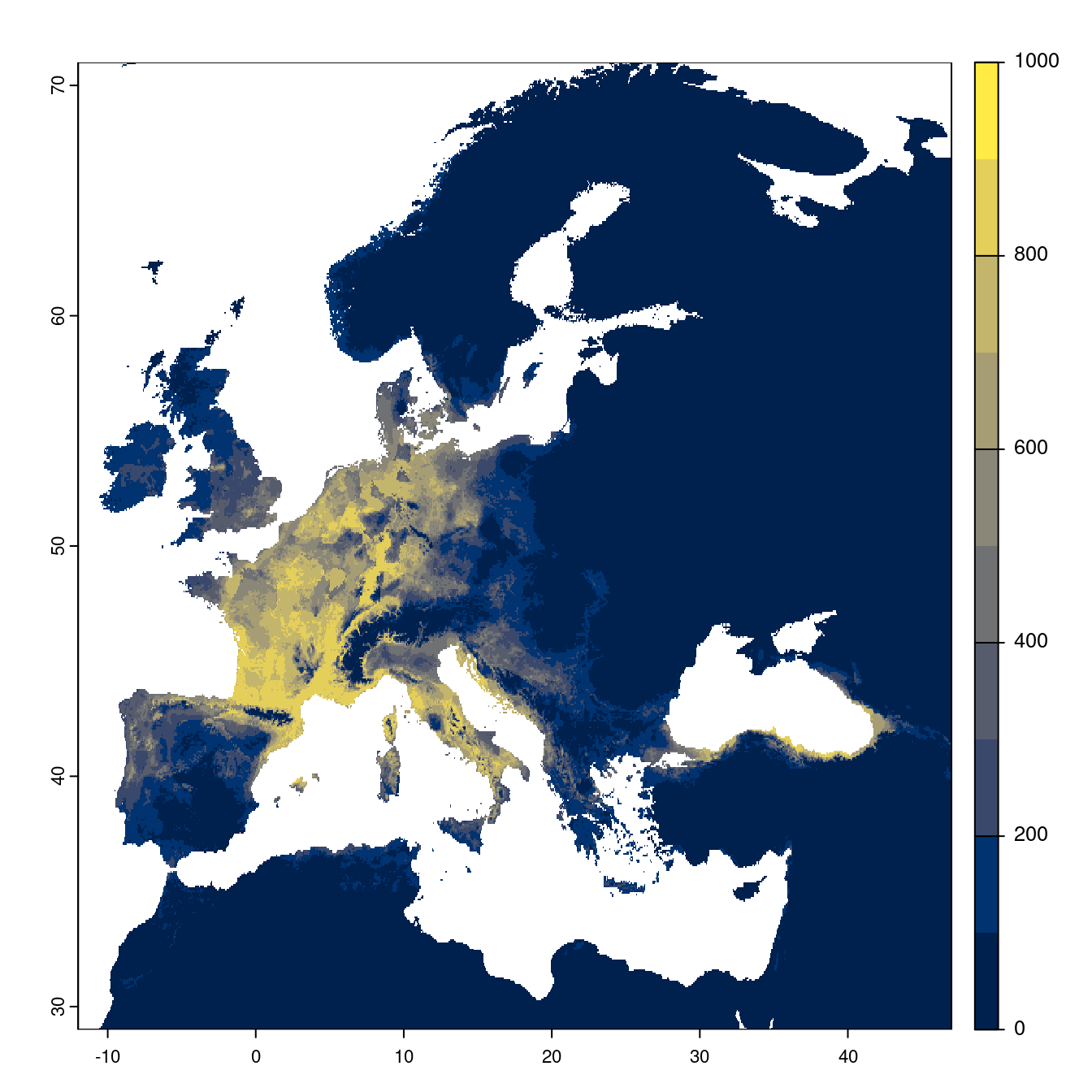
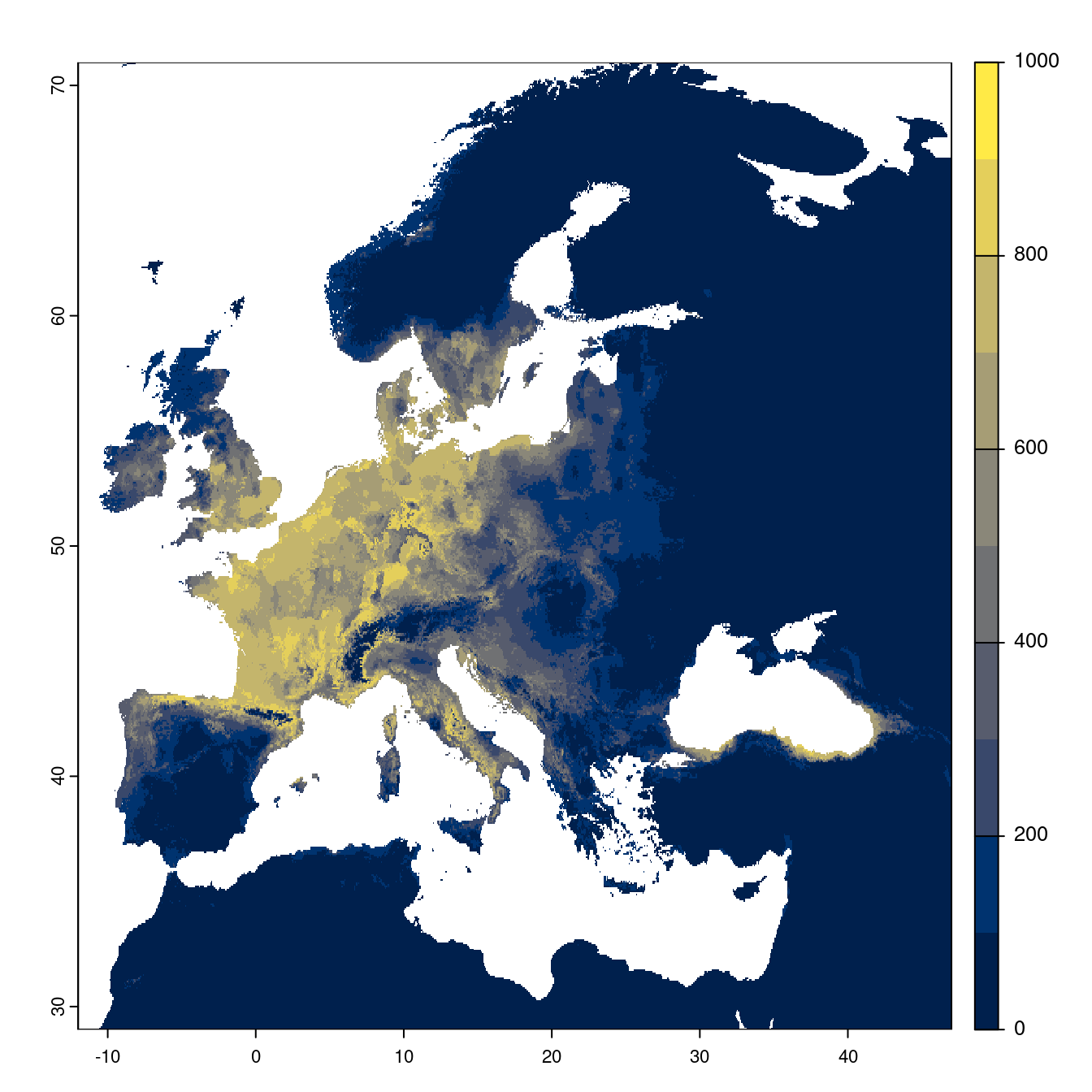
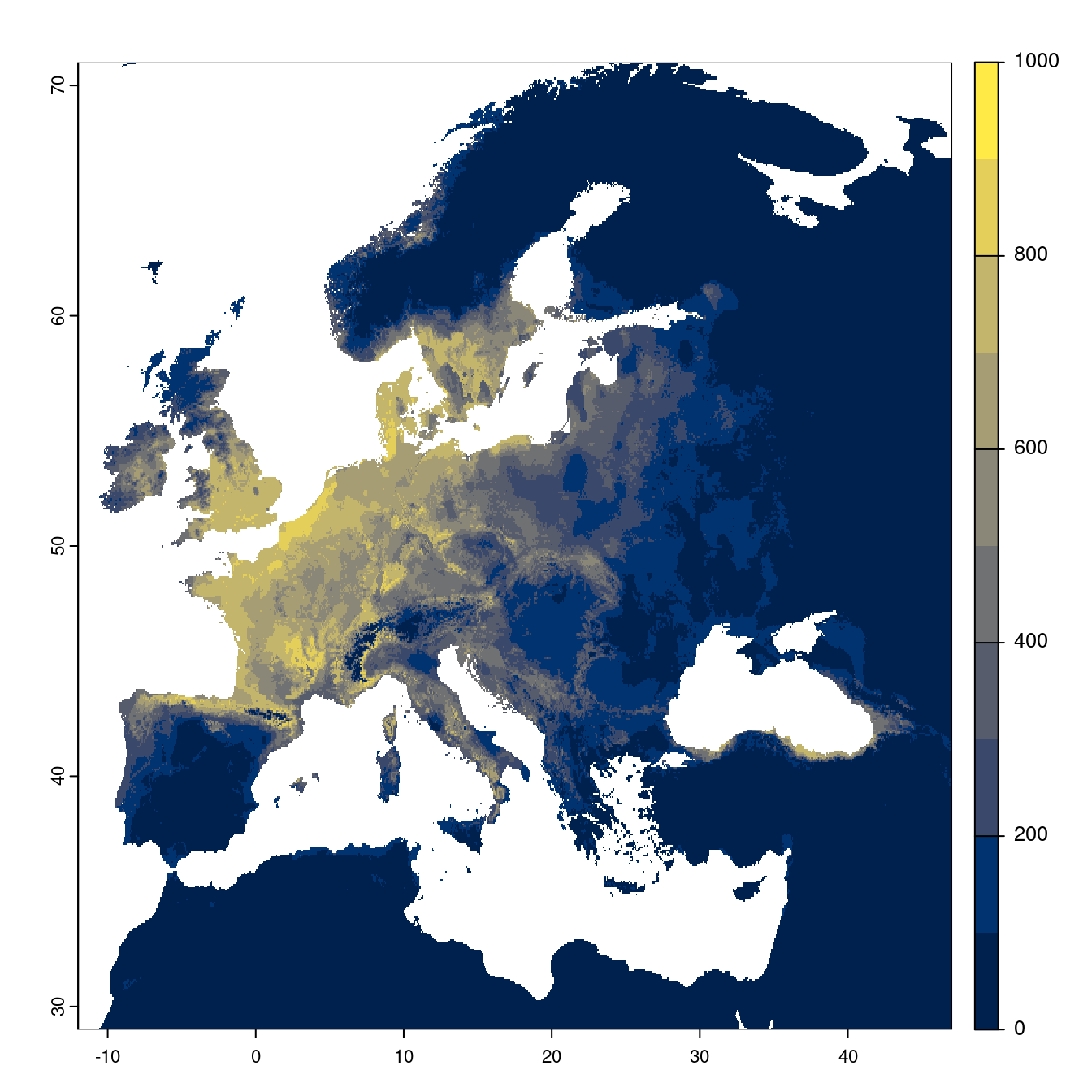
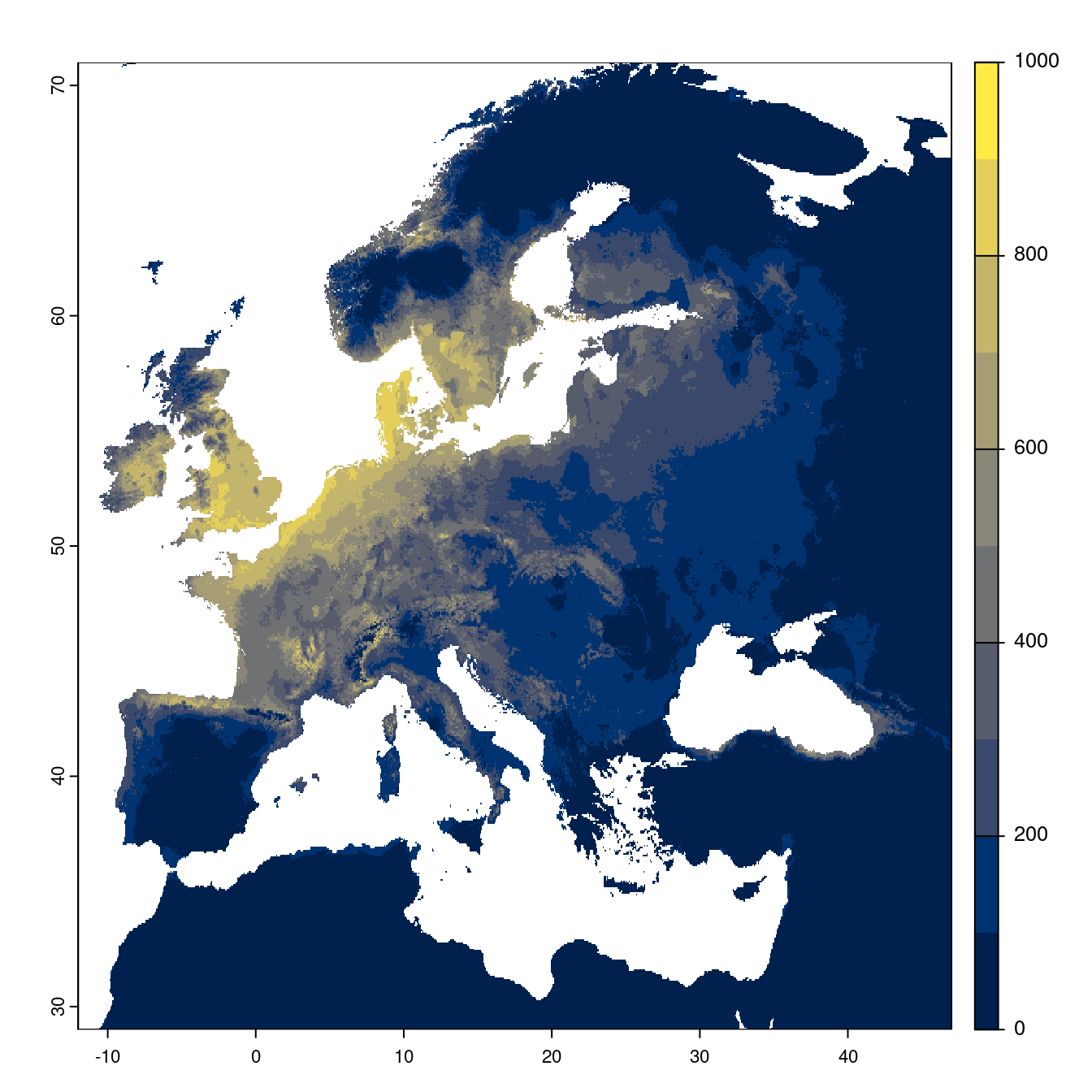
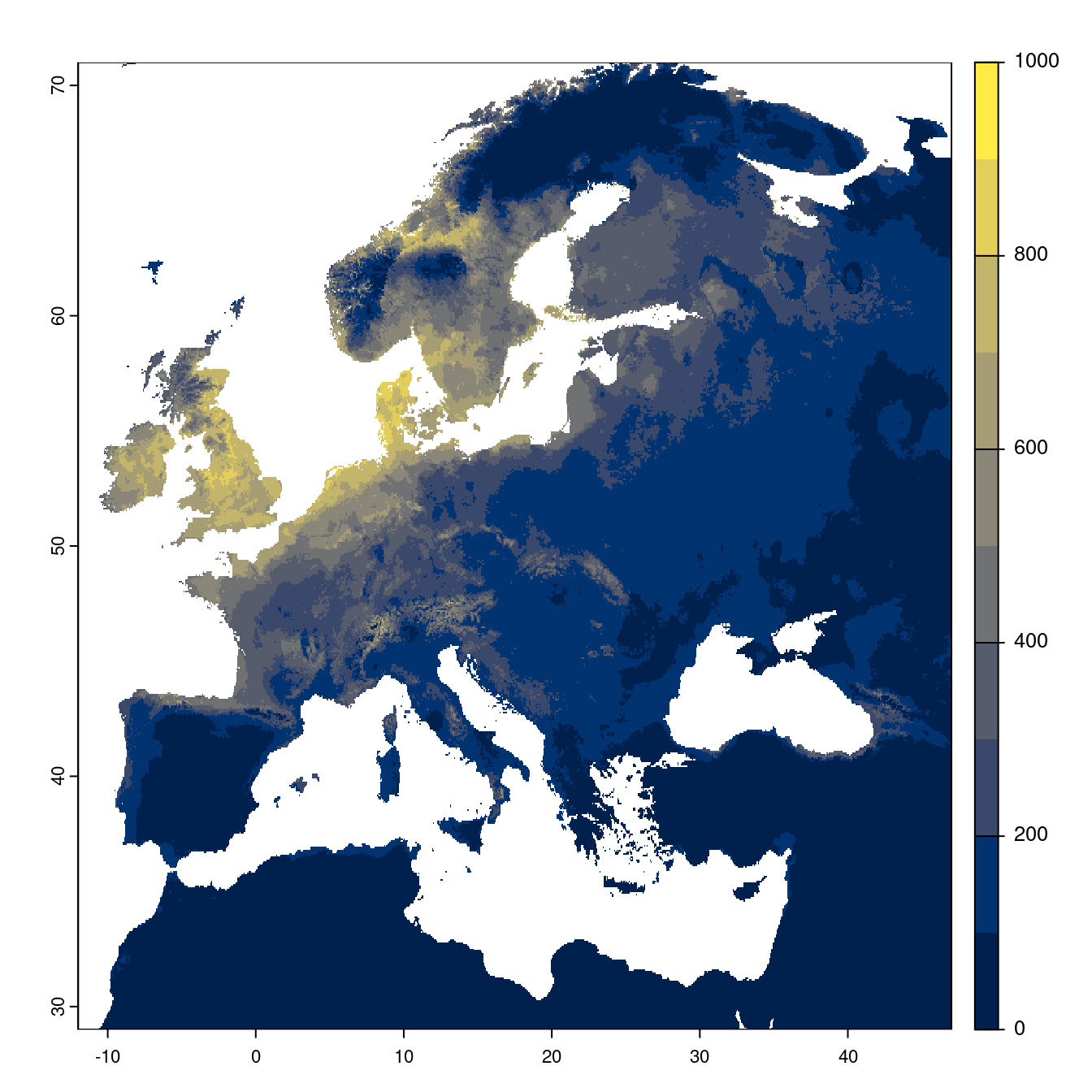
Figure 29.23: Projection des distributions potentielles futures jusqu’à l’horizon 2100 sous le scénario SSP5-8.5 (modèle d’ensemble).
29.4 Inférence à l’échelle de la métropole
- Croisement avec températures de surface 2019 (fournie par la métropole)
- → conditions climatiques favorables à fine échelle
- à peu près 7 pixels × 9 (WorldClim)
29.4.1 Distributions potentielles futures
Même chose que précédemment mais sur les rasters de Grenoble
- SSP1-2.6
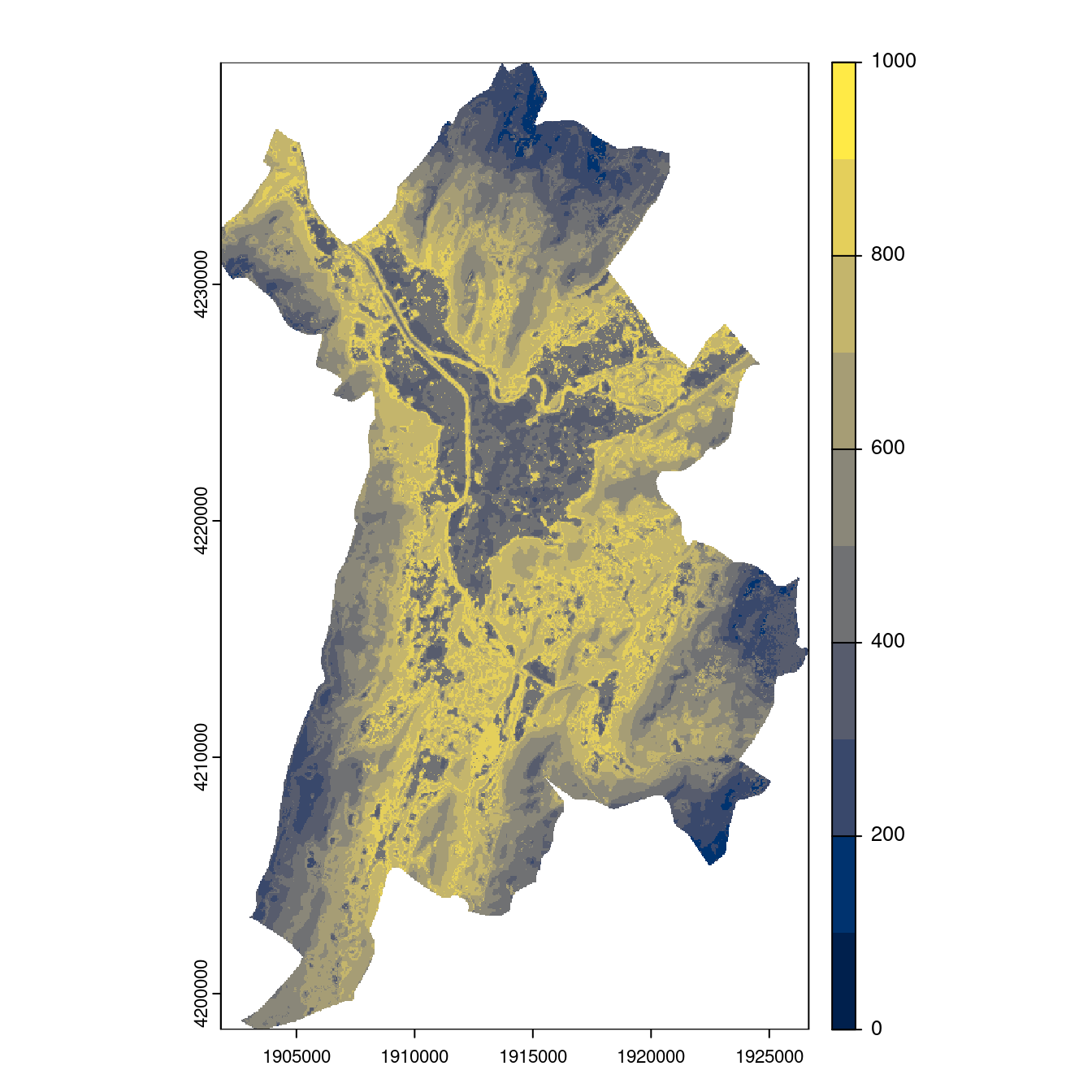
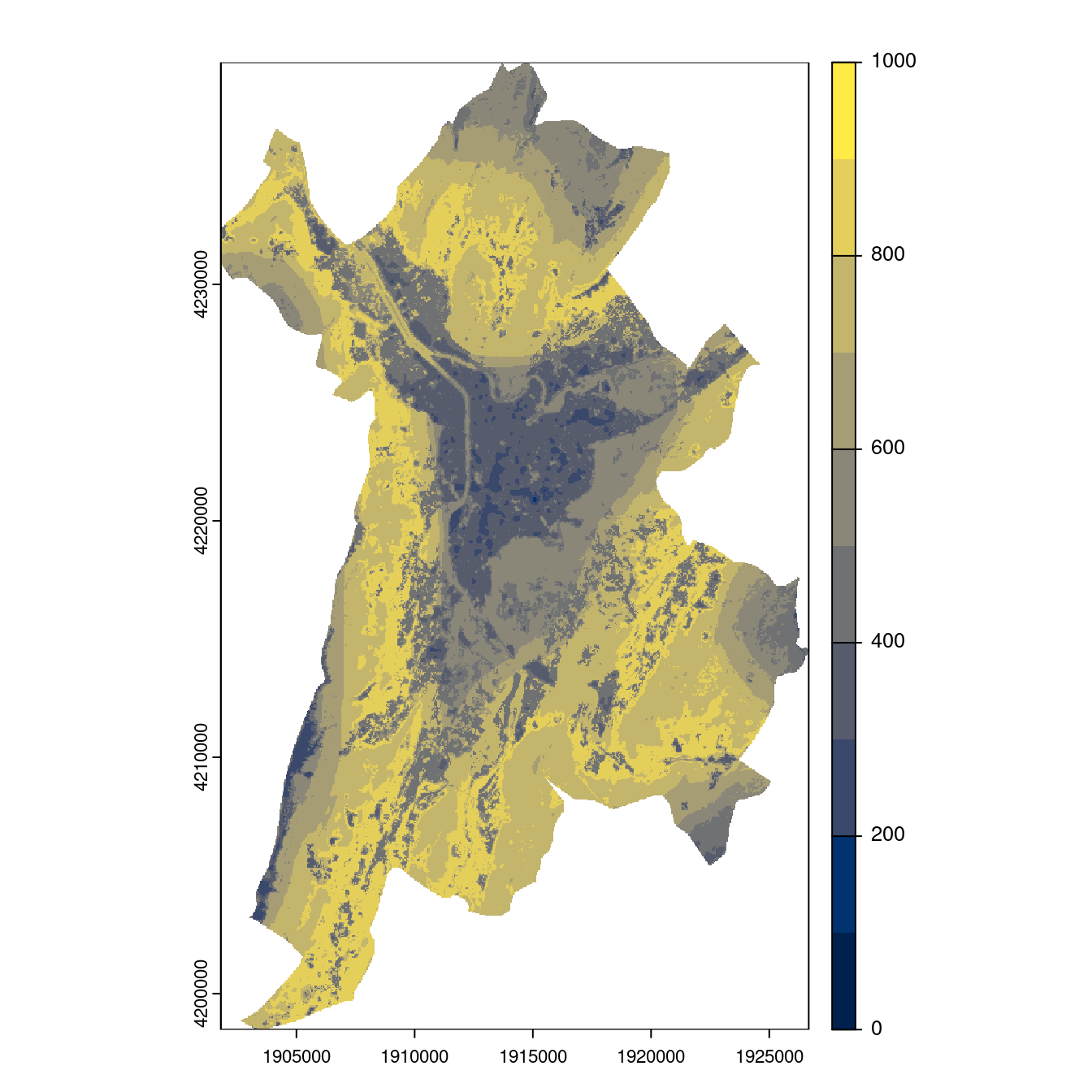
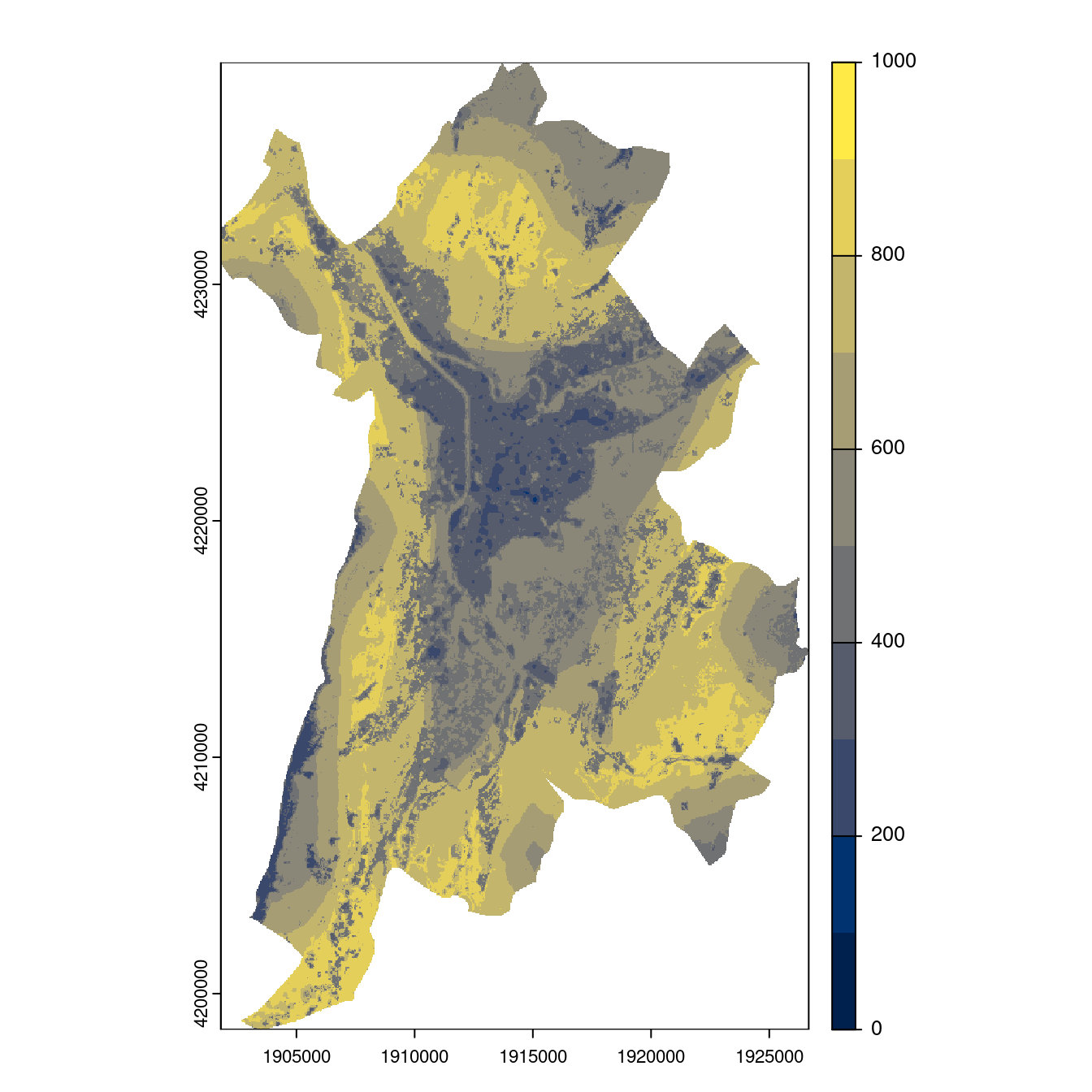
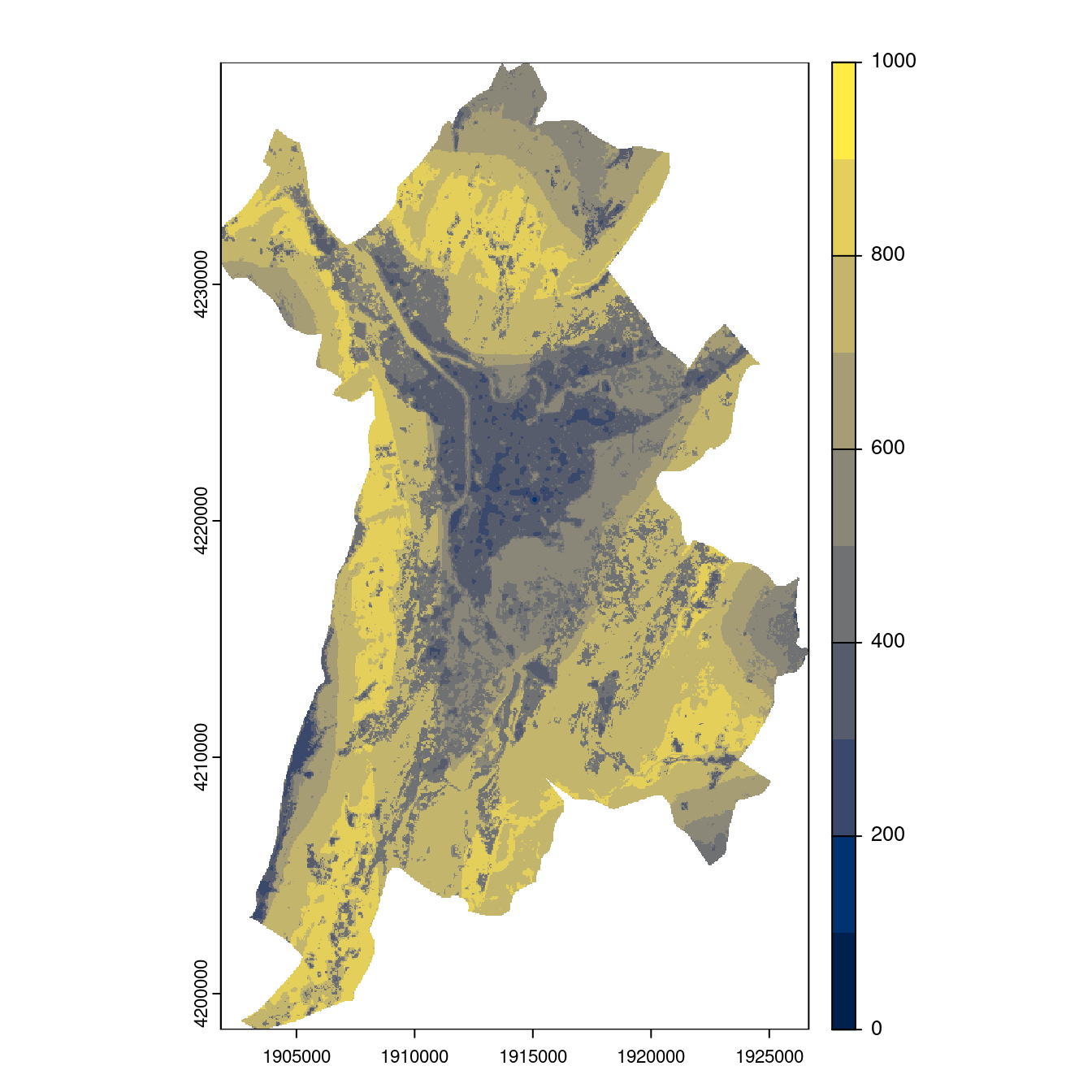
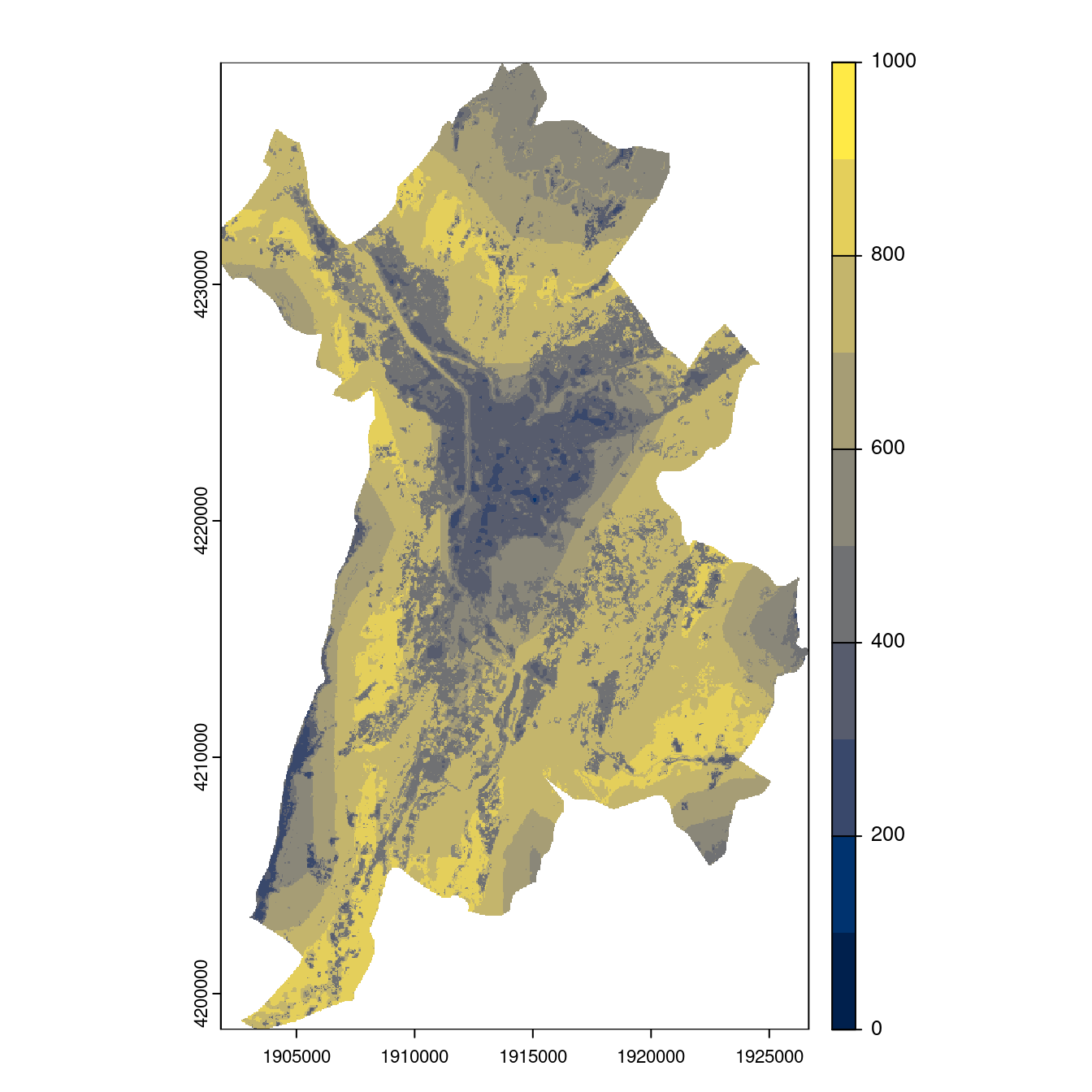
Figure 29.24: Projection des distributions potentielles contemporaine et futures jusqu’à l’horizon 2100 sous le scénario SSP5-8.5 (modèle d’ensemble, GCM IPSL).
- SSP2-4.5
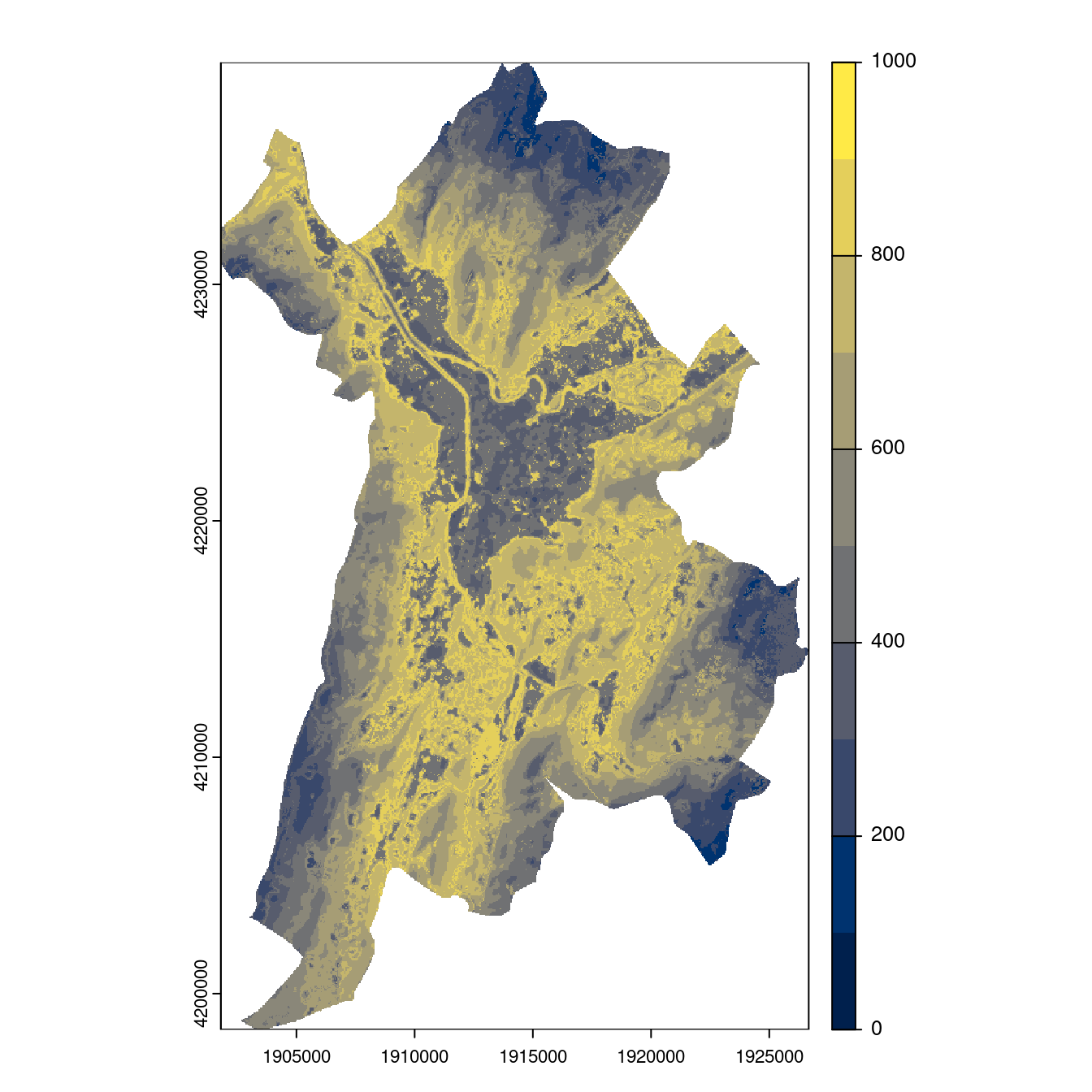
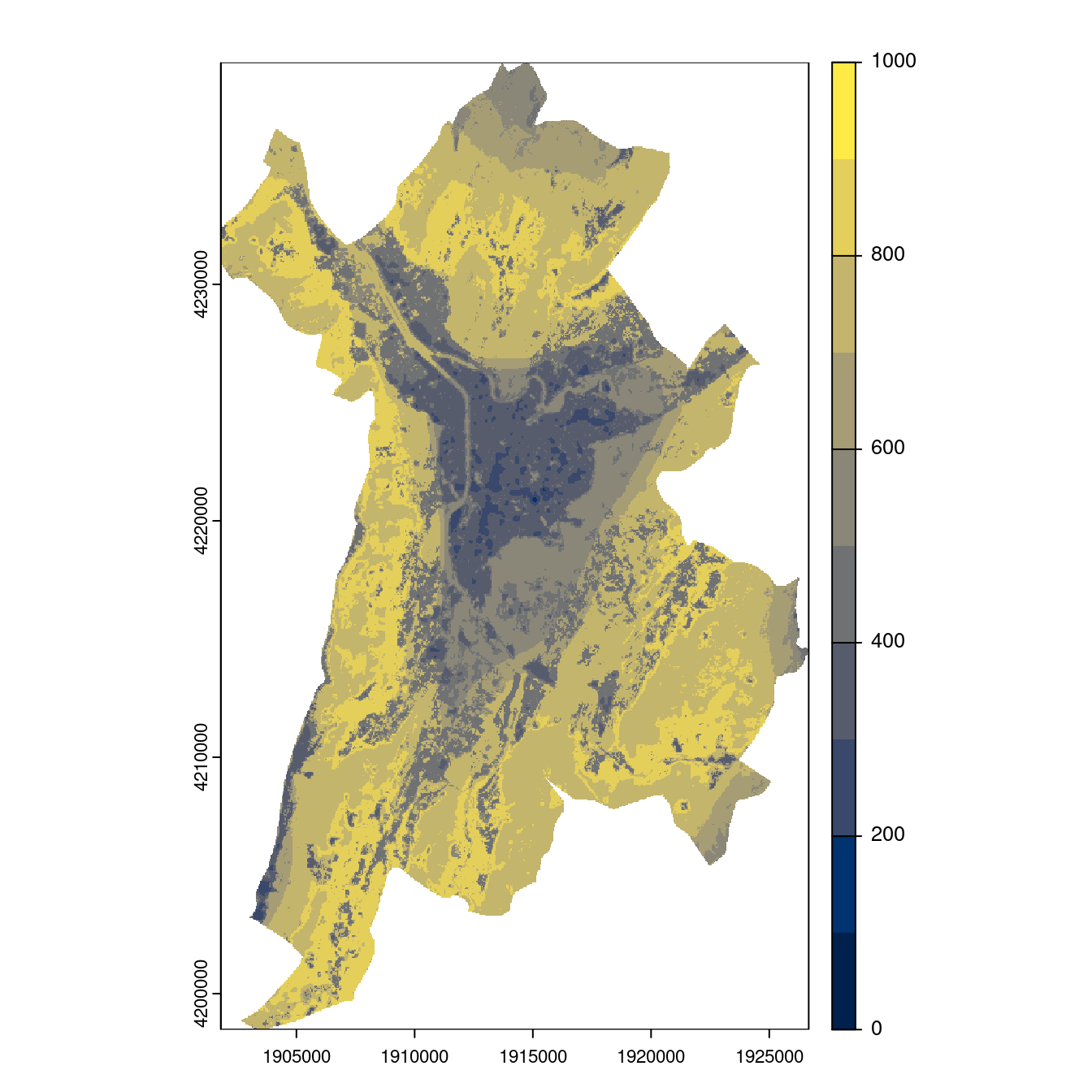
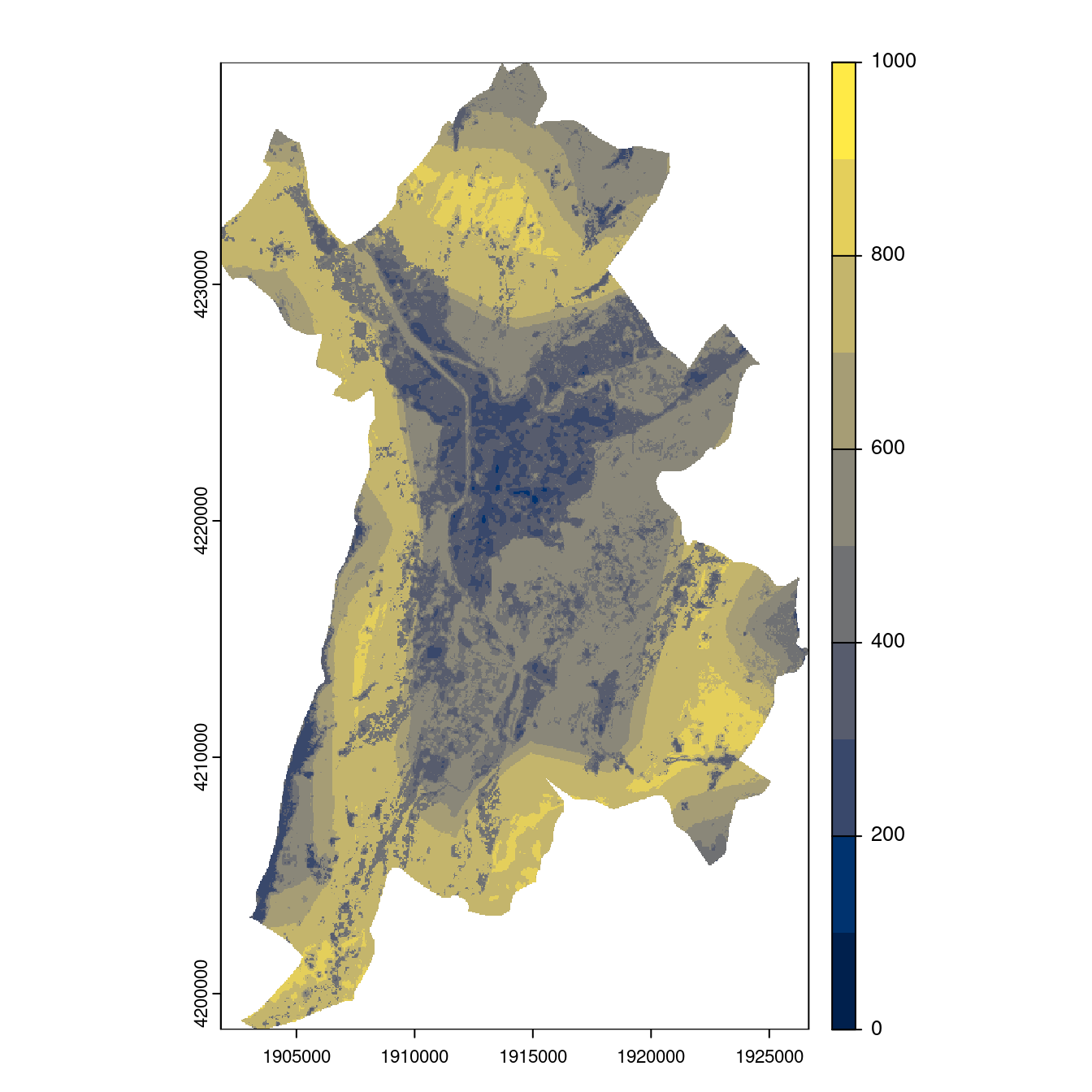
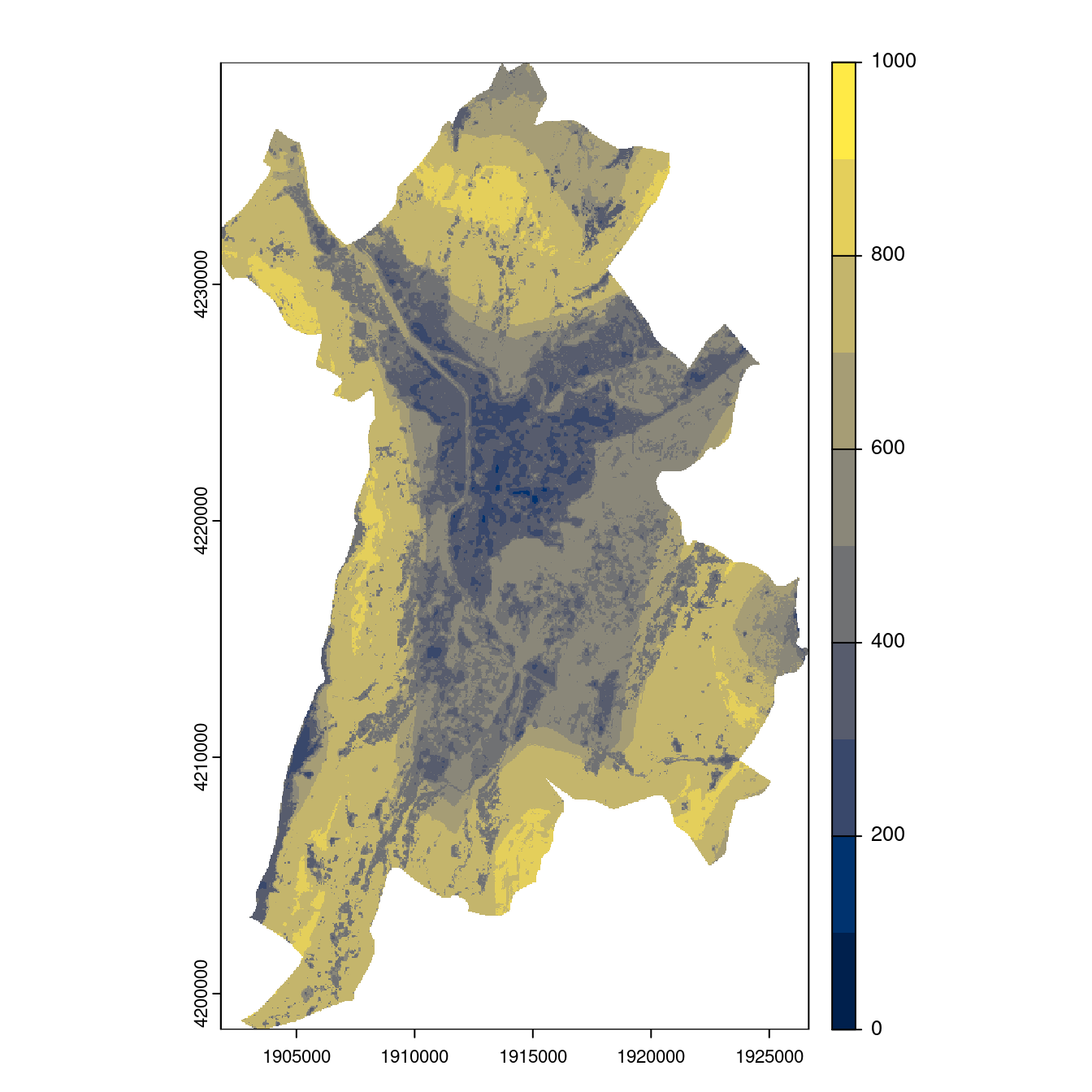
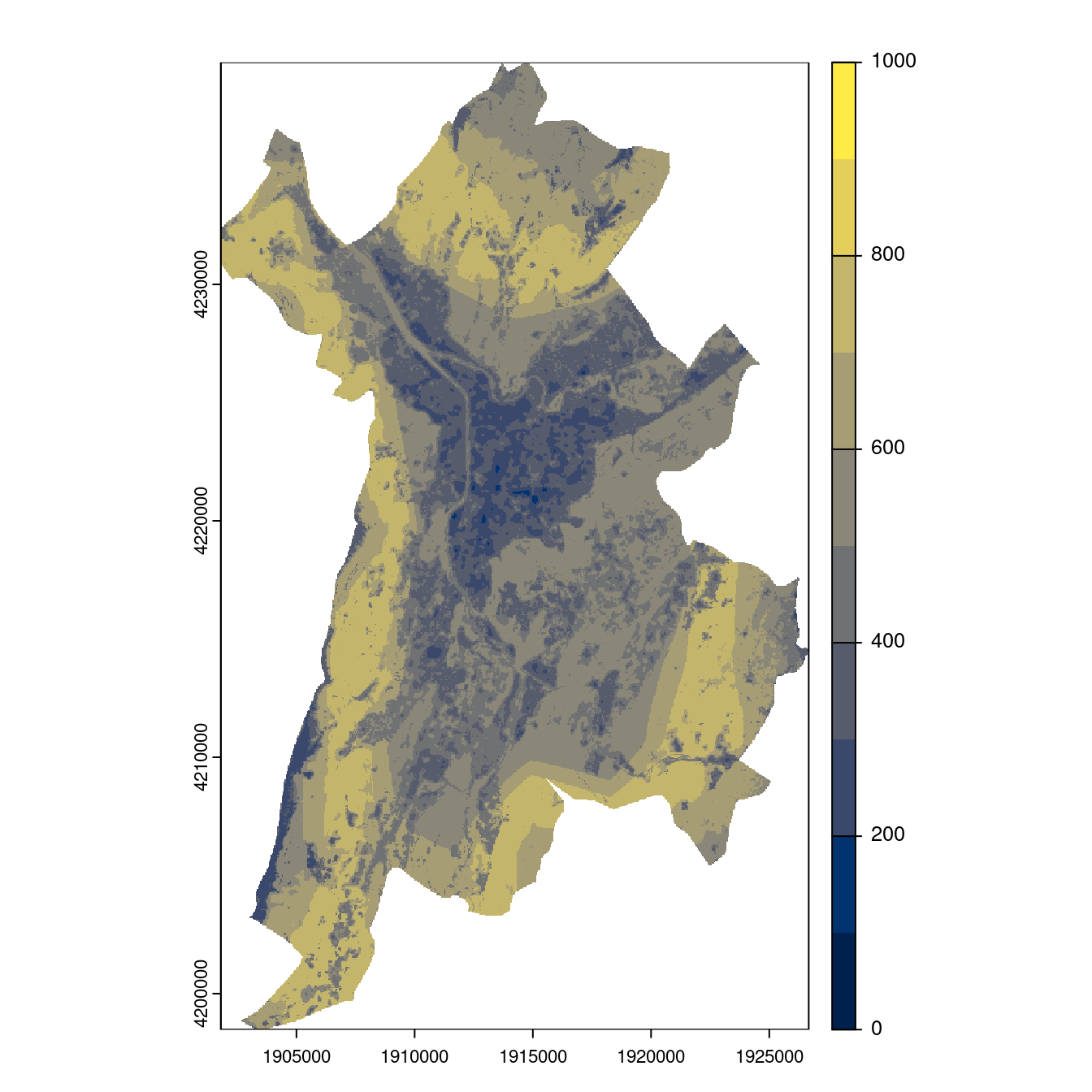
Figure 29.25: Projection des distributions potentielles contemporaine et futures jusqu’à l’horizon 2100 sous le scénario SSP5-8.5 (modèle d’ensemble, GCM IPSL).
- SSP3-7.0
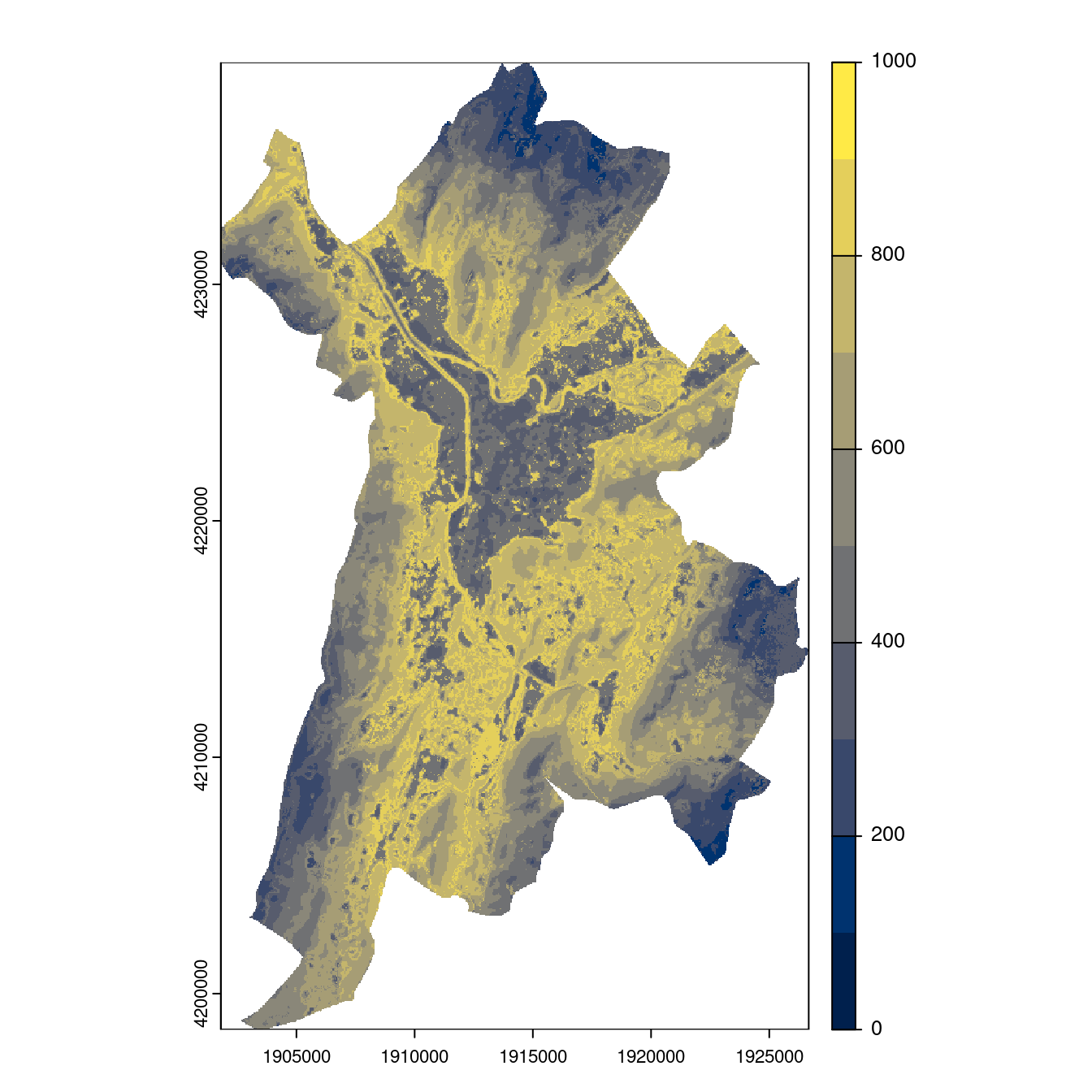
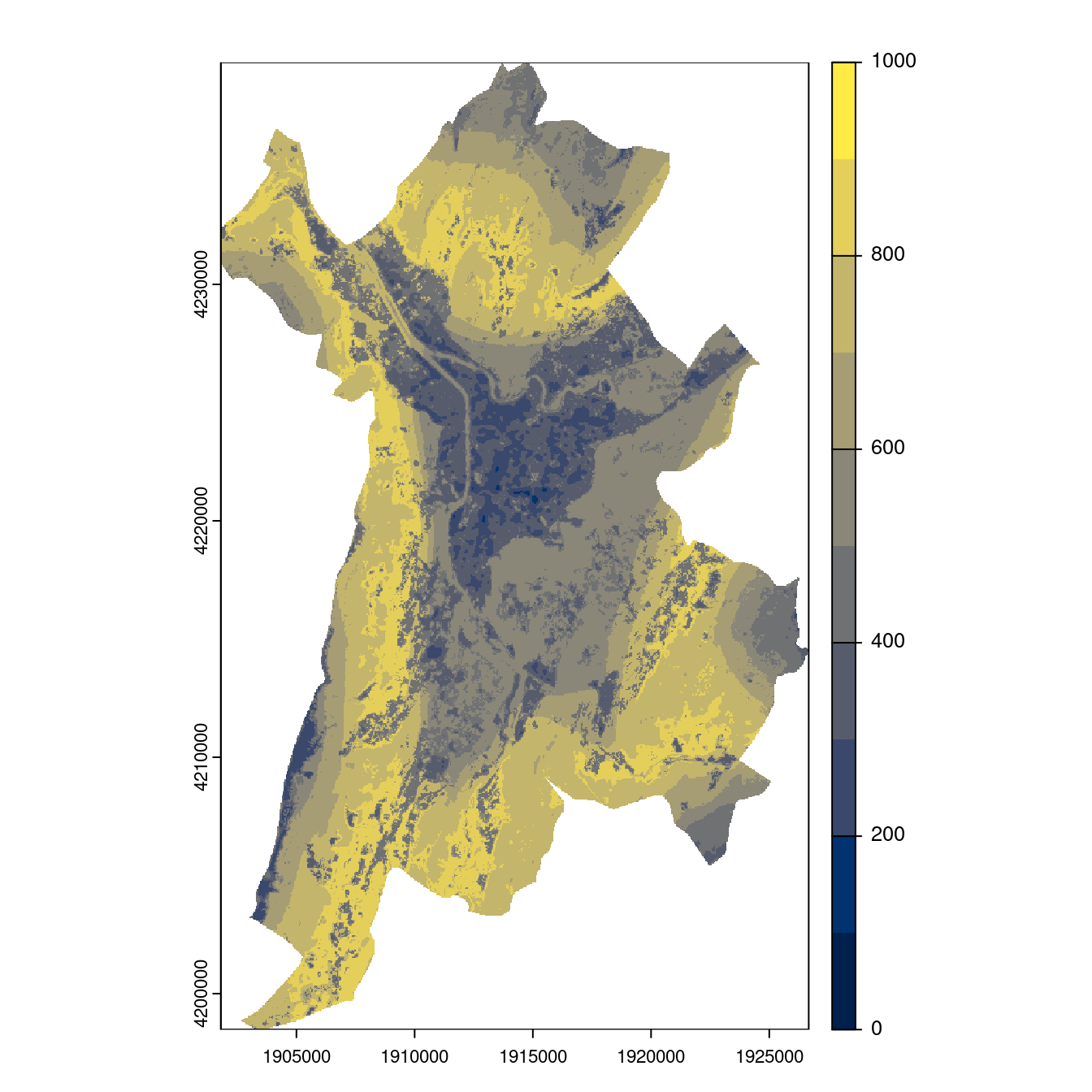
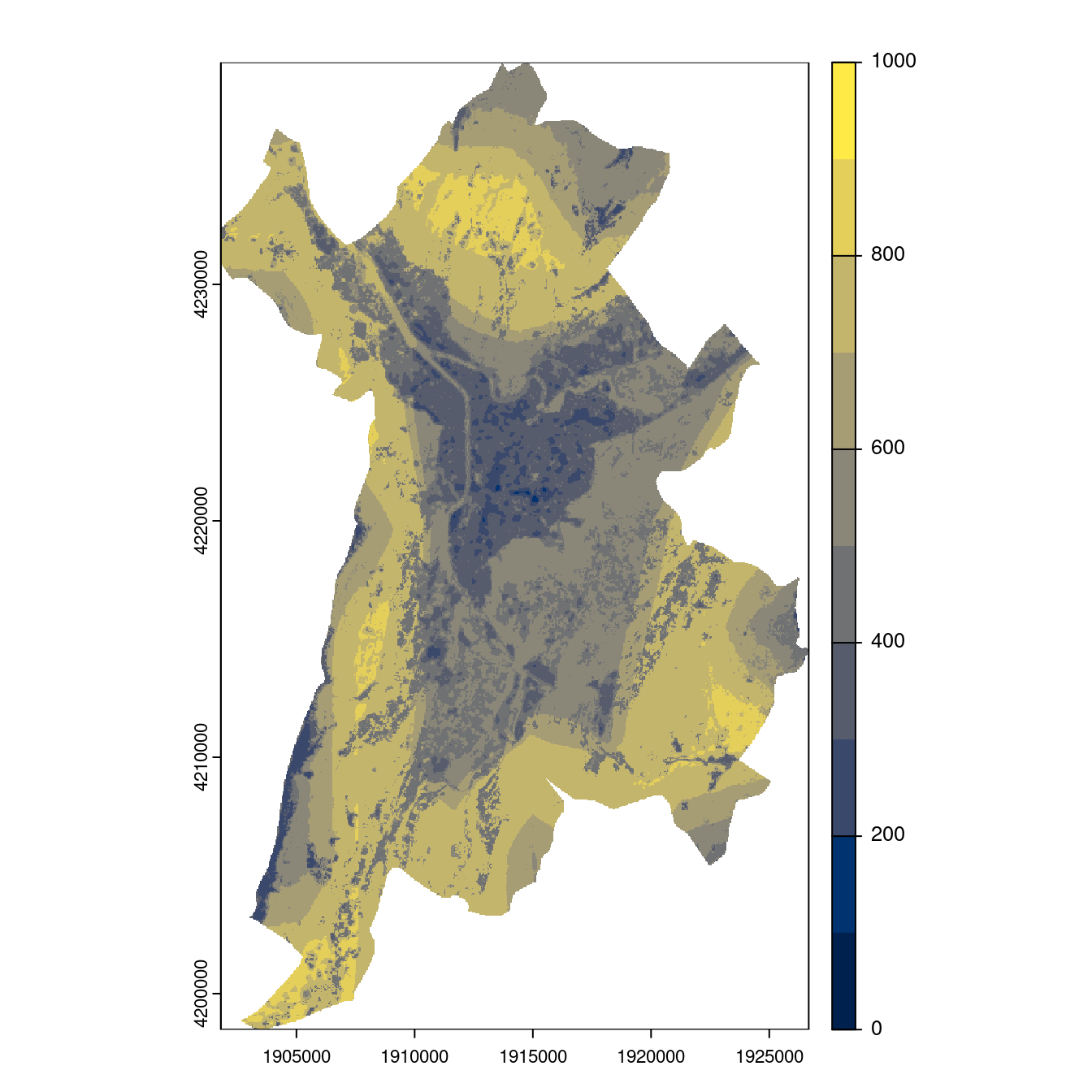
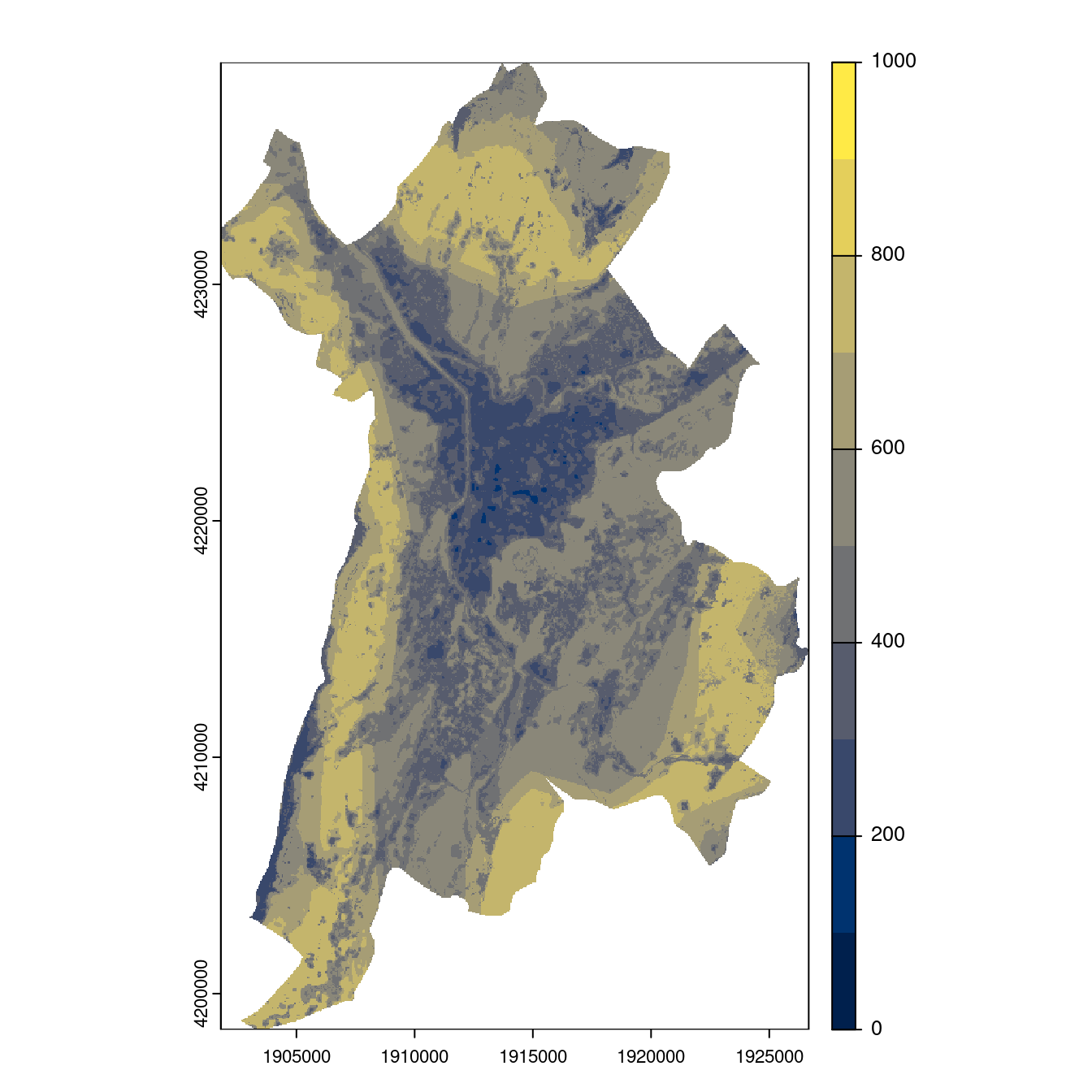
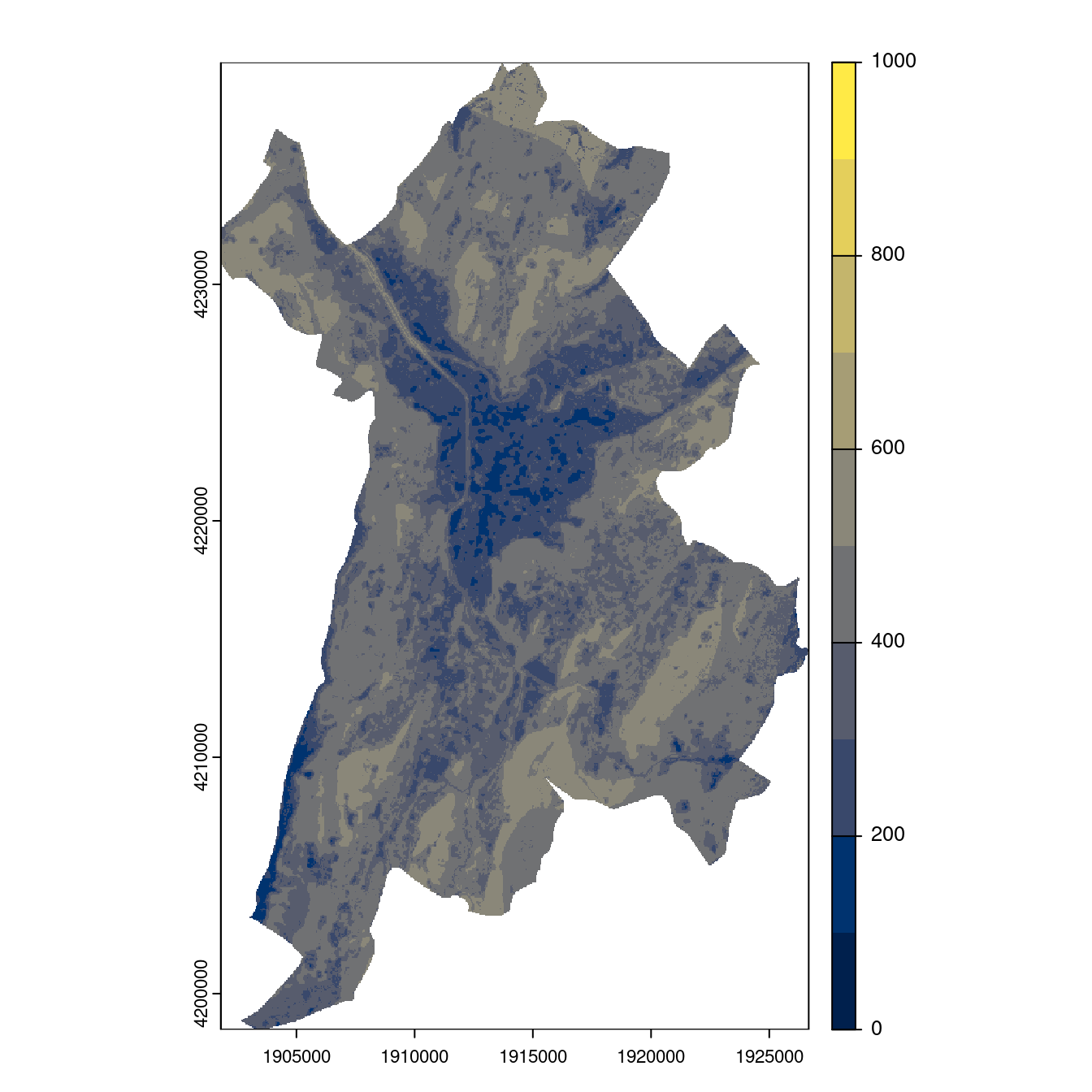
Figure 29.26: Projection des distributions potentielles contemporaine et futures jusqu’à l’horizon 2100 sous le scénario SSP5-8.5 (modèle d’ensemble, GCM IPSL).
- SSP5-8.5
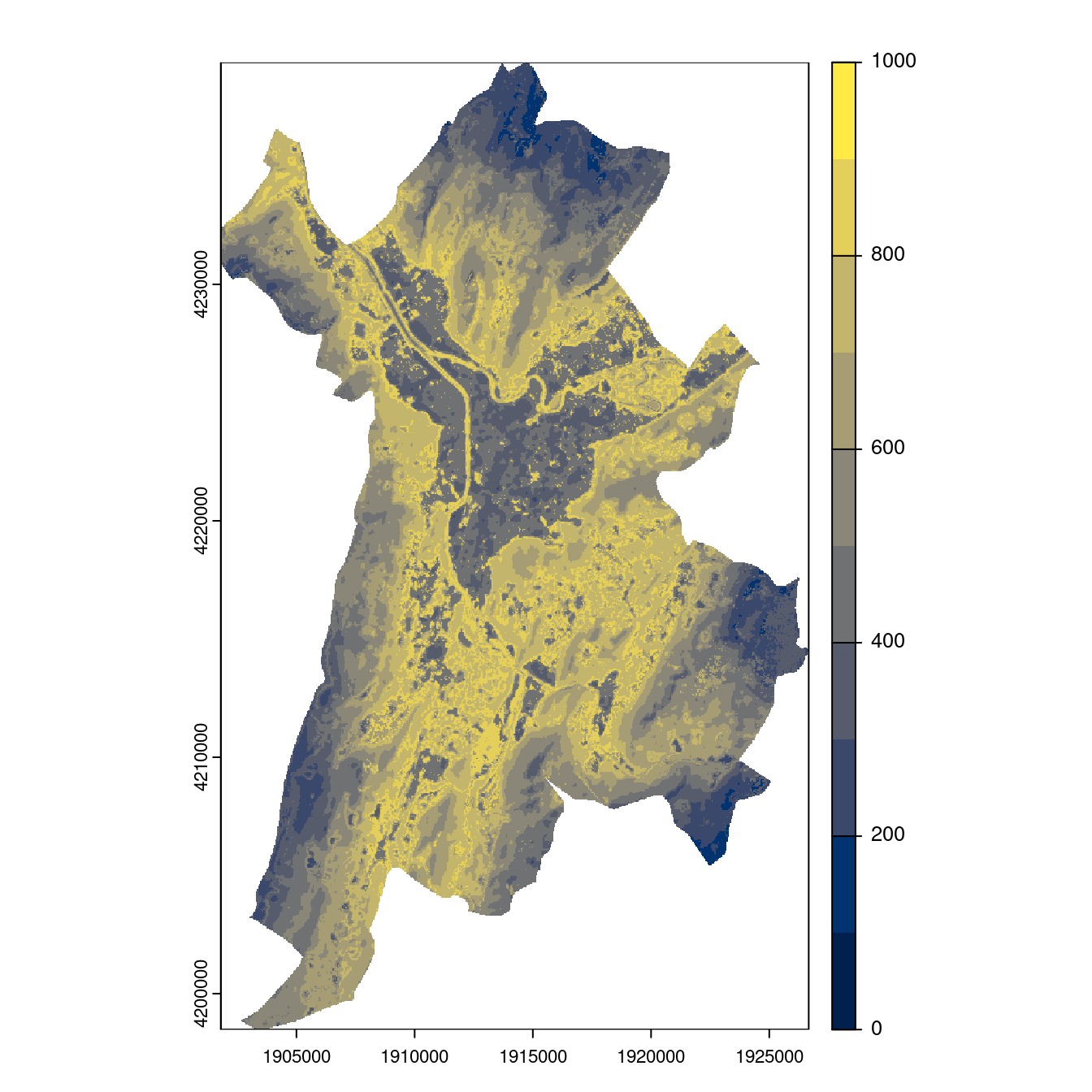
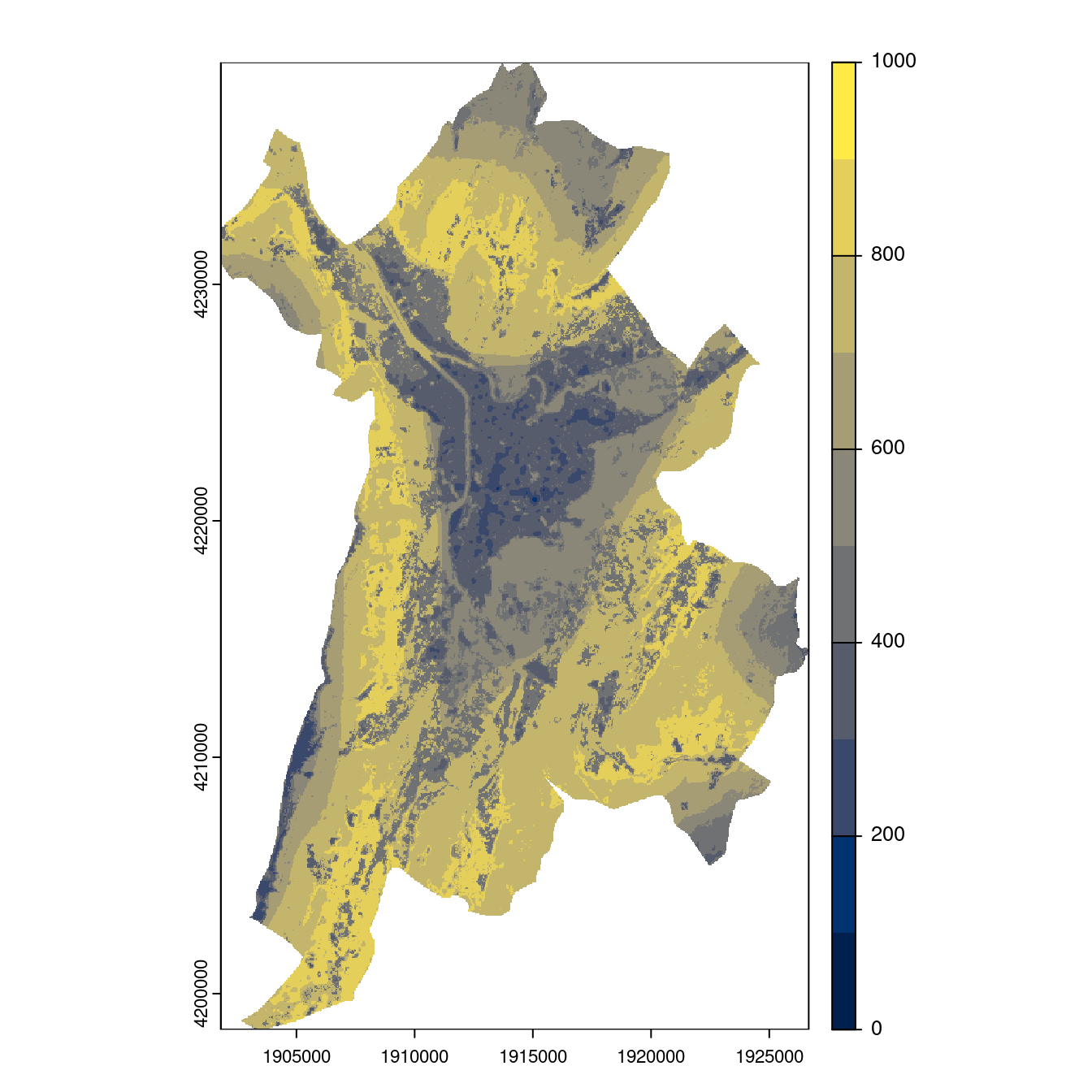
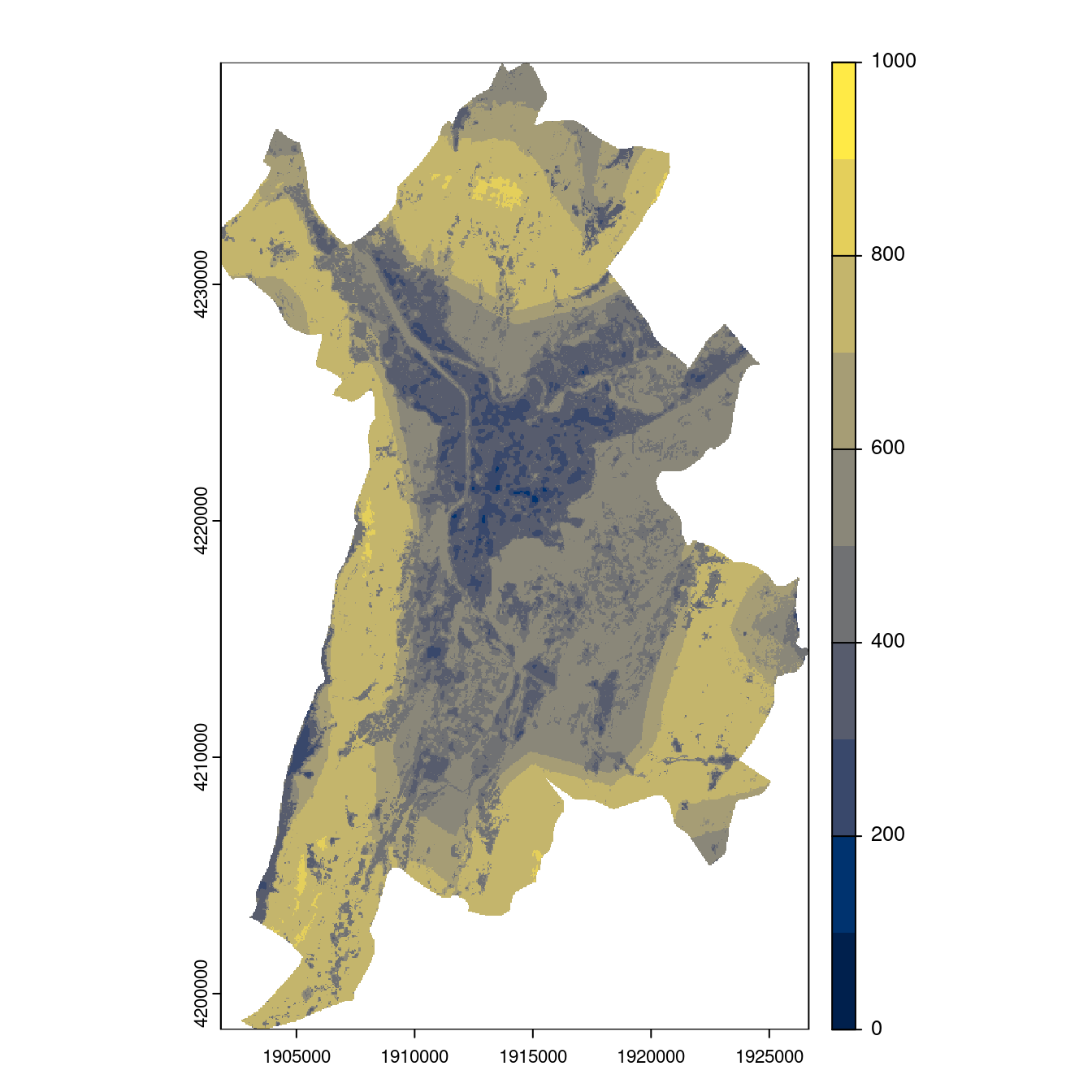
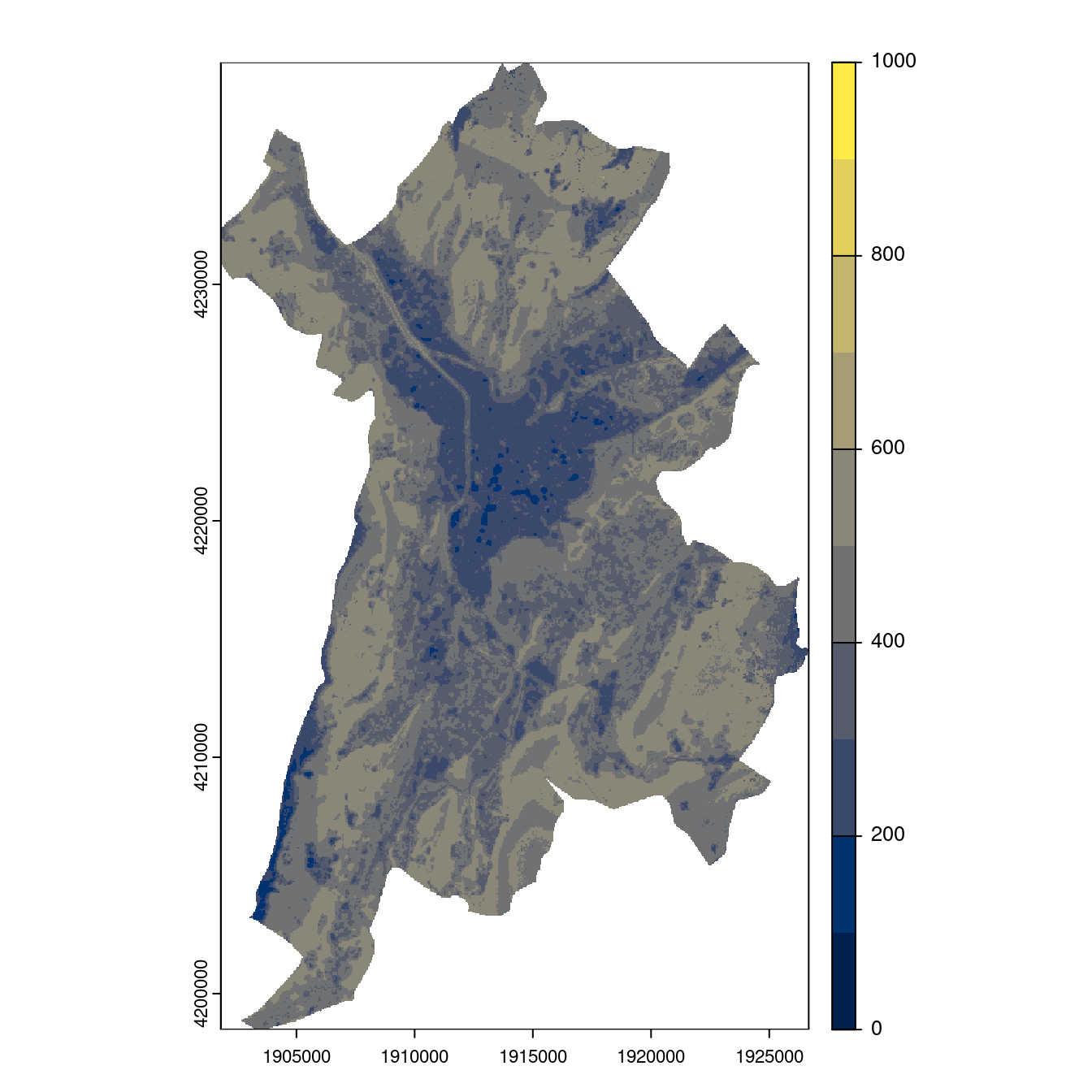
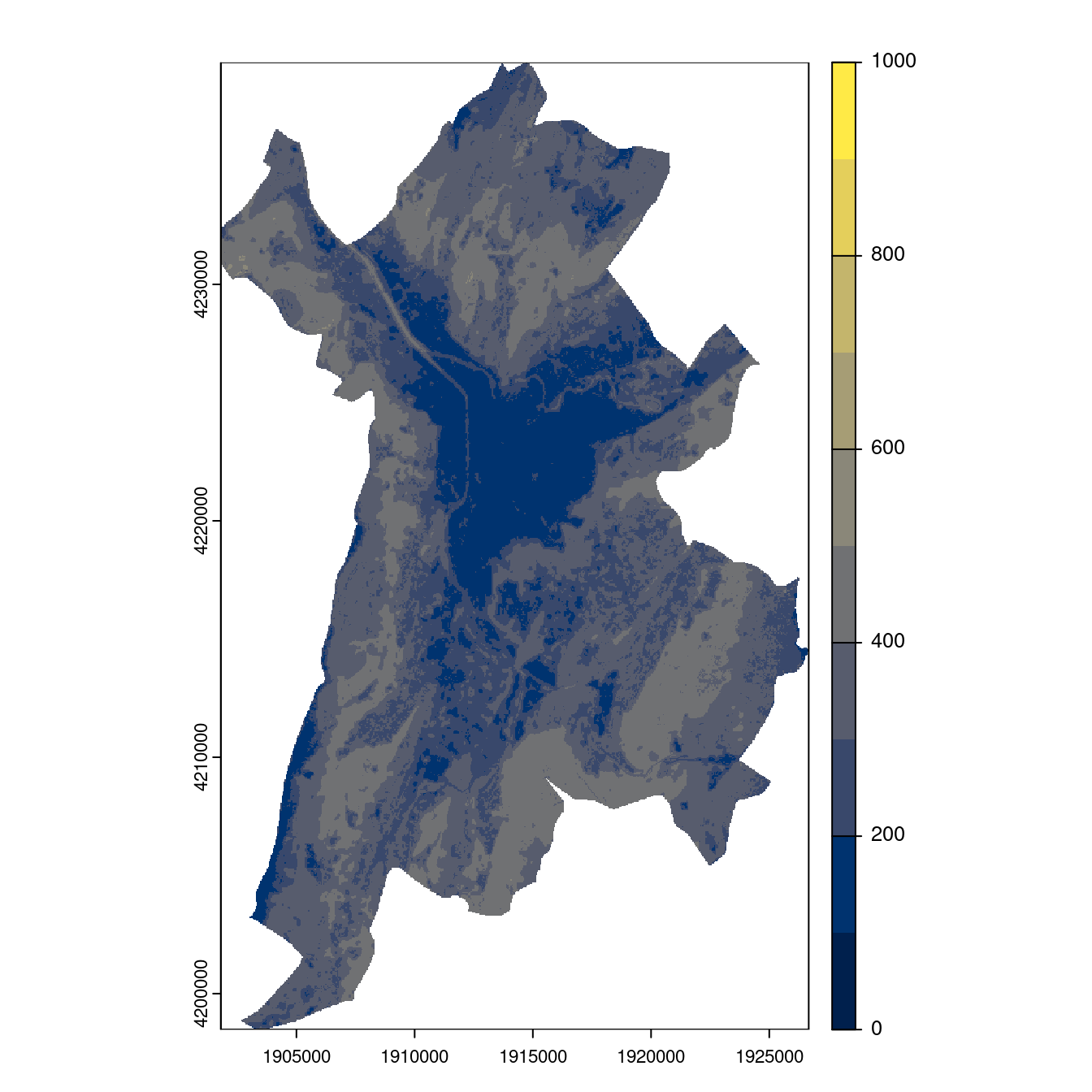
Figure 29.27: Projection des distributions potentielles contemporaine et futures jusqu’à l’horizon 2100 sous le scénario SSP5-8.5 (modèle d’ensemble, GCM IPSL).
29.4.2 Évolution temporelle
# A tibble: 17 × 8
min q1 median mean q3 max ssp year
<table[1d]> <table[1d]> <table[1d]> <table[1d]> <table[1d]> <tab> <fct> <dbl>
1 115 443.00 600 583.1888 734 849 0 2000
2 191 501.00 696 640.5754 784 848 126 2040
3 190 486.00 638 616.8014 770 845 126 2060
4 190 489.00 692 635.3581 780 843 126 2080
5 192 511.00 675 636.6735 771 844 126 2100
6 191 515.00 748 659.4429 790 848 245 2040
7 172 451.00 575 584.9202 756 839 245 2060
8 171 458.00 602 598.1059 752 831 245 2080
9 170 430.25 557 545.2404 680 787 245 2100
10 174 471.00 622 608.8077 769 842 370 2040
11 173 471.00 601 600.7822 752 837 370 2060
12 169 416.00 548 531.7756 674 797 370 2080
13 156 338.00 419 397.8707 465 589 370 2100
14 190 494.00 691 634.7274 779 845 585 2040
15 170 451.00 570 579.6449 730 816 585 2060
16 165 354.00 443 422.1057 503 604 585 2080
17 138 242.00 338 318.3238 393 539 585 2100
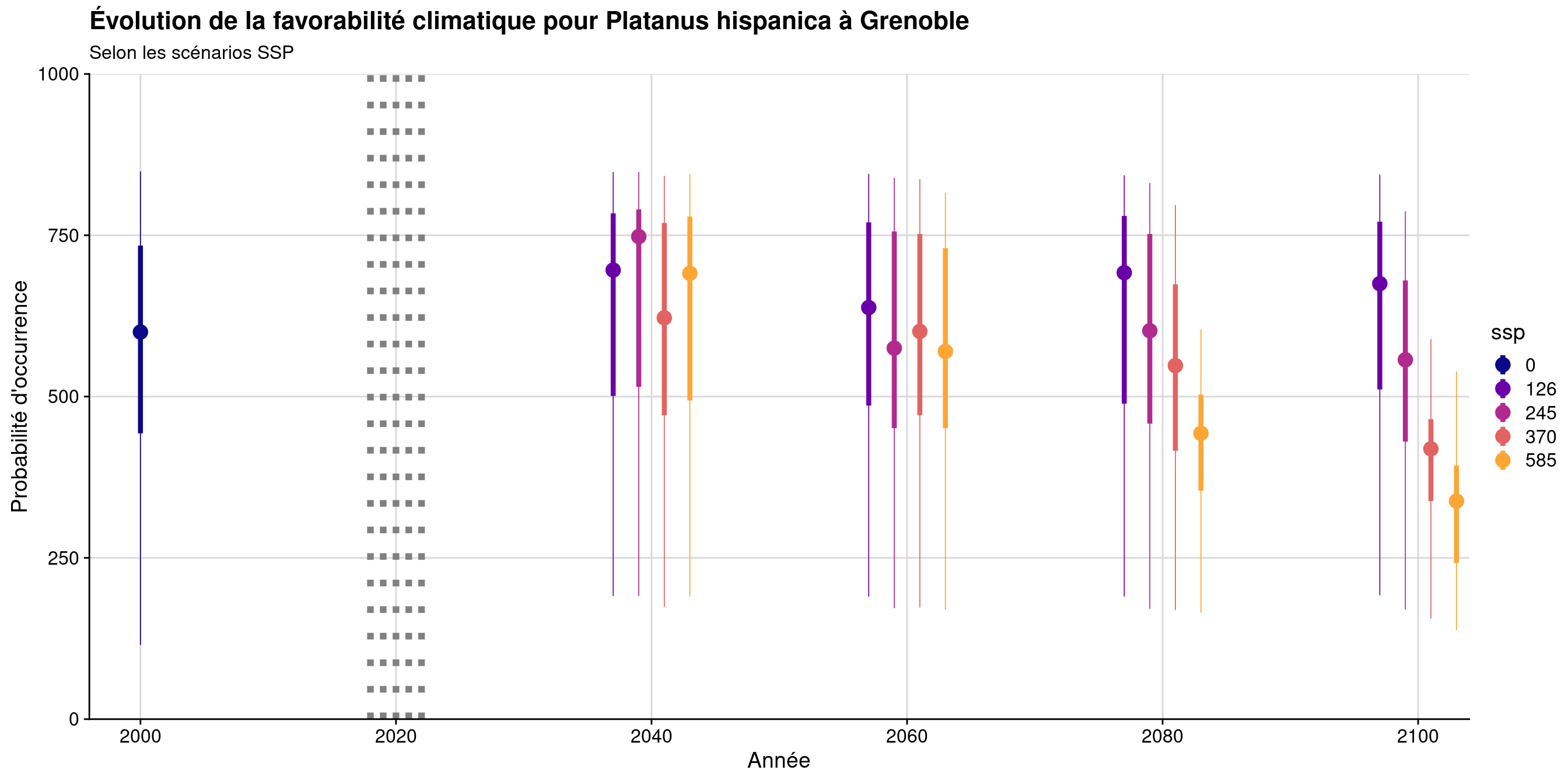
Figure 29.28: Évolution de la favorabilité climatique au cours du XXIème siècle. Le point représente la médiane sur l’étendue de la métropole (i.e. tous les pixels de la carte) ; le trait gras représente 50 % des données intermédiaires (espace inter-quartile) ; le trait fin représente le reste des données du minimum au maximum.